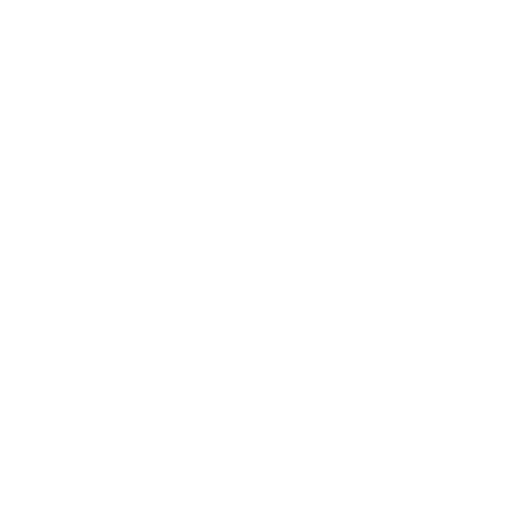
Central Limit Theorem
The Central Limit Theorem (CLT) is an important topic in mathematics. In this article, we will look at the central limit definition, along with all the major concepts that one needs to know about this topic. The central limit theorem can be explained as the mean of all the given samples of a population. This is an approximation if the sample size is large enough and has finite variation.
The central limit theorem can also be explained as the distribution of a sample mean which approximated the normal distribution. This is applicable when the sample size becomes larger. The assumption here is that all the samples are similar and the shape of the population distribution could possibly be anything.
To understand the central limit theorem better, let’s look at an example. Let’s assume that there are ten teams of cricket in your school. Every team has a total of 100 students in it. Now, if we want to measure the average height of all the students in the sports teams, then that would be a humongous task.
The best way to go about this task is to find the heights of all the students and add the values. After that, the sum of heights can be divided by the total number of students. The answer that we arrive at after this step will be the value of average height. This method will be quite tiresome in this example. But can you imagine how hard and extensive a similar task would be with 2000 people?
Due to this reason, this method is not always possible. Thankfully, one can use the central limit theorem to make his or her calculations easy. In the method of the central limit theorem, the individual would start by randomly picking students from different teams. Every sample will include only 20 students. After that, the individual can follow the steps that are mentioned below.
Take all the samples and try to find the mean for every single sample.
Once that is done, then find the mean of the means of the samples.
The value you receive will be the approximate mean height of all the students in the sports teams.
If you plot this data, then you will get a bell curve shape. Check this fact by plotting a histogram of a large sample of mean heights.
Once you are done with the plotting of the histogram, then the shape of your graph should be similar to the image of the graph that is attached below.
(Image will be uploaded soon)
It is important for readers to remember that the sample one takes for plotting the histogram should be sufficiently large. This is because when the sample size is larger, the sample mean distribution will also show normality. This will happen as one goes about calculating the value by using repeated sampling.
Central Limit Theorem Formula
Till now, we have discussed the meaning of the central limit theorem along with what it dictates. The next is to focus on the central limit theorem formula. But before we discuss the formula, it should be noted that the central limit theorem is valid for a large sample size only (n ≥ 30). The formula for the central limit theorem is mentioned below.
μx = μ
σx = σ / √n
In this formula, μ = population mean
σ = population standard deviation
μx = sample mean
σx = sample standard deviation
n = sample size
The Statement and Assumptions of Central Limit Theorem
When it comes to the central limit theorem, then there is a specific statement that students must memorize. The statement is:
The central limit theorem mentions that whenever a random sample of size n is taken from any particular distribution, with mean and variance, then the sample mean would be the approximate normally distributed with both variance and mean. It should be noted that the larger the value of the sample size, the better quality the approximation to the normal.
There are several assumptions that are made in the central limit theorem. Do you know what those assumptions are? If you don’t, then go through the list of those assumptions that are mentioned below.
The final sample should ideally be drawn in a random manner after the condition of randomization.
The sample that is drawn should also be independent of one another. This means that the sample should not influence other samples.
If the sampling is done without any replacement, then the sample size should not exceed 10% of the total population.
The sample size should also be quite large.
Fun Facts About the Central Limit Theorem Application
Do you know about the central limit importance? Do you know about all the different applications of the central limit theorem? According to experts, there are several applications of the central limit theorem. We have created a table of those applications and that table is mentioned below.
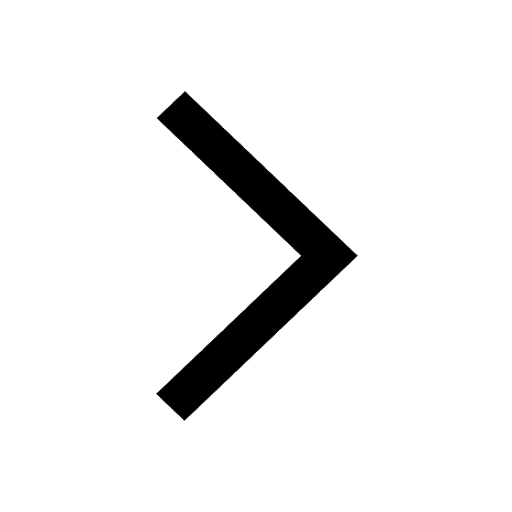
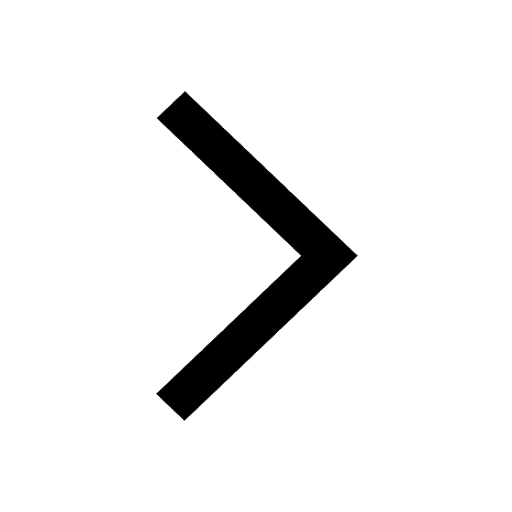
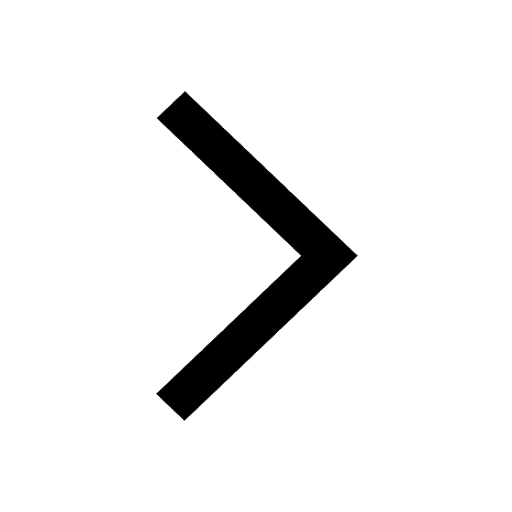
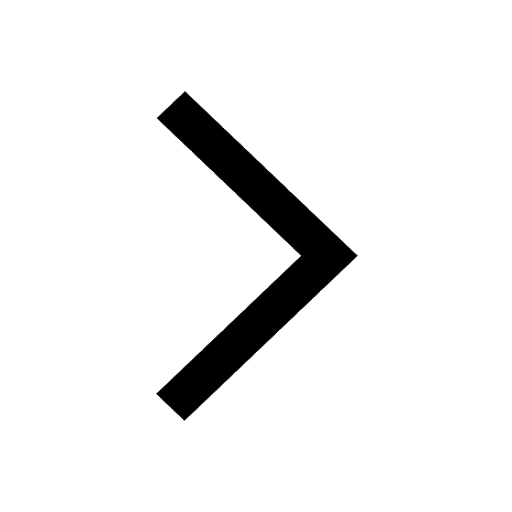
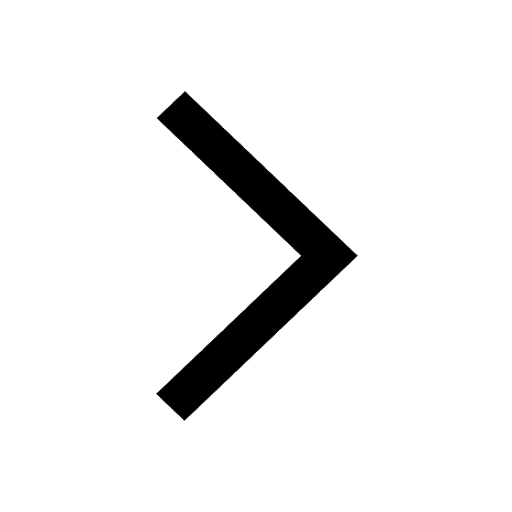
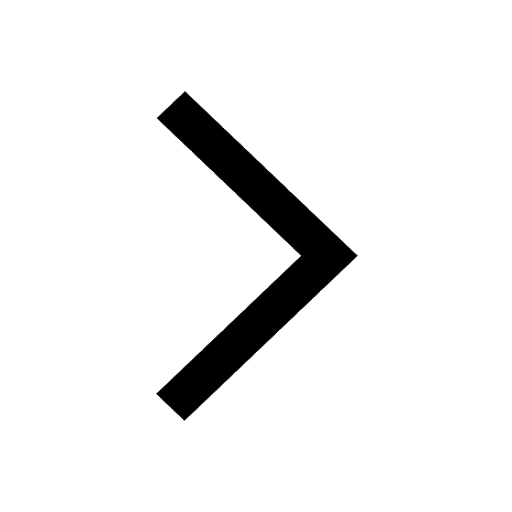
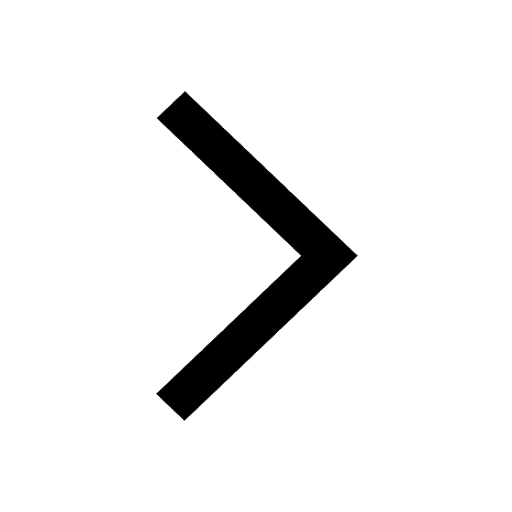
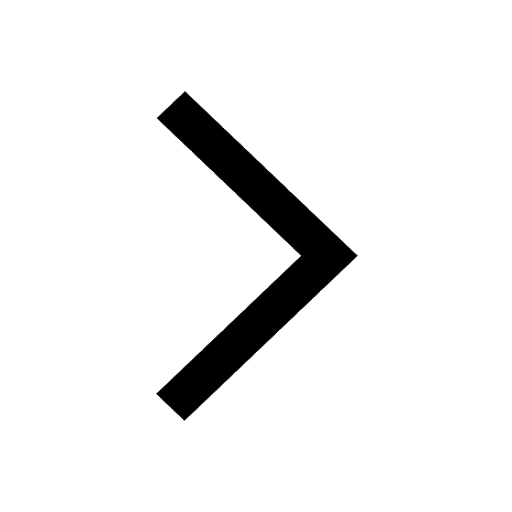
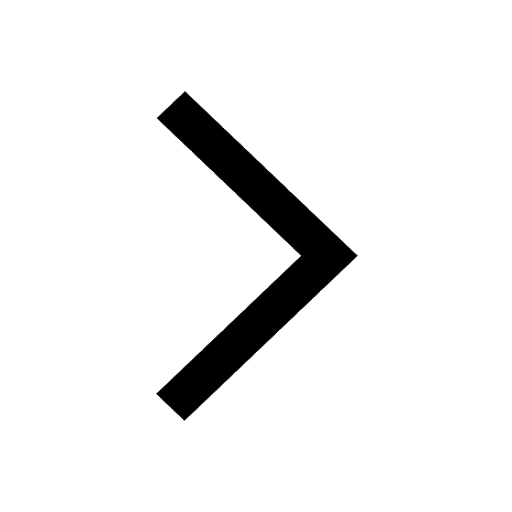
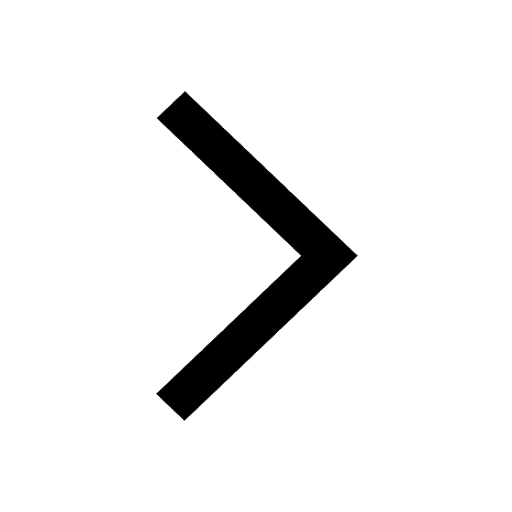
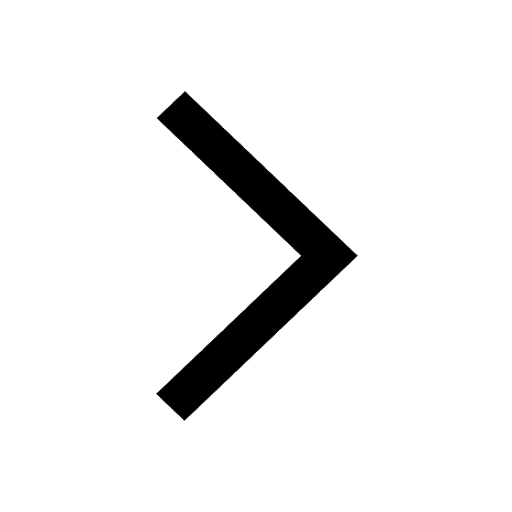
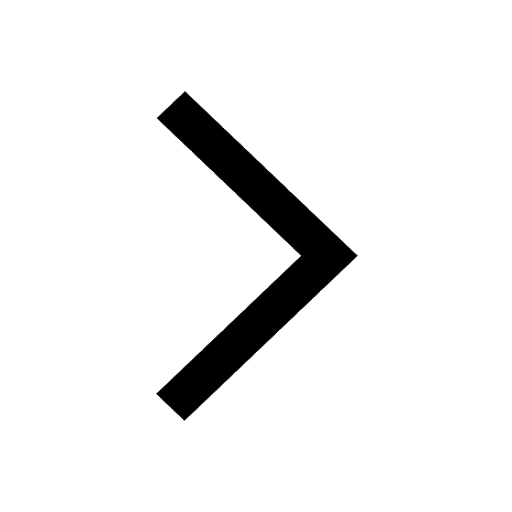
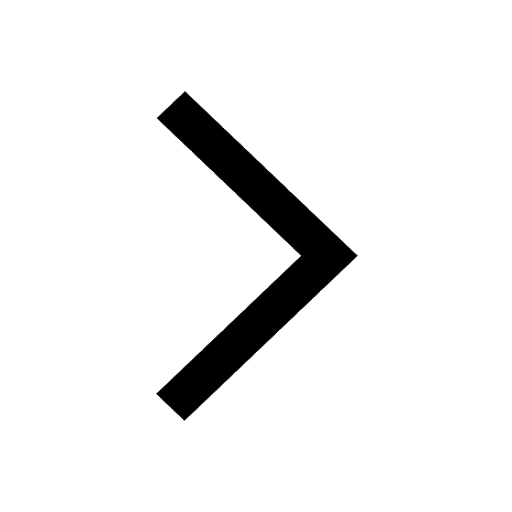
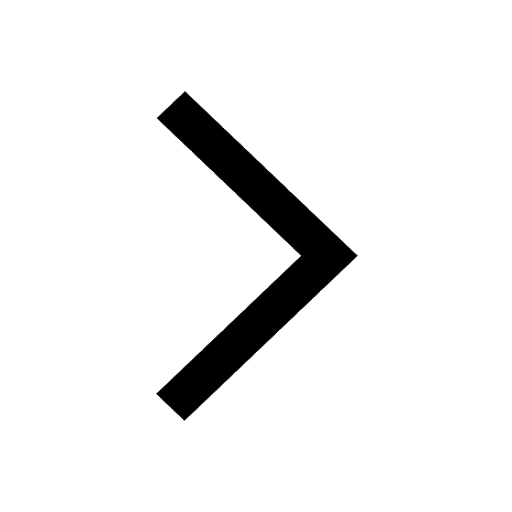
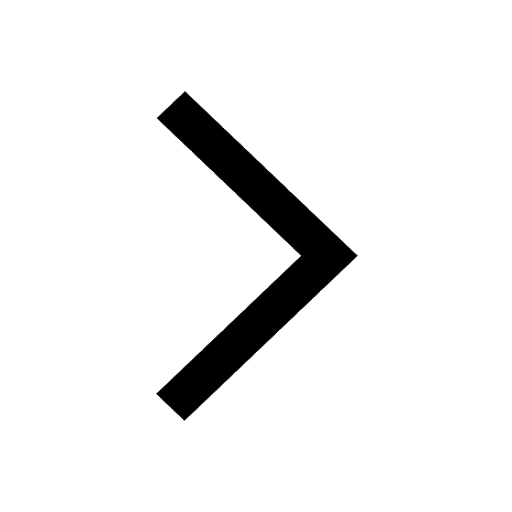
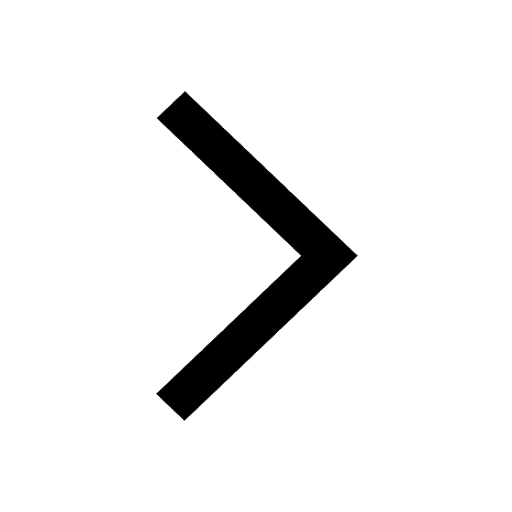
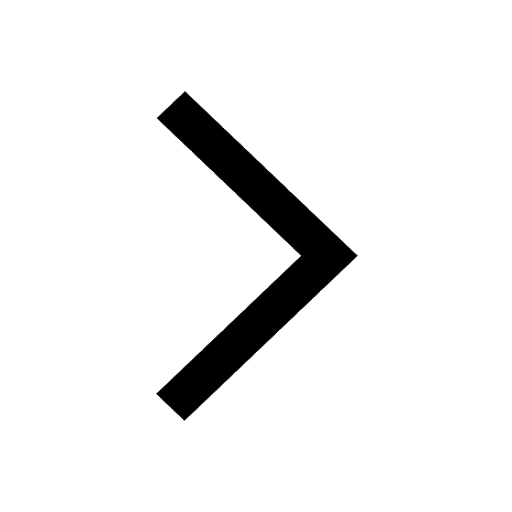
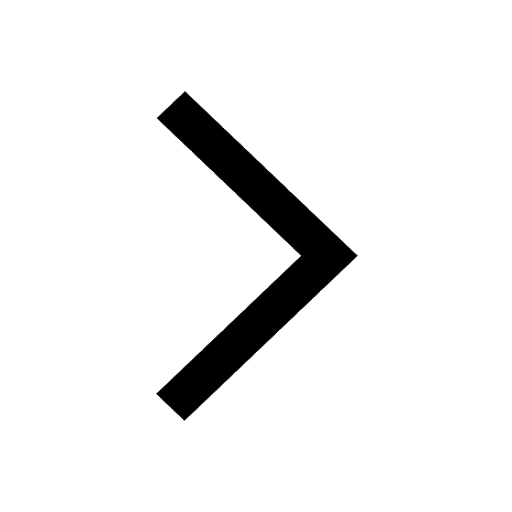
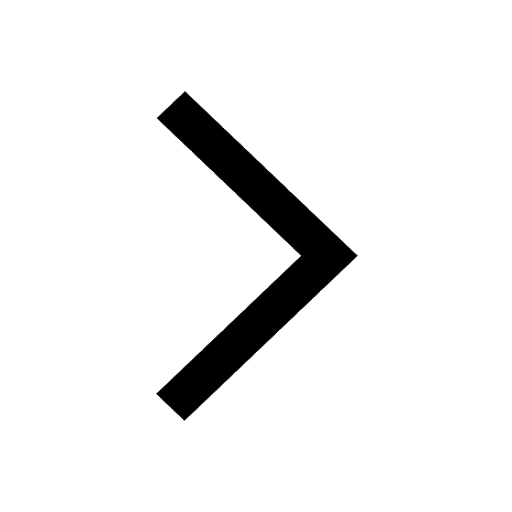
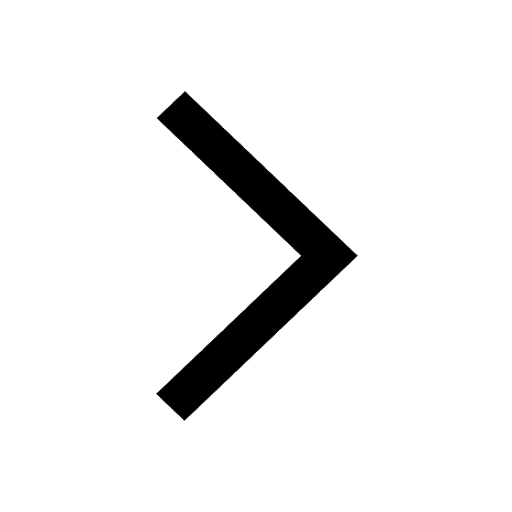
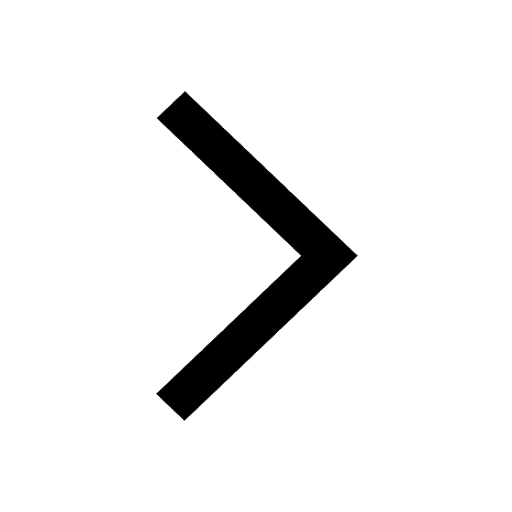
FAQs on What is Central Limit Theorem?
Question 1. Mention Some Applications of the Central Limit Theorem.
Answer: Some applications of the central limit theorem are:
The sample distribution is normal when the distribution is not known or distributed according to the central limit theorem. In this method, the assumption is that the given population will help in data analysis since the distribution is present normally.
The sample mean deviation decreases as we increase the samples that are taken from the population. This provides a clearer and more accurate estimation of the mean of the population.
The sample man can also help in creating a range of values that are more likely to include the value of population mean.
The concept of the central limit theorem is used in election polls to find the estimated value of the percentage of people that support a candidate as confidence intervals.
The central limit theorem can be used for calculating the mean family income in a country or nation.
Question 2. State the Common Uses of the Central Limit Theorem.
Answer: The central limit theorem is commonly used to analyze population parameters. It is also used to frame proper machine learning models. By using the central limit theorem, we can identify and relate if a given sample belongs to the sampling distribution of a population.