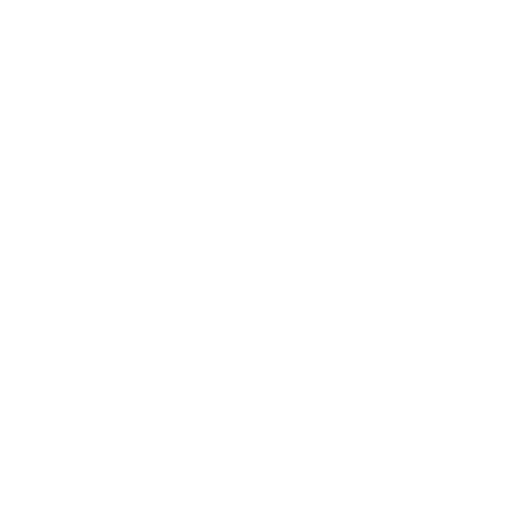
About Multiplication Theorem of Probability
The condition of two events is explained with the help of the multiplication rule probability. Assume two events, J and K, that are associated with a sample space S. When both the J and K events occur, and it is denoted by the set J ∩ K. Hence, the occurrence of both the events J and K are represented by ( J ∩ K ). This event can also be written as JK and using the properties of conditional probability, and you can obtain the probability of event JK. In this article, you will also learn the multiplication theorem of probability.
Proof of Multiplication Rule Probability
Let us now learn the multiplication law of probability.
We know that the conditional probability of an event J given the fact that K has occurred is represented by P ( J | K ), and the formula goes by:
P ( J | K ) = P(J⋂K) / P(K)
Where P ( K ) ≠ 0
P ( J ∩ K ) = P ( K ) × P ( J | K ) ……………………………………..( A )
P ( K | J ) = P ( K ∩ J ) / P ( J )
Where P ( J ) ≠ 0.
P ( K ∩ J ) = P ( J ) × P ( K | J )
Since, P ( J ∩ K ) = P ( K ∩ J )
P ( J ∩ K ) = P ( J ) × P ( K | J ) ………………………………………( B )
From (1) Jnd (2), we get:
P ( J ∩ K ) = P ( K ) × P ( J | K ) = P ( J ) × P ( K | J ) where,
P ( J ) ≠ 0, P ( K ) ≠ 0.
The result that is obtained above is known as the multiplication rule of probability.
For independent events J and K, P( K | J ) = P ( K ). The equation (2) can be modified into,
P( J ∩ K ) = P ( K ) × P ( J )
Multiplication Theorem in Probability
Now that we have learned about the multiplication rules that are implemented in probability, such as;
P ( J ∩ K ) = P ( J ) × P ( K | J ) ; if P ( J ) ≠ 0
P ( J ∩ K ) = P ( K ) × P ( J | K ) ; if P ( K ) ≠ 0
Now, let us focus on learning about the multiplication theorems for independent events of J and K.
The probability of simultaneous occurrence of two independent events will be equal to the product of the probabilities of the events if J and K are two independent events for a random experiment. Hence,
P ( J ∩ K ) = P ( J ) . P ( K )
Now, from understanding the multiplication rule, we know that;
P ( J ∩ K ) = P ( J ) × P ( K | J )
Since J and K events are independent here, we get;
P ( K | J ) = P ( K )
On solving again, we get;
P ( J ∩ K ) = P ( J ) . P ( K )
Hence, proved.
Multiplication Theorem of Probability Examples
1. A bag contains 15 red and 5 blue balls. Without the replacement of the balls, two balls are drawn from a bag one after the other. What is the probability of picking both the balls as red?
Solution:
Let J and K represent the events that the first ball is drawn and the second ball drawn is the red balls.
Now, we have to find P ( J ∩ K ) or P ( JK ).
P ( J ) = P ( the red balls drawn in the first draw ) = 15 / 20
Now, only 14 red balls and 5 blue balls are the ones left in the bag. The conditional probability is that of a Probability of drawing a red ball in the second draw too, where the drawing of the second ball depends on the drawing of the first ball.
Therefore, the conditional probability of K on J will be,
P ( K | J ) = 14 / 19
By using the multiplication rule of probability,
P ( J ∩ K ) = P ( J ) × P ( K | J )
P ( J ∩ K ) = (15 / 20)*(14 / 19)
= 21 / 38
Multiplication Rule of Probability for Independent Events
Independent events are defined as those in which the outcome of one event has no impact on the outcome of another. The probability multiplication method for dependent events may be extended to independent occurrences. We have,
P(A ∩ B) = P(A) P(B | A)
so if the events A and B are independent, then P(B | A) = P(B), and thus, the previous theorem is reduced to P(A ∩ B) = P(A) P(B).
That is, the likelihood of both things occurring at the same time is the product of their probabilities. When events are independent, the particular multiplication rule might be used.
Multiplication Rule of Probability for Dependent Events
Dependent events are ones in which the outcome of one event has an impact on the outcome of another. The possibility of one event is influenced by the occurrence of another. The occurrence of the first event might sometimes influence the likelihood of the second event. For example, if you draw a King from a deck of cards and do not replace it, your chances of obtaining another King are reduced.
From the theorem, we have P(A ∩ B) = P(A) P(B | A), where A and B are independent events. When dealing with dependent events, the general multiplication rule must be applied.
Specific Multiplication Rule
Calculate the combined probability of separate occurrences using the particular multiplication rule. Multiply the odds of the separate events to apply this rule. The occurrence of event A has no impact on the likelihood of event B in the case of independent occurrences. The following is the particular multiplication rule in probability notation:
P(A ∩ B) = P(A) * P(B)
Alternatively, the combined likelihood of A and B happening is equal to the probability of A happening multiplied by the probability of B happening.
General Multiplication Rule
Calculate joint probability for independent or dependent occurrences using the general multiplication rule. When dealing with dependent events, the general multiplication rule is required since it allows you to account for how the occurrence of event A impacts the chance of event B. The general multiplication rule is as follows in standard notation:
P(A ∩ B) = P(A) * P(B|A)
Alternatively, the joint chance of A and B occurring is equal to the likelihood of A happening multiplied by the conditional probability of B happening if A happens.
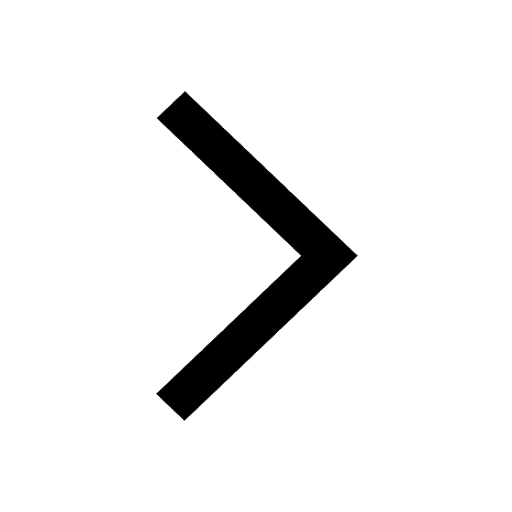
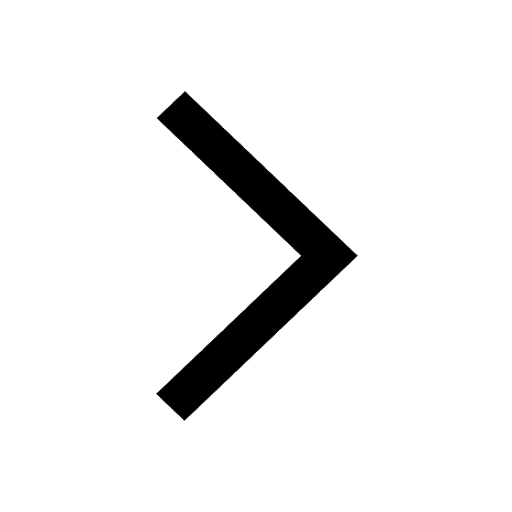
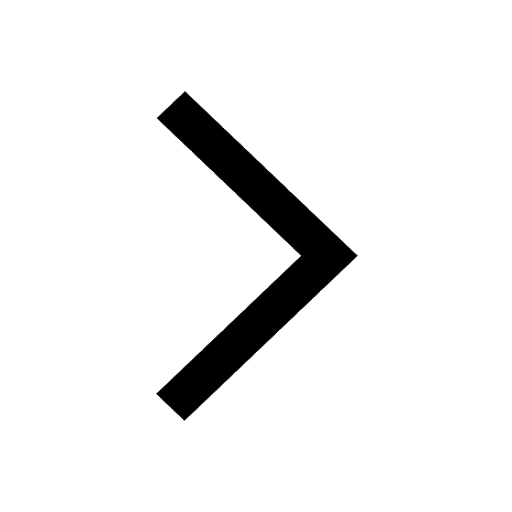
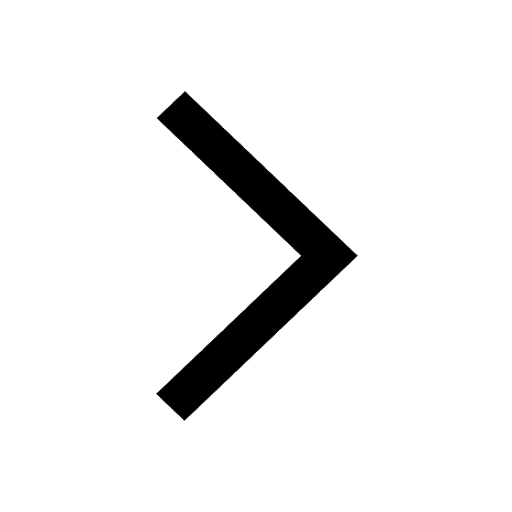
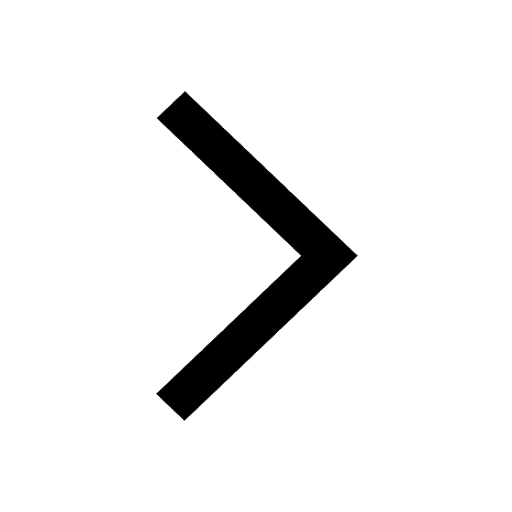
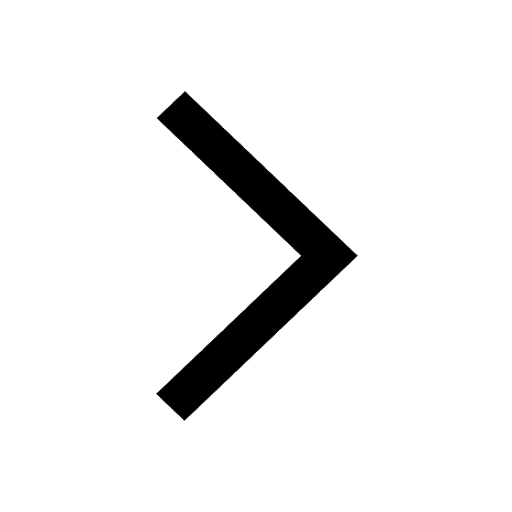
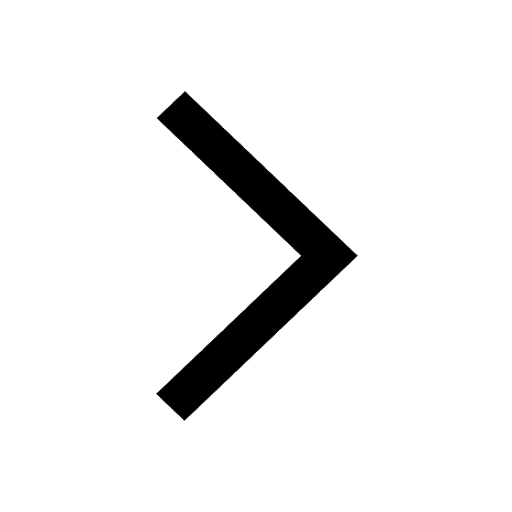
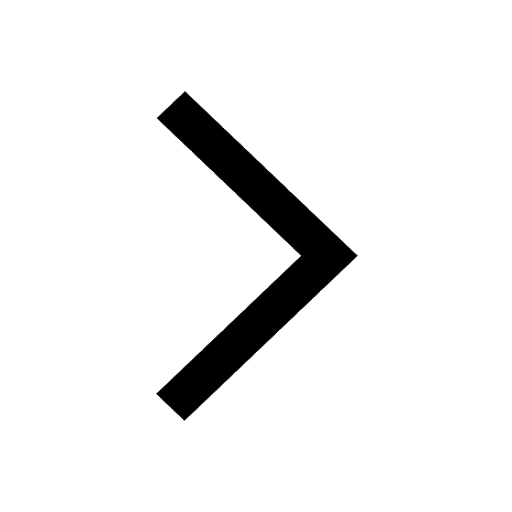
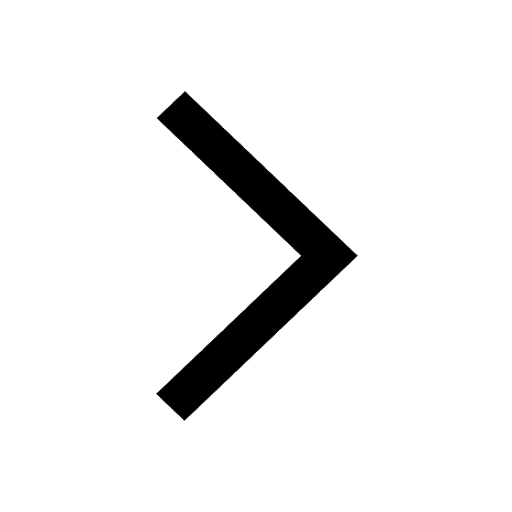
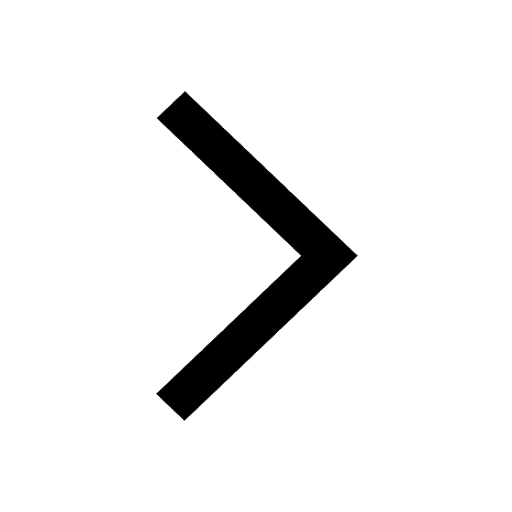
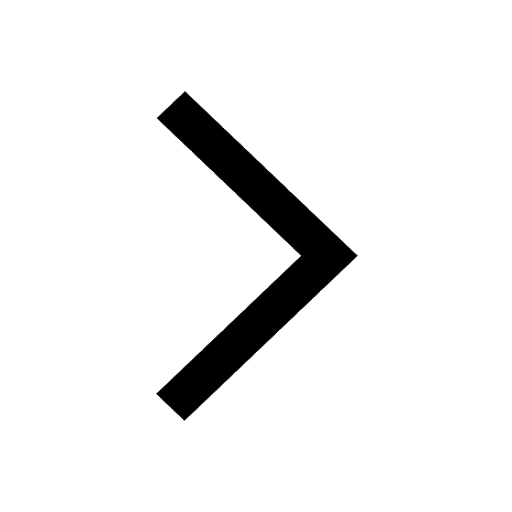
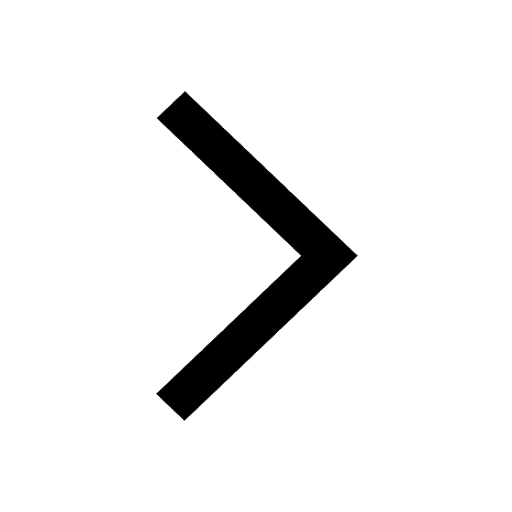
FAQs on Multiplication Theorem of Probability
1. What is the multiplication theorem for Probability?
According to the theorem, "the probability of the simultaneous occurrence of two self-determining events is represented by the product of their distinct probabilities." The condition between two events is explained by the probability multiplication rule. Set A∩B signifies the events in which both events A and B have occurred for two events A and B connected with a sample space S. As a result, (A∩B) signifies the occurrence of occurrences A and B at the same time. Event A∩B is represented as AB. The probability of event AB is calculated using conditional probability characteristics. According to the probability multiplication rule, the likelihood of both events A and B occurring is equal to the product of the probability of B occurring and the conditional probability of event A occurring if event B occurs.
2. What is the law for multiplications?
Multiplication is frequently referred to as repeated addition. For example, the product 3 × 4 is equivalent to the sum of three 4s, 4 + 4 + 4. When it comes to multiplying expressions, there are three key attributes or laws to consider.
Commutative Law of Multiplication - The Commutative of Multiplication is an arithmetic law that states that no matter what sequence you multiply numbers in, the result will always be the same.
Associative Law of Multiplication - It states that no matter how you arrange the numbers you are multiplying together, the result will be the same.
Distributive Law - Every number multiplied by the sum of two or more numbers equals the total of that number multiplied by each of the numbers separately, according to the Distributive Law.
3. What is the Multiplication Rule of Probability Formula?
According to the probability multiplication rule, the likelihood of two occurrences, A and B, happening simultaneously is equal to the probability that B happens multiplied by the conditional probability that A occurs provided that B occurs. By multiplying both sides of the conditional probability definition by the denominator, we get the general multiplication rule.
The multiplication rule can be written as P(A∩B)=P(B)⋅P(A|B).
The universal multiplication rule of probability may be found by simply multiplying both sides of the conditional probability equation by the denominator.