Revision Notes for CBSE Class 12 Maths Chapter 12 (Linear Programming) - Free PDF Download
CBSE Class 12 Maths Notes Chapter 13 Probability plays a crucial role in scoring good marks in maths. Probability is a very important topic from any examination perspective. It measures the uncertainty of happening something. Vedantu notes class 12 provide crisp and excellent key points from probability notes class 12. This article will be the complete probability class 12 notes. We’ll look after the whole probability one by one starting from basic definitions. At the end of the article, we’ll also discuss some of the frequently asked questions of probability to give a better understanding.
CBSE Class 12 Maths Revision Notes 2024-25 - Chapter Wise PDF Notes for Free
In the table below we have provided the PDF links of all the chapters of CBSE Class 12 Maths whereby the students are required to revise the chapters by downloading the PDF.
CBSE Class 12 Maths Chapter-wise Notes | |
Chapter 13: Probability Notes |
Probability Related Important Links
It is a curated compilation of relevant online resources that complement and expand upon the content covered in a specific chapter. Explore these links to access additional readings, explanatory videos, practice exercises, and other valuable materials that enhance your understanding of the chapter's subject matter.
Probability Related Study Materials |
Class 12 Study Materials Important Links
Find a curated selection of study resources for Class 12 subjects, helping students prepare effectively and excel in their academic pursuits.
Important Class 12 Related Links |
Probability Class 12 Notes Maths - Basic Subjective Questions
Section–A (1 Mark Questions)
1. If $P\left ( A\cap B \right )=70%\;P(B)=85%$ the find P(A/B).
Ans . As $P\left ( A/B\right )=\dfrac{P\left ( A\cap B \right )}{P(B)}$
$=\dfrac{70}{100}\times\dfrac{100}{85}=\dfrac{14}{17}$
2. Find the value of k from the probability distribution of the discrete variable X given below:
X | 2 | 3 | 4 | 5 |
$P\left ( X \right )$ | $\frac{5}{k}$ | $\frac{7}{k}$ | $\frac{9}{k}$ | $\frac{11}{k}$ |
Ans. As \sum P\left ( X \right )=1
$\Rightarrow \dfrac{5}{k}+\dfrac{7}{k}+\dfrac{9}{k}+\dfrac{11}{k}=1$
$\Rightarrow k=32$
3. If A and B are two independent events such that $P\left ( A \right )=\frac{1}{7}\;and\;P\left ( B \right )=\frac{1}{6}$ then find $P\left ( A{}'\cap B{}' \right )$ .
Ans. $P\left ( A{}'\cap B{}' \right )=P\left ( A{}' \right )P\left ( B{}' \right )$
$=\left ( 1-\dfrac{1}{7} \right )\left ( 1-\dfrac{1}{6} \right )$
$=\frac{6}{7}\times\dfrac{5}{6}=\frac{5}{7}$
4. A speaks truth in 70% cases and B speaks truth in 85% cases. The probability that they speak the same fact is ________.
Ans. As $P(same\;fact)=P\left ( AB\;or\;A\bar{}B\bar{}\right )$
$=\dfrac{70}{100}\times\dfrac{85}{100}\times\dfrac{30}{100}\times\dfrac{15}{100}$
$=\dfrac{5950+450}{10000}=64%$
5. The possibility of having 53 Thursdays in a non – leap year is ________.
Ans. In a non – leap year, there are 365 days, i.e. 52 weeks.
52 weeks = 364 day
1 year = 52 weeks and 1 day
This extra one day can be mon, tue, wed, thu, fri, sat, or sun.
Total number of outcomes = 7
Number of favourable outcomes = 1
P(having 53 Thursdays) $=\frac{1}{7}$
Section–B (2 Marks Questions)
6. Five cards are drawn successively with replacement from a well shuffled deck of 52 cards. What is the probability that only 3 cards are spades?
Ans Here, probability of getting a spade from a deck of 52 cards $=\frac{13}{52}=\frac{1}{4},p=\frac{1}{4},q=\frac{3}{4}$ Let, x is the number of spades, then x has the binomial distribution with n = 5, $p=\frac{1}{4},q=\frac{3}{4}$
P(only 3 cards are spades) $P=(x=3)=^{5}c_{3}\left ( \frac{3}{5} \right )^{5-3}\left ( \frac{1}{4} \right )^{3}=\frac{45}{512} $
7. In a box containing 100 bulbs, 10 are defective. What is the probability that out of a sample of 5 bulbs, none is defective?
Ans. Probability of defective bulbs $=P=\frac{10}{100}=\frac{1}{10}$
Probability of non defective bulb $=q=1-\frac{1}{10}=\frac{9}{10}$
Let x be the number of defective bulbs.
Therefore x is the binomial distribution with $n=5,p=\frac{1}{10},q=\frac{9}{10}$
Required probability $=P(x-0)=^{5}c_{0}\left ( \frac{9}{10} \right )^{5}\left ( \frac{1}{10}\right )^{0}=\left ( \frac{9}{10} \right )^{5}$
8. Let A and B be two given independent events such that: P(A) = p, P(B) = q & P (exactly one of A, B) $=\frac{2}{3}$, then find value of 3p + 3q – 6pq.
Ans. As $P(A)P(B\bar{})+P(A\bar{})P(B)=\frac{2}{3}$
$\Rightarrow p\cdot (1-q)+(1-p)q=\frac{2}{3}$
$\Rightarrow p-pq+q-pq=\frac{2}{3}$
$\Rightarrow 3p+3q-6pq=2$
9. Mother, father, and son line up at random for a family picture E: Son on one end, F: Father in middle. Find (E | F).
Ans. S-{mfs,msf,fms,fsm,smf,sfm}
E={mfs,fms,smf,sfm}
F=(mfs,sfm)
$E\cap F$={mfs,sfm}
$P(E/F)=\dfrac{P(E\cap F)}{P(F)}=\dfrac{\dfrac{2}{6}}{\dfrac{2}{6}}=1$
10. Prove that if E and F are independent events, then the events E and F’ are also independent.
Ans. Two events A and B are independent if
$P(A\cap B)=P(A).P(B)$
Now, $P(A\cap F{}')=P(E\;and\;not\;F)$
$=P(E)-P(E\cap F)$
$=P(E)-P(E).P(F)$
(Since E and F are independent events)
$=P(E)(1-P(F))$
$=P(E).P(F{}')$
11. Given $P(A)=0.4,P(B)=0.7$ and P(B/A)=0.6 Find $P(A\cup B)$
Ans. $P(B/A)=\frac{P\left ( A\cap B \right )}{P(A)}$
$=0.6\times0.4=P(A\cap B)$
$=P(A\cap B)=0.24$
Now, $P(A\cap B)=P(A)+P(B)-P(A\cap B)$
=0.4+0.7-0.24
=0.86
12. If each element of a second order determinant is either zero or one, what is the probability that the value of the determinant is positive? (Assume that the individual entries of the determinant are chosen independently, each value being assumed with probability $\dfrac{1}{2}$ ).
Ans. There are four entries in a determinant of 2\times2 order. Each entry may be filled up in two ways with 0 or 1.
Number of determinants that can be formed =24=16
The value of determinant is positive in following cases
$\left|\begin{array}{ll}1 & 0 \\ 0 & 1\end{array}\right|,\left|\begin{array}{ll}1 & 0 \\ 1 & 1\end{array}\right|,\left|\begin{array}{ll}1 & 1 \\ 0 & 1\end{array}\right|=3$
Therefore, the probability that the determinant is positive $=\frac{3}{16}$
13. A die is thrown 6 times. If “getting an odd number” is a “success”, what is the probability of (i) 5 successes? (ii) atmost 5 successes.
Ans. Let X: getting an odd number
$p=\frac{1}{2},q=\frac{1}{2},n=6$
(i) $P(X=5)=_{}^{6}\textrm{}C_{5}\left ( \frac{1}{2} \right )^{6}=\frac{3}{32}$
(ii) $P(X\leq 5)=1-P(X=6)=1-\frac{1}{64}=\frac{63}{64}$
PDF Summary - Class 12 Maths Probability Notes (Chapter 13)
Basic Definitions:
Random Experiment:
An experiment in which all possible outcomes are known ahead of time, but the outcome of any particular performance cannot be predicted until the experiment is completed.
For example - Tossing of a coin, throwing a dice, etc.
Sample-space:
A collection of all conceivable outcomes from a single random experiment.
Sample space is denoted as ‘\[S\]’.
For example: If we're interested in the number that appears on the top face of a die, sample space would be
\[S=\left\{ 1,\text{ }2,\text{ }3,\text{ }4,\text{ }5,\text{ }6 \right\}\]
Experiment or Trial:
It is a succession of actions with unpredictably unclear outcomes.
Example - Tossing of a coin, selecting a card from deck of cards, throwing a dice.
Event:
Subset of sample – space.
In any sample space, we might be more interested in the occurrence of various occurrences than the existence of a given element.
Simple Event:
If an event is a set with only one sample-space element, it is called a single-element event.
Compound Event:
A compound event can be represented as a collection of sample points.
Example:
Event of drawing a spade from a deck of cards is the subset \[A=\left\{ \text{spade} \right\}\] of the sample space \[S=\left\{ \text{heart, spade, club, diamond} \right\}.\]
Therefore \[A\] is a simple event. None the event B of drawing a balck card is a compound event since
\[B=\left\{ spade\text{ }U\text{ }c\operatorname{lub} \right\}=\left\{ spade,c\operatorname{lub} \right\}\].
Probability:
If each one of \[N\] alternative equally likely outcomes can result from a random experiment, and if exactly \[n\] of these events favours \[A\].
\[A,P\left( A \right)=\dfrac{n}{N}\]
That is \[\dfrac{\text{Favourable Cases}}{\text{Total No}\text{. of Caes}}\]
Remarks:
Just because a given event has a probability of one does not indicate it will happen with certainty.
It is just predicting that the event is most likely to occur in contrast to other events.
Predictions are based on historical data and, of course, the method of analysing the data at hand.
Similarly, just because the probability of an occurrence is zero does not entail that it will never happen!
Mutually Exclusive Event:
Two mutually exclusive occurrences cannot occur at the same time.
Independent Events:
When the occurrence or non-occurrence of one event has no bearing on the occurrence or non-occurrence of another, the events are said to be independent.
Exhaustive Event:
If a random experiment always results in the occurrence of at least one of a set of events, it is said to be exhaustive.
Conditional Probability:
The conditional probability is the probability that an event \[B\] will occur given the knowledge that an event \[A\]has previously occurred.
This probability is written \[P\text{ }\left( B|A \right),\] notation for the probability of \[B\] given \[A\].
When events \[A\] and \[B\] are the independent, the conditional probability of event \[B\] for event \[A\] is the probability of event \[B\], that is \[P\], in the case when events \[A\] and \[B\] are independent (that is, event \[A\] has no effect on the probability of event \[B\]) \[P\text{ }\left( B \right)\].
Since the events \[A\] and \[B\] are not independent, the probability that they will intersect (that both events will occur) is given by
\[P\text{ }\left( A\text{ and }B \right)\text{ }=\text{ }P\text{ }\left( A \right)\text{ }P\text{ }\left( B|A \right)\].
If a random experiment has two sample space of \[E\] and \[S\] that these two events linked with the same sample space, the conditional probability of the event \[E\] given that \[S\] has occurred,
That is,
\[P(E|S)\] is given by
$P(E|S)=\dfrac{P(E\cap S)}{P(S)}$
Provided $P(S)\ne 0$
Example:
If $\text{P(A) = }\dfrac{5}{\text{12}}\text{, P(D) = }\dfrac{7}{\text{12}}$ and $P(A\cap D)=\dfrac{3}{12}$ , evaluate \[P(A|D)\].
\[P(A|D)=\dfrac{P(A\cap D)}{P(D)}\]
\[P(A|D)=\dfrac{\dfrac{3}{12}}{\dfrac{7}{12}}\]
\[P(A|D)=\dfrac{3}{7}\]
Properties of Conditional Probability:
Let’s consider \[E\] and \[F\] be the events of a sample space \[S\] of an experiment, then
Property \[1\] :
\[P\left( S|F \right)=P\left( F|F \right)=1\]
We know that,
\[P(E|F)=\dfrac{P(S\cap F)}{P(F)}=\dfrac{P(F)}{P(F)}=1\]
Also,
\[P(F|F)=\dfrac{P(F\cap F)}{P(F)}=\dfrac{P(F)}{P(F)}=1\]
Thus,
$P(S|F)=P(F|F)=1$
Property \[2\]:
Let’s consider that \[M\] and \[N\]be the any two events of a sample space \[S\] and \[F\] be an event of \[S\] such that\[P\left( F \right)\ne 0\] , then
$P((M\cup N)|F=P(M|F)+P(N|F)-P((M\cap N)|F$
If \[M\]and \[N\] are discontinuous events,
$P((M\cup N)|F)=P(M|F)+P(N|F)$
We have
$P((M\cup N)|F)=\dfrac{P[(M\cap N)|F]}{P(F)}$
$=\dfrac{P[(M\cap F)\cup (N\cap F)]}{P(F)}$
(Based on the distributive law of set union over intersection)
$=\dfrac{P(M\cap F)+P(N\cap F)-P(M\cap N\cap F)}{P(F)}$
\[=\dfrac{P(M\cap F)}{P(F)}+\dfrac{P(N\cap F)}{P(F)}-\dfrac{P[(M\cap N)\cap F]}{P(F)}\]
$=P(M|F)+P(N|F)-P((M\cap N)|F)$
When \[N\] and \[N\] are disjoint events, then
$P((M\cap N)|F)=0$
$\Rightarrow P((M\cup N)|F)=P(M|F)+P(N|F)$
Property \[3\] :
$P(E'|F)=1-P(E|F)$
From Property 1,
$P(S|F)=1$
$\Rightarrow P(E\cup E'|F)=1$ Since, $S=E\cup E'$
$\Rightarrow P(E|F)+P(E'|F)=1$ Since, $E$ and $E'$ are disjoint events
Thus,
$P(E'|F)=1-P(E|F)$
Multiplication Theorem on Probability:
Let \[E\] and \[F\] be two events linked with a sample space \[S\].
\[P\left( E|F \right)\] denotes the conditional probability of event \[E\] given that \[F\] has occurred .
$P(E|F)=\dfrac{P(E\cap F)}{P(F)},P(F)\ne 0$
From this result, we can write
$P(E\cap F)=P(F).P(E|F)........(1)$
Also, we know that
$P(F|E)=\dfrac{P(F\cap E)}{P(E)},P(E)\ne 0$
or $P(F|E)=\dfrac{P(F\cap E)}{P(E)}$ (since $E\cap F=F\cap E)$
Thus, $P(E\cap F)=P(E).P(F|E).........(2)$
Combining (1) and (2), we find that
$P(E\cap F)=P(E).P(F|E)$
$=P(F).P(E|F)$
Since, $P(E)\ne 0$ and $P(F)\ne 0$.
The above result is called as the Multiplication rule of probability.
Example:
There are \[25\] black and \[30\] white balls in an urn. Two balls are drawn one after the other from the urn without being replaced. Is it likely that both drawn balls will be black?
Ans.:
Consider, \[E\] and \[F\] are representing the events in which the first and second balls drawn are both black.
\[P\left( E \right)=P\] (Black ball in first draw) $=\dfrac{25}{55}$
Given that the first ball chosen is black, the event has taken place, and the urn now contains both \[24\] black and \[30\] white balls.
As a result, the conditional probability of \[F\] given that \[E\] has occurred is the given that the first ball pulled was black, the likelihood of the second ball being black is high.
That is
\[P\left( F|E \right)\text{ }=\text{ }\dfrac{24}{54}\]
By multiplication rule of probability, we have
\[P\text{ }\left( E\text{ }\cap \text{ }F \right)\text{ }=\text{ }P\text{ }\left( E
\right)\text{ }P\text{ }\left( F|E \right)\]
\[=\dfrac{25}{55}\text{ }\times \text{ }\dfrac{24}{55}=\dfrac{600}{3025}\]
\[=\dfrac{24}{121}\]
Note:
Probability multiplication rule for more than two events if \[E\] is true, \[F\]and \[G\] are three events of sample space, we have
\[P\left( E\cap F\cap G \right)=P\left( E \right)P\left( F|E \right)P\left( G|\left( E\text{ }\cap \text{ }F \right) \right)=P\left( E \right)P\left( F|E \right)P\left( G|EF \right)\]
Similarly, the probability multiplication rule can be extended to four or more events.
Independent Events:
If \[E\] and \[F\] are two events whose likelihood of occurrence is unaffected by the probability of occurrence of the other. Independent events are what they're termed when they happen on their own.
If \[E\] and \[F\] are two events that occur during the same random experiment, they are considered to be independent.
\[P\left( E\cap F \right)=P\left( E \right).\text{ }P\left( F \right)\]
Remarks:
Two events \[E\] and \[F\]are dependent if they are not independent, that is
\[P\left( E\cap F \right)\ne P\left( E \right).\text{ }P\left( F \right)\]
It's easy to get mixed up between independent and mutually exclusive events.
The term "independent" is defined in terms of "event probability," whereas "mutually exclusive" is defined in terms of "event probability" (subset of sample space).
Mutually exclusive events will never have a shared result, whereas independent events may.
Two mutually exclusive events with nonzero possibilities of occurrence cannot be mutually exclusive, and two mutually exclusive occurrences with nonzero probabilities of occurrence cannot be mutually exclusive.
Two experiments are independent for every pair of events \[E\] and \[F\], where first experiment is linked with the second experiment, then the probability of the simultaneous occurrence of the events \[E\] and \[F\]only when the two experiments are performed which is the product of \[P\left( E \right)\] and \[P\left( F \right)\] calculated separately on the basis of two experiments, that is
\[P\left( E\cap F \right)=P\left( E \right).\text{ }P\left( F \right)\]
Three events \[A,\text{ }B\] and \[C\] are said to be mutually independent, if
\[P\text{ }\left( A\text{ }\cap \text{ }B \right)\text{ }=\text{ }P\text{ }\left( A \right)\text{ }P\text{ }\left( B \right)\]
\[P\text{ }\left( A\text{ }\cap \text{ }C \right)\text{ }=\text{ }P\text{ }\left( A \right)\text{ }P\text{ }\left( C \right)\]
\[P\text{ }\left( B\text{ }\cap \text{ }C \right)\text{ }=\text{ }P\text{ }\left( B \right)\text{ }P\left( C \right)\] and
\[P\text{ }\left( A\text{ }\cap \text{ }B\text{ }\cap \text{ }C \right)\text{ }=\text{ }P\text{ }\left( A \right)\text{ }P\text{ }\left( B \right)\text{ }P\left( C \right)\]
We argue that three occurrences are not independent if at least one of the aforementioned conditions is not met.
Example
A die is thrown. Let \[E\] be the event ‘the number appearing is a multiple of \[2\] and \[F\] be the event ‘the number appearing is odd’ then find whether \[E\] and \[F\] are independent?
Solution:
The sample space is \[S=\left\{ 1,\text{ }2,\text{ }3,\text{ }4,\text{ }5,\text{ }6 \right\}\]
Now \[E=\left\{ 2,4,6 \right\},\text{ }F=\left\{ 1,3,5 \right\}\] and \[E\cap F=\left\{ 4 \right\}\]
Then
\[P\left( E \right)=\dfrac{3}{6}=\dfrac{1}{2}\]
\[P\left( F \right)=\dfrac{3}{6}=\dfrac{1}{2}\]
\[P\left( E\cap F \right)=\dfrac{1}{4}\]
Clearly,
\[P\left( E\cap F \right)=P\text{ }\left( E \right).\text{ }P\text{ }\left( F \right).\]
Hence, \[E\] and \[F\] are independent events.
Bayes' Theorem: Description:
Also called as inverse probability theorem
Consider that there are two sacs \[I\] and \[II\] .
Sac \[I\] contains \[2\] white and \[3\] red kites
Sac \[II\] contains \[4\] white and \[5\] red kites.
One kite is drawn at random from one of the sacs.
Probability of selecting any of the sac (that is $\dfrac{1}{2}$) or probability of drawing a kite of a particular colour that is white from a particular sac \[I\].
If we are given the sac from which the kite is drawn, the probability that the kite drawn will be of a specific colour.
If the colour of the kite pulled is known, we must find the reverse likelihood of sac \[II\] being selected when an event occurs after it is known to find the probability that the sac drawn is from a particular sac \[II\].
John Bayes, a famous mathematician, used conditional probability to address the challenge of obtaining reverse probability.
As a result, the ‘Bayes theorem' was named after him, and it was published posthumously in \[1763\].
Definitions:
Partition of a sample space:
The partition of the sample space \[S\] stated to be a set of events \[{{E}_{1}},\text{ }{{E}_{2}},\text{ }...,\text{ }{{E}_{n}}\]
If
\[Ei\text{ }\cap \text{ }Ej\text{ }=\text{ }\varphi \] , \[i\text{ }\ne \text{ }j,\text{ }i,\text{ }j\text{ }=\text{ }1,\text{ }2,\text{ }3,\text{ }...,\text{ }n\]
\[{{E}_{1}}\cup {{E }_{2}}\cup ...\cup {{E}_{n}}=\text{ }S\] and
\[P\text{ }\left( Ei \right)\text{ }>\text{ }0\] for all \[i\text{ }=\text{ }1,\text{ }2,\text{ }......,n\] .
If the events \[{{E}_{1}},\text{ }{{E}_{2}},\text{ }...,\text{ }{{E}_{n}}\] are pairwise disjoint, exhaustive, and have nonzero probabilities, they represent a partition of the sample space \[S\].
Theorem of Total Probability:
Let {\[{{E}_{1}},\text{ }{{E}_{2}},\text{ }...,\text{ }{{E}_{n}}\]} be a partition of the sample space \[S\],
Suppose that each of the events \[{{E}_{1}},\text{ }{{E}_{2}},\text{ }...,\text{ }{{E}_{n}}\]has nonzero probability of occurrence.
Let \[A\] be the any event linked with \[S\], then
$P(A)=P({{E}_{1}})P(A|{{E}_{1}})+P({{E}_{2}})P(A|{{E}_{2}})......+P({{E}_{n}})P(A|{{E}_{n}})$
$=\sum\limits_{j=1}^{n}{P({{E}_{j}})P(A|{{E}_{f}})}$
Proof:
We have given that \[{{E}_{1}},\text{ }{{E}_{2}},\text{ }...,\text{ }{{E}_{n}}\]is a partition of the sample space \[S\]. Therefore,
$S={{E}_{1}}\cup {{E}_{2}}\cup ....\cup {{E}_{n}}$
And
${{E}_{i}}\cap {{E}_{j}}=\Phi ,i\ne j,i,j=1,2,......,n$
Now, we know that for any event $A$ ,
$A=A\cap S$
$=A\cap ({{E}_{1}}\cup {{E}_{2}}\cup ....\cup {{E}_{n}})$
$=(A\cap {{E}_{1}})\cup (A\cap {{E}_{2}})\cup ....\cup (A\cap {{E}_{n}})$
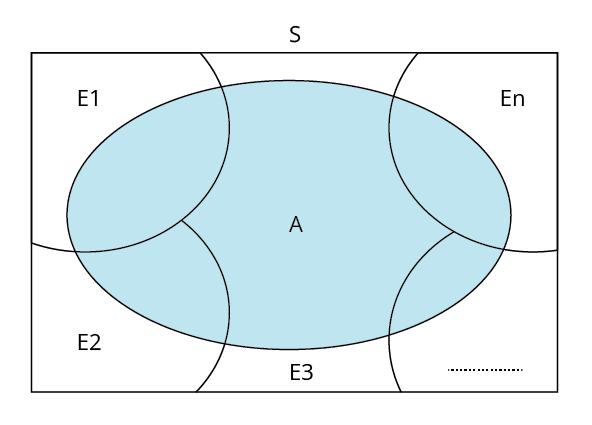
Also $A\cap {{E}_{i}}$ and $A\cap {{E}_{j}}$ are respectively the subsets of ${{E}_{i}}$ and ${{E}_{j}}$. We know that ${{E}_{i}}$ and ${{E}_{j}}$are disjoint, for $i\ne j$ , therefore, $A\cap {{E}_{i}}$ and $A\cap {{E}_{j}}$are also disjoint for all $i\ne j,i,j=1,2,......,n$.
Thus ,
$P(A)=P[(A\cap {{E}_{1}})\cup (A\cap {{E}_{2}})\cup ....\cup (A\cap {{E}_{n}})$
$=P(A\cap {{E}_{1}})+(A\cap {{E}_{2}})+....+(A\cap {{E}_{n}})$
Now, by multiplication rule of probability, we get
$P(A\cap {{E}_{i}})=P({{E}_{i}})P(A|{{E}_{i}})$ as $P({{E}_{i}})\ne 0\forall i=1,2,....,n$
Therefore,
$P(A)=P({{E}_{1}})P(A|{{E}_{1}})+P({{E}_{2}})P(A|{{E}_{2}})+.....+P({{E}_{n}})P(A|{{E}_{n}})$
Or
$P(A)=\sum\limits_{j=1}^{n}{P({{E}_{f}})P(A|{{E}_{f}})}$
Bayes' Theorem: Proof:
If \[{{E}_{1}},\text{ }{{E}_{2}},\text{ }...,\text{ }{{E}_{n}}\]are n non empty events that make up a partition of sample space S, i.e. \[{{E}_{1}},\text{ }{{E}_{2}},\text{ }...,\text{ }{{E}_{n}}\]are pairwise disjoint and \[{{E}_{1}}\cup {{E}_{2}}\cup ...\cup {{E}_{n}}=S\] and \[A\] is any event with a probability greater than zero, then
$P({{E}_{I}}|A)=\dfrac{P({{E}_{J}})P(A|{{E}_{i}})}{\sum\limits_{j=1}^{n}{P({{E}_{J}})P(A|{{E}_{j}})}}$ for any $i=1,2,3,....,n$
Proof:
We can conclude from the conditional probability formula that
$P({{E}_{i}}|A)=\dfrac{P(A\cap {{E}_{i}})}{P(A)}$
$=\dfrac{P({{E}_{i}})P(A|{{E}_{i}})}{P(A)}$ (By the probability multiplication rule)
$=\dfrac{P({{E}_{i}})P(A|{{E}_{i}})}{\sum\limits_{j=1}^{n}{P({{E}_{j}})P(A|{{E}_{j}})}}$ (As a result of the total probability theorem)
Remark:
When Bayes' theorem is employed, the following nomenclature is commonly used.
Hypotheses are occurrences such as \[{{E}_{1}},\text{ }{{E}_{2}},\text{ }...,\text{ }{{E}_{n}}\].
The priori probability of the hypothesis \[Ei\] is \[P\left( Ei \right)\].
The posteriori probability of the hypothesis \[Ei\] is the conditional probability \[P\left( Ei|A \right)\].
The formula for the likelihood of "causes" is also known as the "causes formula." Because the \[Ei\]'s are a subset of the sample space \[S\], only one of the \[Ei\]'s happens (that is one of the events \[Ei\] must occur and only one can occur). As a result, provided that event \[A\] has occurred, the foregoing formula provides us the likelihood of a specific \[Ei\].
Random Variables and its Probability Distributions:
As illustrated in the following examples/experiments, we were not only interested in the specific outcome that occurred in most random experiments in Sample space, but also in the number connected with that outcome.
Experiments:
While tossing two dice, we can be interested in the sum of the numbers on the two dice.
We might want to know how many heads we got by tossing a coin $10$ times
In the experiment of randomly selecting four articles (one after the other) from a batch of \[30\] articles, \[15\] of which are defective, we want to know the number of defectives in the sample of four, not in the precise sequence of defective and non-defective products.
In all of the aforementioned experiments,
We have a rule that allocates a single real number to each experiment's conclusion.
This single real number may change depending on the experiment's outcome. As a result, it is a variable.
Its value is also determined by the outcome of a random experiment, which is why it is referred to as a random variable.
\[X\] is commonly used to represent a random variable.
Because a random variable can have any real value, the set of real numbers is its co-domain. As a result, a random variable is defined as follows:
The sample space of a random experiment is the domain of a random variable, which is a real-valued function.
For instance, consider the experiment of tossing a coin thrice in a row.
Sample space of the experiment is \[S=\left\{ HH,\text{ }HT,\text{ }TH,\text{ }TT \right\}.\]
If \[X\] signifies the number of heads obtained, then \[X\] is a random variable with the following values for each outcome:
\[X\left( HH \right)\text{ }=\text{ }2,\text{ }X\text{ }\left( HT \right)\text{ }=\text{ }1\] , \[X\text{ }\left( TH \right)\text{ }=\text{ }1,\text{ }X\text{ }\left( TT \right)\text{ }=\text{ }0\]
Let \[Y\] denote the number of heads minus the number of tails for each outcome of the above sample space \[S\].
For each outcome of the above sample space \[S\], let \[Y\] signify the number of heads minus the number of tails.
\[Y\text{ }\left( HH \right)\text{ }=\text{ }2,\text{ }Y\text{ }\left( HT \right)\text{ }=\text{ }0\] ,\[Y\text{ }\left( TH \right)\text{ }=\text{ }0,\text{ }Y\text{ }\left( TT \right)\text{ }=\text{ }\text{ }2\].
As a result, \[X\] and \[Y\] are two separate random variables defined on the same sample space \[S\] .
Note: On the same sample space, many random variables can be defined.
A Random Variable's Probability Distribution:
The probability distribution of the random variable \[X\] is a description that offers the random variable's values as well as the probabilities associated with it.
In general, a random variable's probability distribution is defined as follows:
The system of numbers is the probability distribution of a random variable \[X\].
$X:{{x}_{1}}{{x}_{2}}.......{{x}_{n}}$
$P(X):{{P}_{1}}{{P}_{2}}.......{{P}_{n}}$
where,
${{p}_{i}}>0,\sum\limits_{i=1}^{n}{{{p}_{i}}}=1,i=1,2,......,n$
The real numbers ${{x}_{1}},{{x}_{2}},....,{{x}_{n}}$ are the possible vales of the random variable $X$ and ${{p}_{i}}(i=1,2,...,n)$is the probability of the random variable $X$taking the value ${{x}_{i}}$ that is,
$P(X={{x}_{i}})={{p}_{i}}$
All elements of the sample space are also covered for all potential values of the random variable \[X\].
As a result, the total probability in a probability distribution must equal one.
Only at certain points (\[s\]) in the sample space is \[X={{x}_{i}}\] true.
As a result, the probability that \[X\] takes the value \[{{x}_{i}}\] is never \[0\] , that is
\[P\left( X=xi \right)\ne 0\].
Mean of a Random Variable:
In the sense that it roughly locates the random variable's middle or average value, the mean is a measure of central tendency.
Consider \[X\] be a random variable with the possible values \[{{x}_{1}},\text{ }{{\text{x}}_{2}},\text{ }{{\text{x}}_{3}},...,\text{ }{{\text{x}}_{n}}\]occuring with probabilities \[{{p}_{1}},\text{ }{{p}_{2}},\text{ }{{p}_{3}},...,\text{ }{{p}_{n}}\] , respectively.
The mean of \[X\], denoted by\[\mu \], is the number$\sum\limits_{i=1}^{n}{{{x}_{i}}{{p}_{i}}}$ that is the mean of \[X\] is the weighted average of the possible values of \[X\], each value being weighted by its probability with which it occurs.
The mean of \[X\] , represented by \[\mu \] , is the number $\sum\limits_{i=1}^{n}{{{x}_{i}}{{p}_{i}}}$ that is the mean of \[X\] is the weighted average of the possible values of \[X\] , with each value being weighted by its probability of occurrence.
The expectation of \[X\] is also known as it’s mean and it’s denoted by \[E\left( X \right)\].
Thus,
$E(X)=\mu
=\sum\limits_{i=1}^{n}{{{x}_{i}}{{p}_{i}}}={{x}_{1}}{{p}_{1}}+{{x}_{2}}{{p}_{2}}+....+{{x}_{n}}{{p}_{n}}$
The sum of all possible values of by their respective probabilities is the mean or expectation of a random variable \[X\] .
Variance of a Random Variable:
The mean of a random variable provides no information regarding the variability of the random variable's values.
If the variance is small, the random variable's values are near to the mean.
Random variables with different probability distributions, as demonstrated in the distributions of \[X\] and \[Y\] , can have equal means, as illustrated in the following distributions.
$X$ | $1$ | $2$ | $3$ | $4$ |
$P(X)$ | $\dfrac{1}{8}$ | $\dfrac{2}{8}$ | $\dfrac{3}{8}$ | $\dfrac{4}{8}$ |
$Y$ | $-1$ | $0$ | $4$ | $5$ | $6$ |
$P(Y)$ | $\dfrac{1}{8}$ | $\dfrac{2}{8}$ | $\dfrac{3}{8}$ | $\dfrac{1}{8}$ | $\dfrac{1}{8}$ |
$E(X)=1\times \dfrac{1}{8}+2\times \dfrac{2}{8}+3\times \dfrac{3}{8}+4\times \dfrac{2}{8}$
$E(X)=\dfrac{22}{8}=2.75$
$E(Y)=-1\times \dfrac{1}{8}+0\times \dfrac{2}{8}+4\times \dfrac{3}{8}+5\times \dfrac{1}{8}+6\times \dfrac{1}{8}$
$E(Y)=\dfrac{22}{8}=2.75$
Although the variables \[X\] and \[Y\] are distinct, their means are the same.
Below is a diagrammatic representation of these distributions.
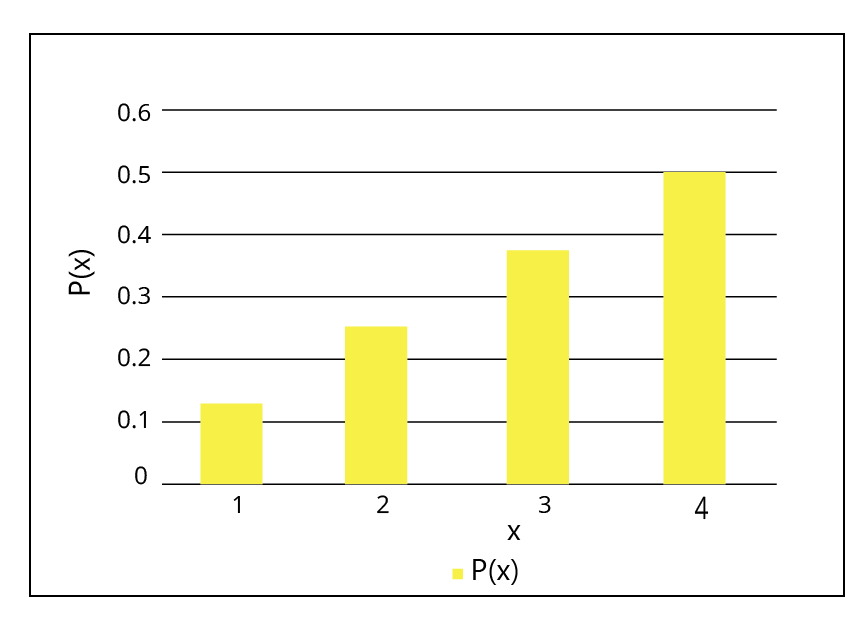
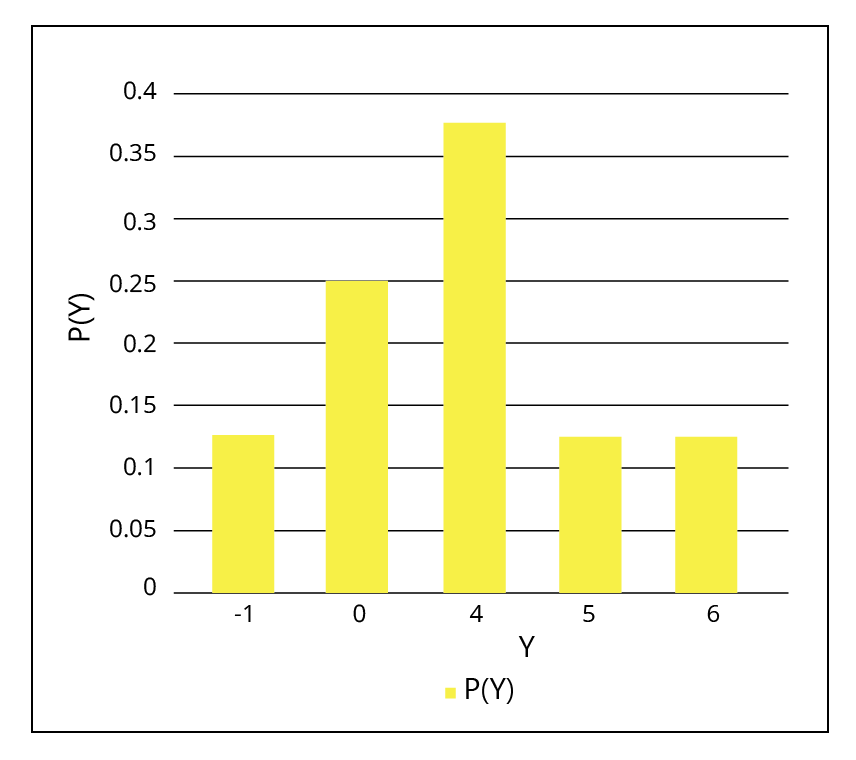
Assume that \[X\] is a random variable with possible values \[{{x}_{1}},\text{ }{{x}_{2}},...,{{x}_{n}}\] and possible probabilities \[p({{x}_{1}}),\text{ }p\left( {{x}_{2}} \right),...,\text{ }p\left( {{x}_{n}} \right)\] respectively.
Let $\mu =E(X)$ be the mean of \[X\].
The variance of \[X\], denoted by $Var(X)$ or $\sigma _{x}^{2}$ is defined as
$\sigma _{x}^{2}=Var(X)=\sum\limits_{i=1}^{n}{{{({{x}_{i}}-\mu )}^{2}}p({{x}_{i}})}$
$\sigma _{x}^{2}=E{{(X-\mu )}^{2}}$
or equivalently
$\sigma_{x}^{2}=\sqrt{Var(X)}=\sqrt{\sum\limits_{i=1}^{n}{{{({{x}_{i}}-\mu )}^{2}}p({{x}_{i}})}}$
The non- negative number is known as the standard deviation of the random variable.
The variance of a random variable can be calculated as;
We know that,
$Var(X)=\sum\limits_{i=1}^{n}{{{({{x}_{i}}-\mu )}^{2}}p({{x}_{i}})}$
$=\sum\limits_{i=1}^{n}{({{x}_{i}}^{2}+{{\mu}^{2}}-2\mu {{x}_{i}})p({{x}_{i}})}$
$=\sum\limits_{i=1}^{n}{{{x}_{i}}^{2}p({{x}_{i}})}+\sum\limits_{i=1}^{n}{{{\mu }^{2}}p({{x}_{i}})}-\sum\limits_{i=1}^{n}{2\mu {{x}_{i}}p({{x}_{i}})}$
$=\sum\limits_{i=1}^{n}{{{x}_{i}}^{2}p({{x}_{i}})}+{{\mu}^{2}}\sum\limits_{i=1}^{n}{p({{x}_{i}})}-2\mu \sum\limits_{i=1}^{n}{{{x}_{i}}p({{x}_{i}})}$
$=\sum\limits_{i=1}^{n}{{{x}_{i}}^{2}p({{x}_{i}})}+{{\mu}^{2}}-2\mu $$\left[ \text{since }\sum\limits_{i=1}^{n}{p({{x}_{i}})}=1\text{ and }\mu =\sum\limits_{i=1}^{n}{{{x}_{i}}p({{x}_{i}})} \right]$
$=\sum\limits_{i=1}^{n}{x_{i}^{2}p({{x}_{i}})-{{\mu }^{2}}}$
or
$Var(X)=\sum\limits_{i=1}^{n}{{{x}_{i}}^{2}p({{x}_{i}})}-{{\left( \sum\limits_{i=1}^{n}{{{x}_{i}}p({{x}_{i}})} \right)}^{2}}$
or
$Var(X)=E({{X}^{2}})-{{[E(X)]}^{2}}$
Where,
$E({{X}^{2}})=\sum\limits_{i=1}^{n}{{{x}_{i}}^{2}p({{x}_{i}})}$
Bernoulli Trials and Binomial Distribution
Bernoulli Trials:
The results of any trial are unrelated to the results of other trials.
The chance of success or failure in each of these trials is the same.
Bernoulli trials are independent trials with only two outcomes, commonly referred to as "success" or "failure."
Random experiment trials are called Bernoulli trials if they meet the following criteria:
There should be a limited number of trials, each of which should be independent of one another.
Each experiment can only result in one of two outcomes: success or failure.
In each trial, the probability of success are the same.
Example:
Seven balls are drawn in a row from an urn that contains eight red and ten black balls. Determine whether the drawing ball trials are Bernoulli trials when the drawn ball is
(i) replaced
(ii) not replaced in the urn after each draw.
Ans.:
The number of trials is finite.
When the drawing of red ball is done with replacement, the probability of success (that is drawing of red ball) is
\[p=\dfrac{8}{18}\]
This is true for each of the seven trials (draws).
As a result, Bernoulli trials are used to draw balls with replacements.
The probability of success (drawing of red ball) in the first trial is \[\dfrac{8}{18}\] when the drawing is done without replacement.
If the red ball drawn is first, the second trial is \[\dfrac{7}{17}\] ; if the first ball drawn is black, the second trial is \[\dfrac{8}{17}\], and so on.
The probability of success is clearly not the same for all trials and hence they are not Bernoulli trials.
The Binomial Distribution:
The binomial expansion of the probability distribution of the number of successes in an experiment consisting of \[n\] Bernoulli trials may be used to derive the probability distribution of the number of successes in an experiment consisting of \[n\] Bernoulli trials \[{{\left( q\text{ }+\text{ }p \right)}^{n}}\].
As a result, this distribution of success numbers \[X\] can be represented as
$X$ | $0$ | $1$ | $2$ | … | $x$ | … | $n$ |
$P(X)$ | $^{n}{{C}_{0}}{{q}^{n}}$ | $^{n}{{C}_{1}}{{q}^{n-1}}{{p}^{1}}$ | $^{n}{{C}_{2}}{{q}^{n-2}}{{p}^{2}}$ | $^{n}{{C}_{x}}{{q}^{n-x}}{{p}^{x}}$ | $^{n}{{C}_{n}}{{q}^{n}}$ |
The above probability distribution is known as a binomial distribution with parameters \[n\] and \[p\] , because we can determine the whole probability distribution for given values of \[n\] and \[p\].
The probability of \[x\] successes \[P\left( X\text{ }=\text{ }x \right)\] is also denoted by \[P\left( x \right)\] and is given by
$P(x){{=}^{n}}{{C}_{x}}{{q}^{n-x}}{{p}^{x}}$
$x=0,1,....,n.$
$(q=1-p)$
This \[P\left( x \right)\] is called the probability function of the binomial distribution.
A binomial distribution with \[n-\] Bernoulli trials and probability of success in each trial as \[p\], is denoted by \[B\left( n,p \right)\].
Probability Notes Class 12
Experiment: An experiment is an operation that results in well-defined results.
Random Experiment: A random experiment is an experiment in which the outcome may not be the same even if experimenting in identical conditions.
Sample Space: A collection of all possible outcomes is called sample space. Generally, it is represented by S. For example, suppose an unbiased coin is tossed, then either heads or tails will come. Then the sample space will be {H, T} where H represents the heads and T represents the tails.
Event: Let us have a random experiment and sample space associated with it then subsets of sample space are called events. For example in throwing a die and getting either 1, 2, 3, 4, 5, or 6.
Equally Likely Events: It is a relative property of two events. Two events are said to be equally likely if none of them is expected to occur in preference to the other.
E.g. In throwing an unbiased coin, heads and tails are equally likely to come.
Mutually Exclusive Events: A n-number of events is said to be mutually exclusive if the occurrence of one excludes the happening of the other, i.e. if A and B are mutually exclusive, then (A ∩ B) = Φ.
For example, suppose a die has been thrown, all numbers from 1 to 6 are mutually exclusive because the occurrence of one rule puts the chances of occurrence of other numbers.
Exhaustive Events: A n-number of events is said to be exhaustive if all events collectively make the sample space. In other words, the performance of the experiment always results in the occurrence of at least one of them.
Let E1, E2, E3....En are exhaustive events, then by definition, we have E1⋃ E2⋃ E3.....En = S.
For Example: when we toss two coins, the exhaustive number of cases is 4. Since any of the heads and tails of the first coin can be associated with any of the heads and tails of the other coin.
The Complement of an Event: Let S be a sample space and B be an event in it. The complement of is represented by B’ or B̅ and B’= {x: x ∈ S, x ∉ B}.
What is the Probability of an Event?
As we have already mentioned at the beginning of the article, probability measures the uncertainty of happening something. Mathematically it’s a ratio. Whose values range between 0 to 1. Let E be an event then the probability of E can be calculated as:
P(E) = (Number of favourable cases to E)/(Total number of exhaustive cases) = [n(A)]/[n(S)] = m/n
Properties of Probability
(i) Probability of any event will be between 0 and 1. That is 0 ≤ P(A) ≤ 1.
(ii) If the calculated Probability of an event is zero then it means that event is impossible to happen.
(iii) If the calculated Probability of an event is one then it means that event will surely happen.
(iv) Probability of an event and its complement is equal to the probability of sample space, which is 1. That is P(E ∪ E’) = P(S).
(v) Probability of intersection of an event and its complement is equal to the probability of null set. Which is zero. That is P(E ∩ E’) = P(Φ).
(vi) P(E’)’ = P(E).
(vii) P(X ∪ Y) = P(X) + P(Y) – P(X ∩ Y).
Conditional Probability
Let us consider A and B are two events associated with a sample space S of a random experiment. The probability of occurrence of event A, when event B has already occurred, is called the conditional probability of event A over event B. It is denoted by P(A/B).
Its formula is
P(A/B) = [P(A∩B)]/P(B) where P(B) is not equal to zero. As we have already mentioned that it has already occurred.
Similarly, we can define the conditional probability of event B over event A.
P(A/B) = [P(B∩A)]/P(B)
Independent Event: Let A and B are two events. They are said to be independent if the probability of occurrence or non-occurrence of either of them doesn’t affect the probability of others.
For any two independent events A and B, we have the relation.
P(A ∩ B) = P(A) . P(B)
Many students have the misconception that independent events and mutually exclusive events are the same but it is not the case. They have a different meaning.
Total Probability Theorem
Let A1, A2, A3...An are events that are a partition of sample space S of an experiment. If X is an event associated with the sample space S, then:
\[P(X) = \int_{i=1}^{n} P(A_{i}) P(X/A_{i})\]
Conclusion:
Probability is one of the scoring topics in mathematics, and questions are very basic and simple, Probability and statistics cover an overall weightage of 11 to 15 marks, Main questions will come from topics including Conditional Probability, Properties of Conditional Probability, Multiplication Theorem, Bayes Theorem, and Probability Distributions, Theorem of Total Probability, etc. Try to practice maximum questions to get good marks in the board examination.
Revision Notes for Other Chapters
Find here CBSE Class 12 Maths revision notes for other chapters:
Other Related Links
FAQs on Probability Class 12 Notes CBSE Maths Chapter 13 [Free PDF Download]
1. What is the Multiplication Rule of Probability? What is the Multiplication Rule for Three Events?
Let suppose that A and B are two events associated with a sample space A, then the probability of occurrence simultaneously of the events A and B is:
P(A ∩ B) = P(A) × P(B/A), where P(F) ≠ 0.
This rule is termed a multiplication rule of probability.
The multiplication rule can be generalized for Three Events: If A, B, and C are three events of sample space, then:
P(A∩B∩C) = P(A) × P(B/A) × P(C/A∩B)
2. State Bayes Theorem.
Let us suppose A1, A2, A3...An are non-empty events. These events partition the sample space S. That is A1, A2, A3...An are pairwise disjoint and A1ሀA2ሀA3...ሀAn = S. Also, each Ai > 0 ∀ i = 1, 2, 3, ...n. Also, let X be a non-zero event then the probability:
P(Ai/X) = [P(Ai) × P(X/Ai)]/[Σi=1n P(Ai) P(X/Ai)]
3. What is Booley’s Inequality?
Let us suppose E1, E2, E3,...En are events associated with a sample space S of an experiment. Then according to Booley’s inequality:
(a) P(E1∩E2∩E3∩...∩En) ≥ Σi=1n P(Ei) - (n - 1)
(b) P(⋃i=1n Ei) ≤ Σi=1n P(Ei)
4. Can I download the Notes for Chapter 13 Probability of Class 12 Maths in PDF?
Yes. Students can download the notes of the chapter probability from the official website of Vedantu. Open the Vedantu website, select study material and then CBSE. Under the CBSE, select Revision notes and then Class 12 revision notes. Students will be directed to a new page where they can find all the chapters of Maths for Class 12. Select Chapter 13- Probability. Students now can view the notes offline or download them in PDF format.
5. How many questions are there in Chapter 13 Probability of Class 12 Maths?
There are a total of five exercises containing 81 questions in Class 12 Maths Chapter 13 Probability. All the questions test the students how much they have understood the topics. Students can plan a strategy to make their preparation easier and gain a strong foundation on the topics by analyzing the number of problems given in each section. The format of these solutions is in such a way that students gain a sound knowledge of all the important concepts and basics based on conditional probability.
6. What are the important topics covered in Chapter 13 Probability of Class 12 Maths?
Chapter 13 Probability of Class 12 Maths explains to the students all the significant properties and theorems of conditional probability. The important topics that are covered in this chapter are based on the theorems and terms related to conditional probability such as multiplication theorem on probability, Bayes’ theorem, mean and variance of a random variable, independent events, total probability, random variables, and its probability distribution. Solving all the questions based on these will help the students to improve their fundamental knowledge of probability. The main aim of framing such solutions is to help the students in their studies by promoting math proficiency.
7. What are the importance of NCERT Solutions and revision notes for Chapter 13 Probability of Class 12 Maths?
NCERT Solutions and revision notes for Chapter 13 Probability of Class 12 Maths are very important to study materials as it makes the students gain a clear knowledge of conditional probability. It will help the students to understand the various applications of probability in different fields. The format of these solutions is framed in such a way that it will help to raise the mathematical learning and confidence in students. Practising the questions with these resources makes the students gain a sense of self-accomplishment and confidence which is required to face various competitive exams.
These solutions are available on Vedantu's official website(vedantu.com) and mobile app free of cost.
8. Why should I practice NCERT Solutions for Chapter 13 Probability of Class 12 Maths?
NCERT Solutions for Chapter 13 Probability of Class 12 Maths are well created to make the students obtain a deep understanding of the practical applications of probability. Students should practice the sample problems which are provided in these solutions as they are based on the practical observation of probability in our environment. Students can improve their problem-solving skills by working with examples from their daily lives. It will also help them to improve their mental abilities to work around the most difficult situations.
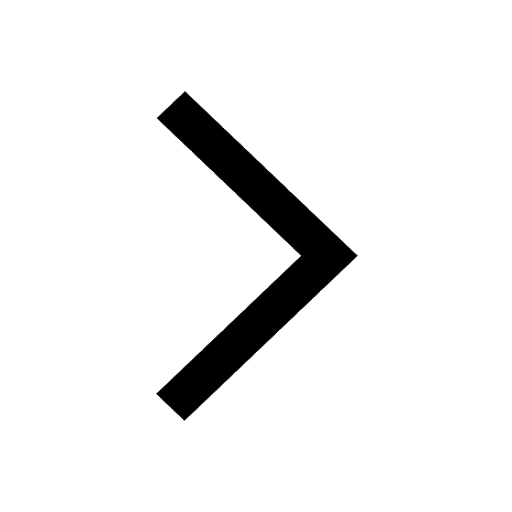
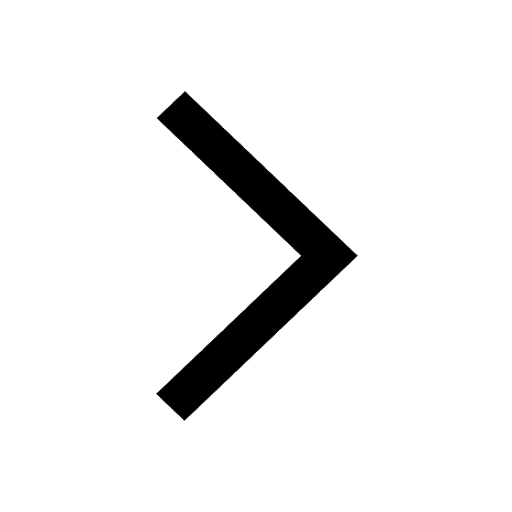
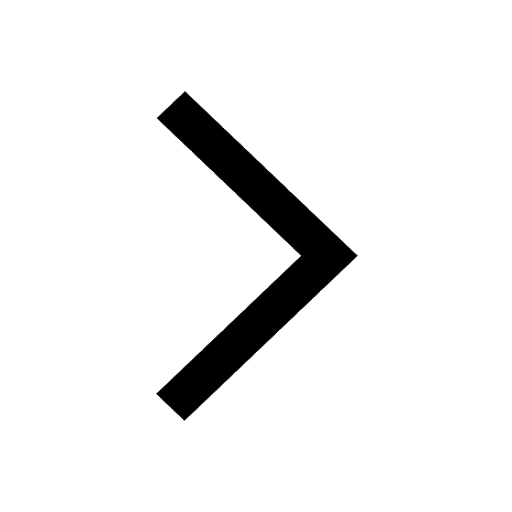
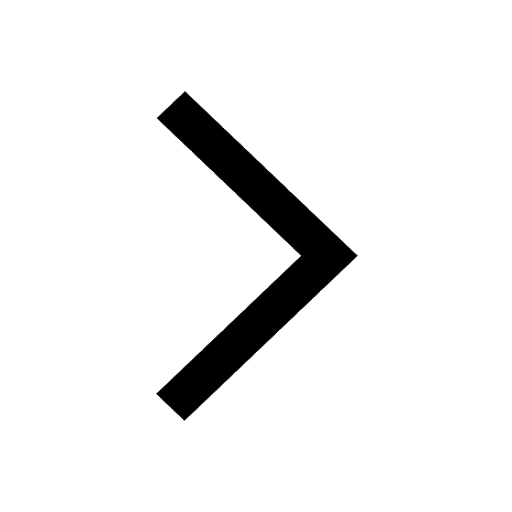
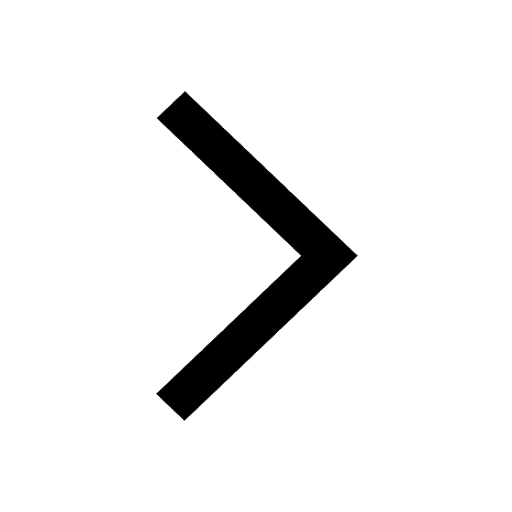
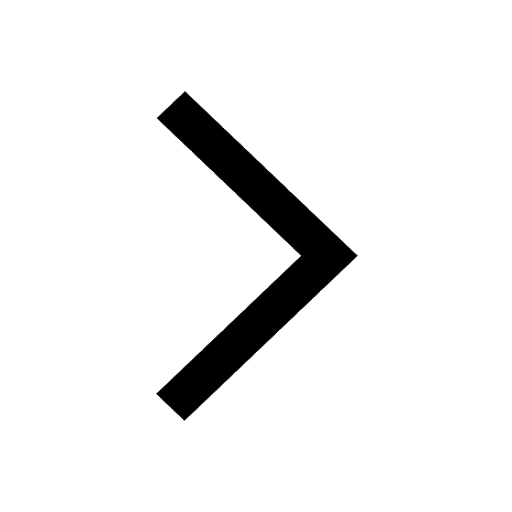
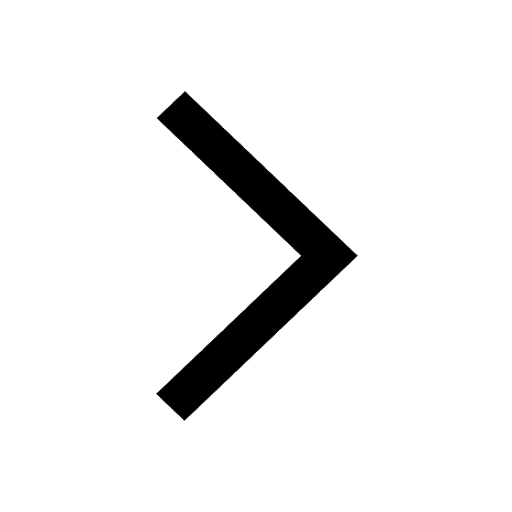
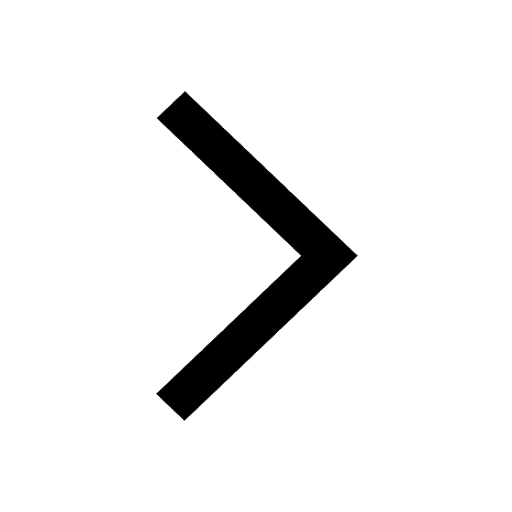
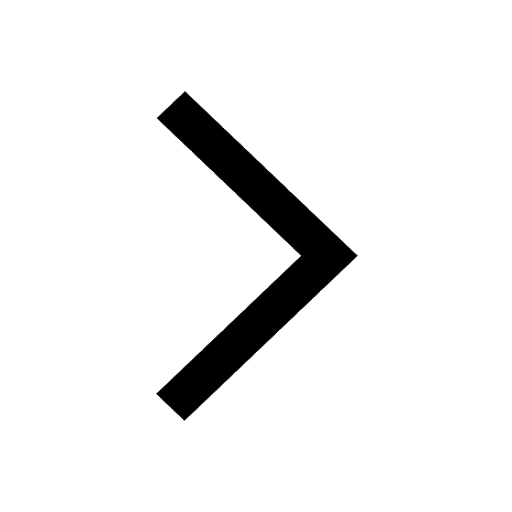
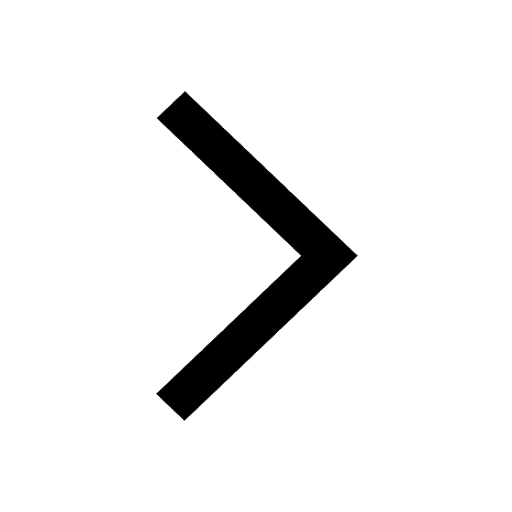
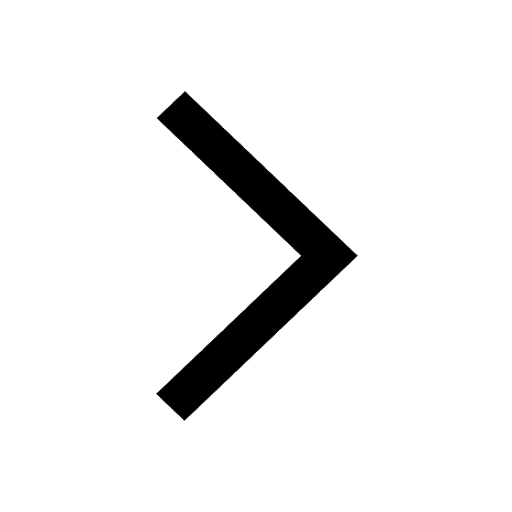
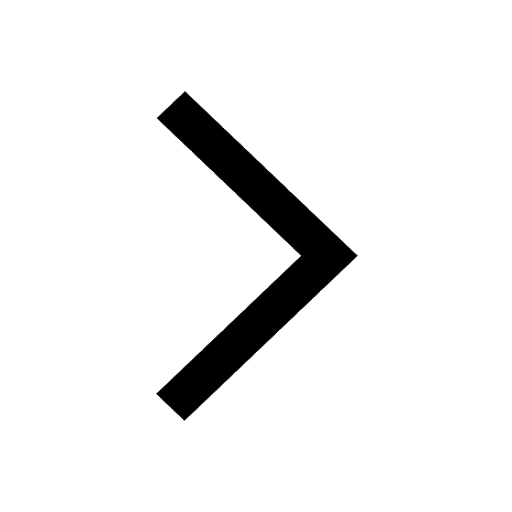
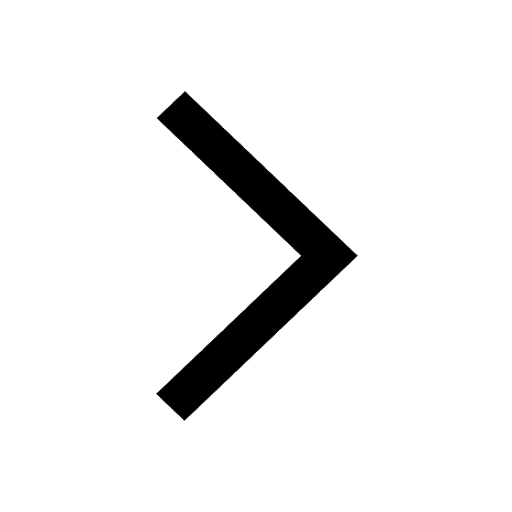
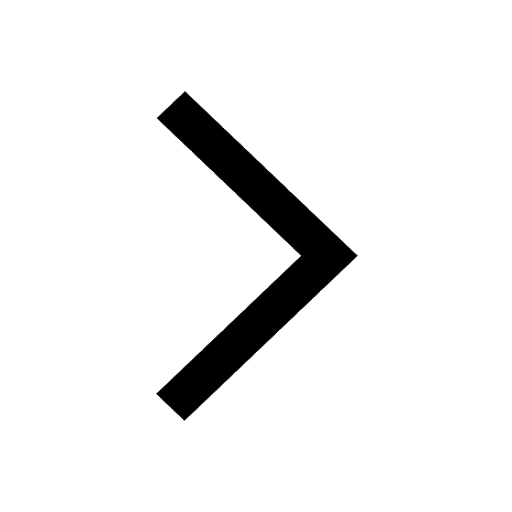
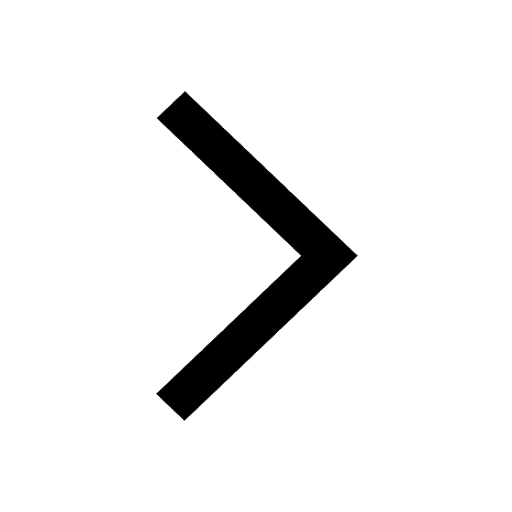
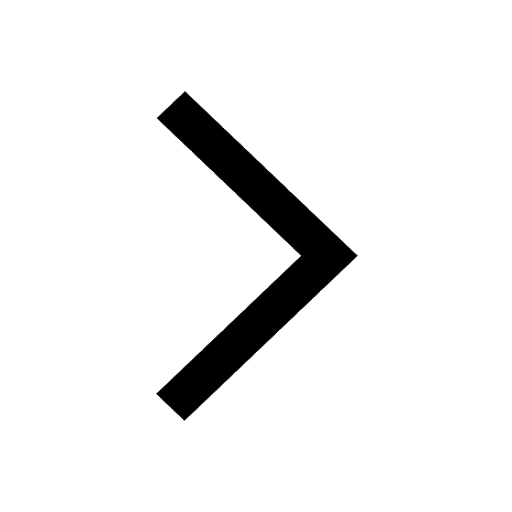
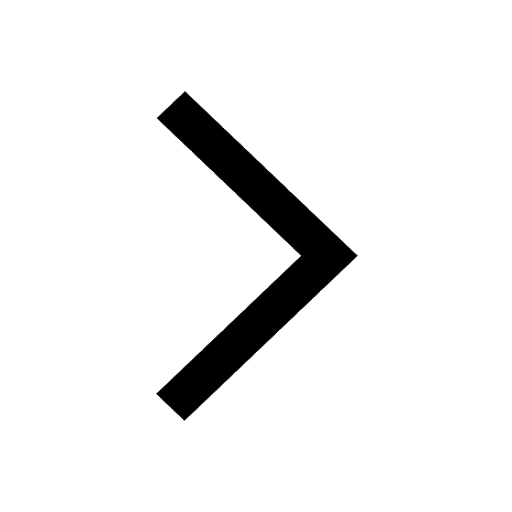
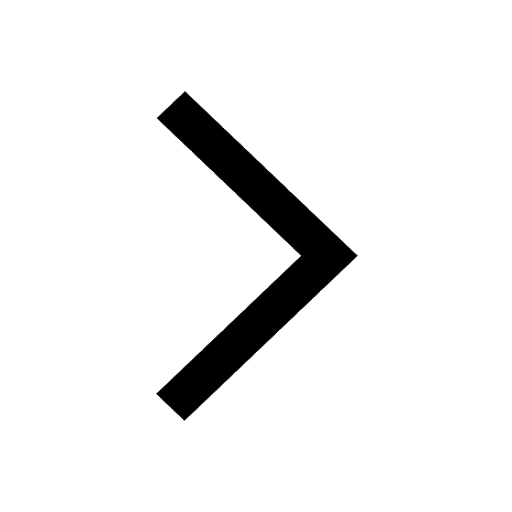
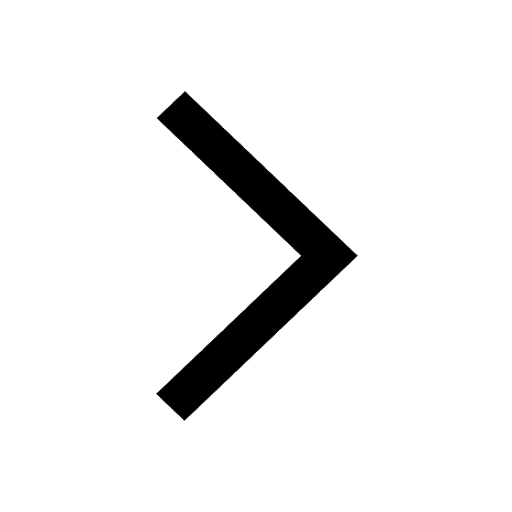
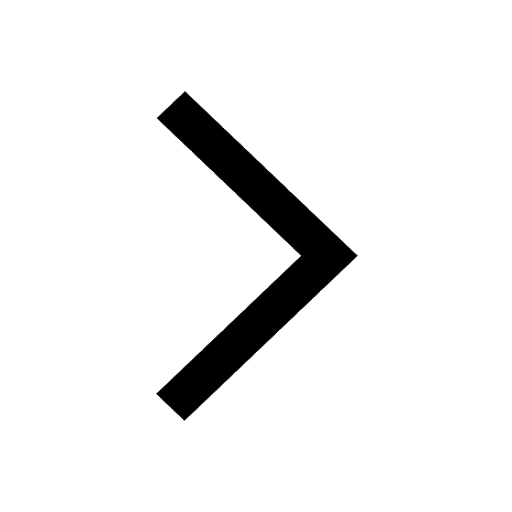
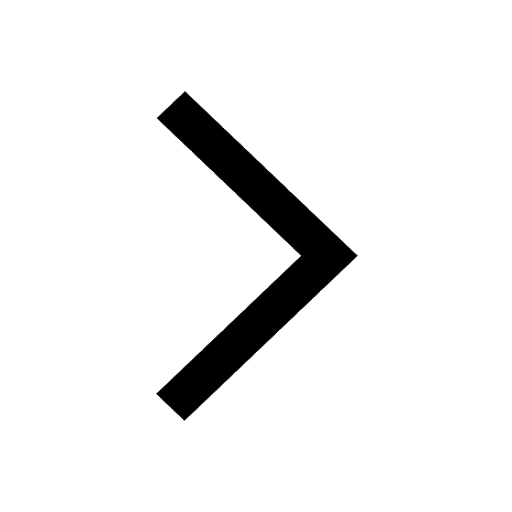