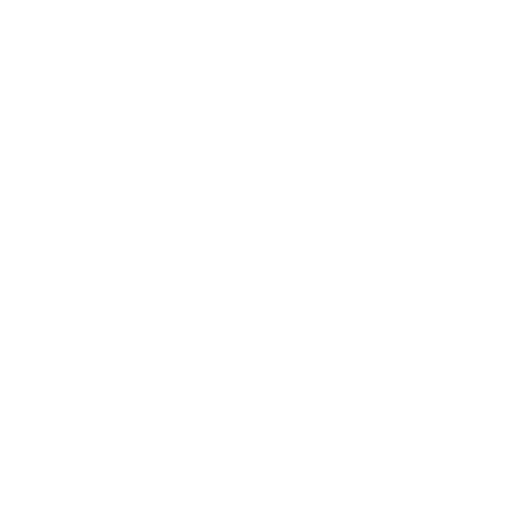
Introduction to Cumulative Distribution Function
In Mathematics, Statistics and Probability play a very important role in helping to calculate data sufficiency. The Cumulative Distribution Function is a major part of both these sub-disciplines and it is used in a number of applications. This function, also abbreviated as CDF, takes into account that a random variable valued at a real point, like X, is evaluated at x. In this case, the function holds that X will be of a lower value than x or will be valued the same as x.
We mentioned that X is a random variable. What this means is that this variable explains the probable resulting values on an unexpected phenomenon. Understanding this is fundamental to understanding the Cumulative Distribution Function.
What is a Cumulative Distribution Function?
CDF of a random variable ‘X’ is a function which can be defined as,
FX(x) = P(X ≤ x)
The right-hand side of the cumulative distribution function formula represents the probability of a random variable ‘X’ which takes the value that is less than or equal to that of the x. The semi-closed interval in which the probability of ‘X’ lies is (a.b], where a < b,
P(a < X ≤ b) = Fx(b) - Fx(a)
Note that the ≤ sign which is used here is not conventionally used at all times, but it can be useful for discrete distributions. Depending on this, the right use of binomial and Poisson’s Distribution tables are employed. Many important formulas in Mathematics are totally dependent on the equal to or the lesser than sign, such as Paul Levy’s inversion formula.
Understanding Cumulative Distributions
When random variables such as X, Y, and so on are solved, the letter that is used to subscript is the lower case of the same letter. This is done to avoid unnecessary confusion and mixups. However, the use of a subscript may not be necessary when a single random variable is being used. If the capital letter F is used for the cumulative distribution function then the lowercase letter f is used in the probability density and the probability mass functions.
The continuous random variable probability density function can be derived by differentiating the cumulative distribution function. This is shown by the Fundamental Theorem of Calculus.
\[f(x) = \frac{d}{dx} f(x) \]
The CDF of a continuous random variable ‘X’ can be written as integral of a probability density function. The ‘r’ cumulative distribution function represents the random variable that contains specified distribution.
\[F_x(x) = \int_{-\infty}^{x} f_x(t)dt \]
Understanding the Properties of CDF
In case any of the below-mentioned conditions are fulfilled, the given function can be qualified as a cumulative distribution function of the random variable:
Every CDF function is right continuous and it is non increasing. Where \[\lim\limits_{x \rightarrow -\infty } F_x(x) = 0, \lim\limits_{x \rightarrow +\infty } F_x(x) = 1 \]
If ‘X’ is a discrete random variable then its values will be x1, x2, .....etc and the probability Pi = p(xi) thus the CDF of the random variable ‘X’ is discontinuous at the points of xi. FX(x) = P(X ≤ x) = Σxi ≤ x P(X = xi) = Σxi ≤ x p(xi).
If the CDF of a real-valued function is said to be continuous, then ‘X’ is called a continuous random variable Fx(b) - Fx(a) = P(a < X ≤ b) = ∫ab fX(x) dx.
The function fx = derivative of Fx is the probability density function of X.
Derived Functions
Complementary Cumulative Distribution Function: It is also known as tail distribution or exceedance, it is defined as, Fx(x)=P(X>x)=1−FX(x)
Folded Cumulative Distribution: When the cumulative distributive function is plotted, and the plot resembles an ‘S’ shape it is known as FCD or mountain plot.
Inverse Distribution Function: The inverse distribution function or the quantile function can be defined when the CDF is increasing and continuous. F−1(p),pϵ[0,1]F−1(p),pϵ[0,1]F^{-1} (p), p \epsilon [0,1] such that F(x) = p.
Empirical Distribution Function: The estimation of cumulative distributive function that has points generated on a sample is called empirical distribution function.
Solved Example 1.
1. What is the cumulative distribution function formula?
Given the CDF F(x) for the discrete random variable X,
Find: (a) P(X = 3) (b) P(X > 2)
Solution:
CDF of a random variable ‘X’ is a function which can be defined as,
FX(x) = P(X ≤ x)(a) P(X = 3)
To obtain the CDF of the given distribution, here we have to solve till the value is less than or equal to three. From the table, we can obtain the value
F(3) = P(X 3) = P(X = 1) + P(X = 2) + P(X = 3)
From the table, we can get the value of F(3) directly, which is equal to 0.67.
(b) P(X > 2)
P(X > 2) = 1 - P(X ≤ 2)
P(X > 2) = 1 - F(2)
P(X > 2) = 1 - 0.32P
(X > 2) = 0.87
2. What is the CDF of normal distribution in r?
Given the probability distribution for a random variable x,
find (a) P(x ≤ 4.5) (b) P(x > 4.5)
Solution:
The CDF of the normal distribution can be denoted by " φ " the probability of a random variable that has a related error function.
(a) P(x ≤ 4.5) = F(4.5) = 0.8
(b) P(x > 4.5) = 1 - P(x ≤ 4.5)
(c) P(x > 4.5) = 1 - 0.8
(d) P(x > 4.5) = 0.2
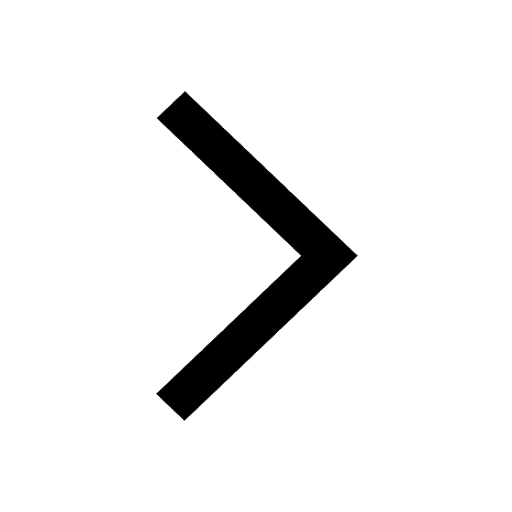
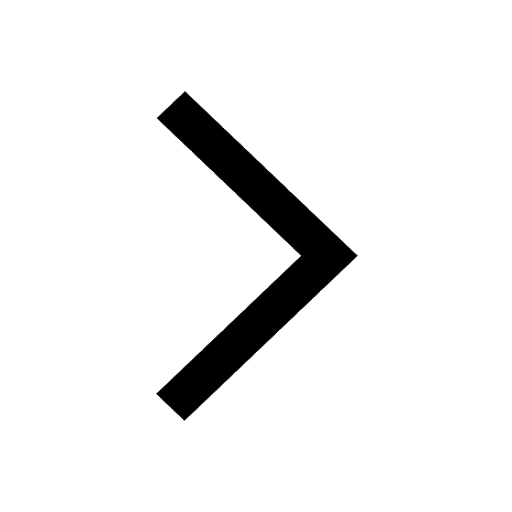
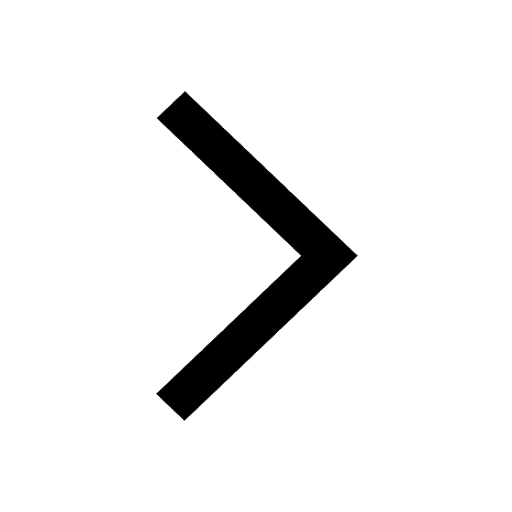
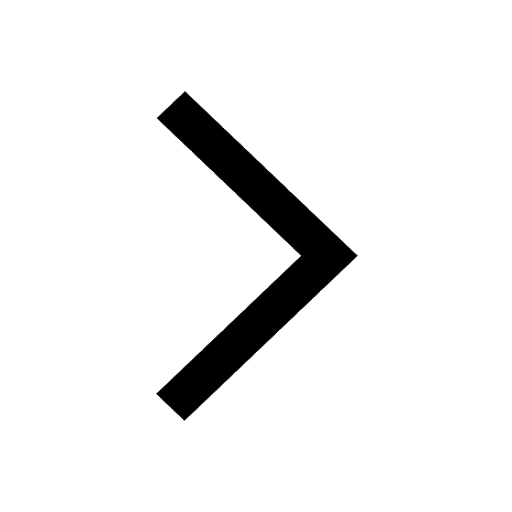
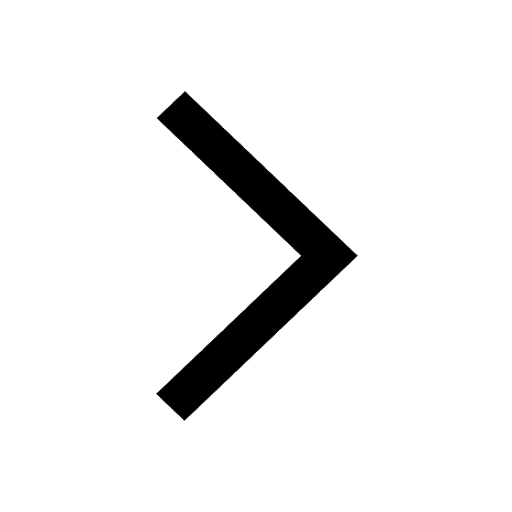
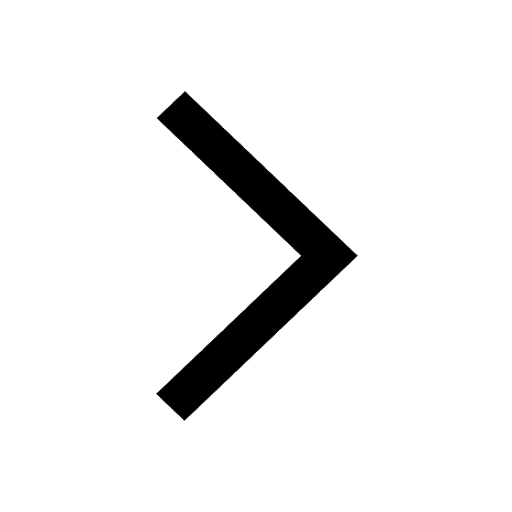
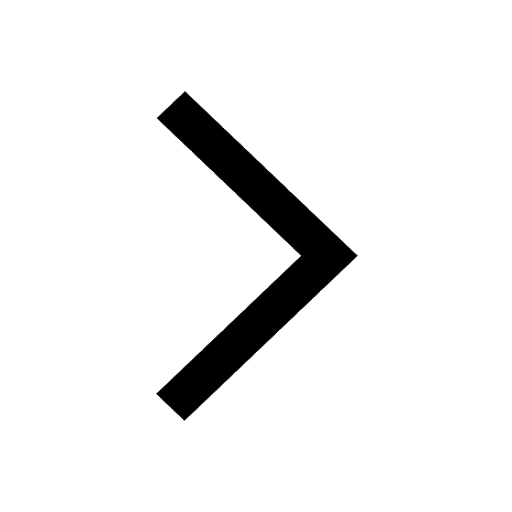
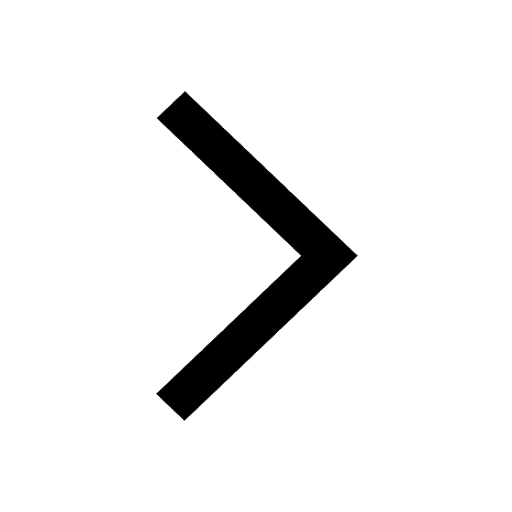
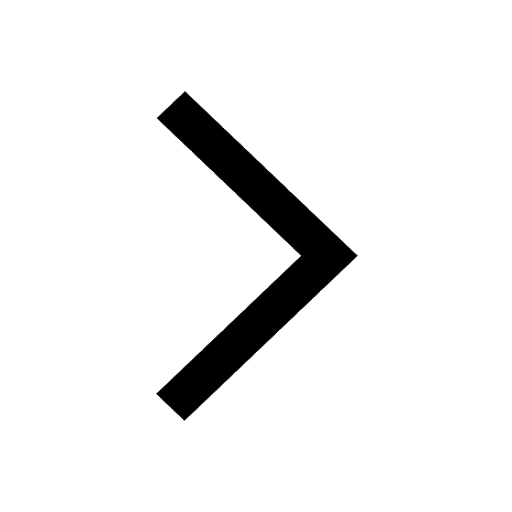
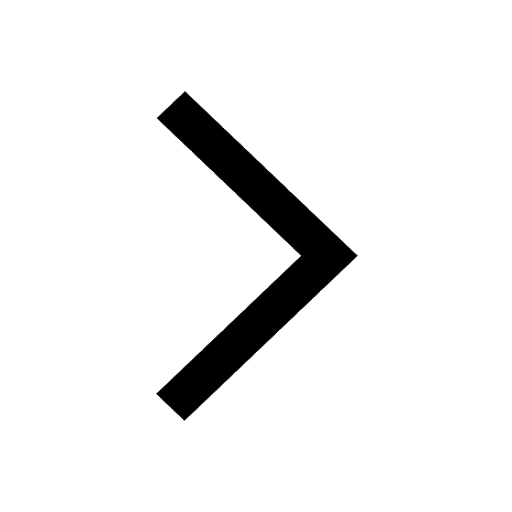
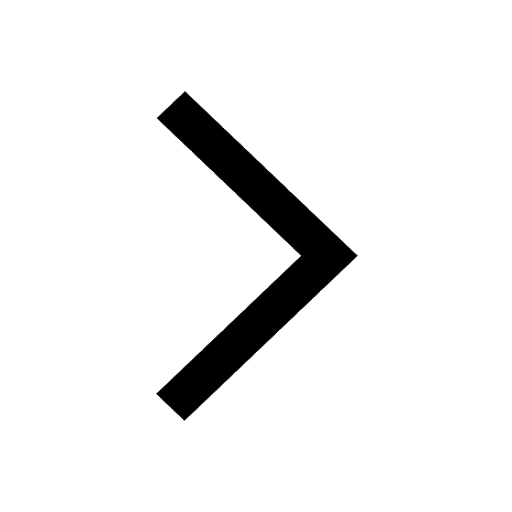
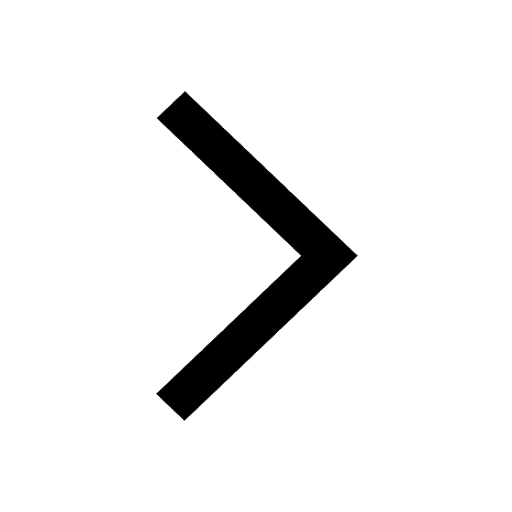
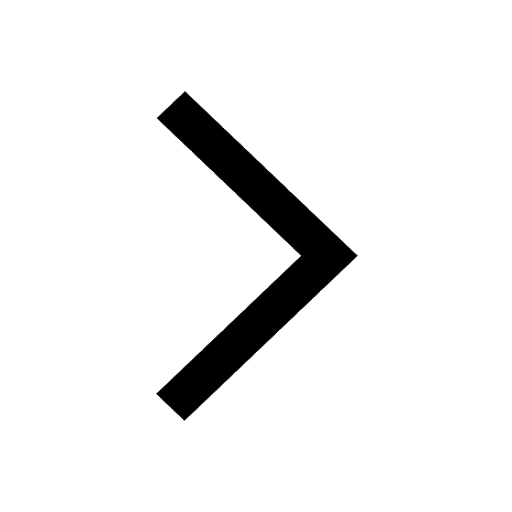
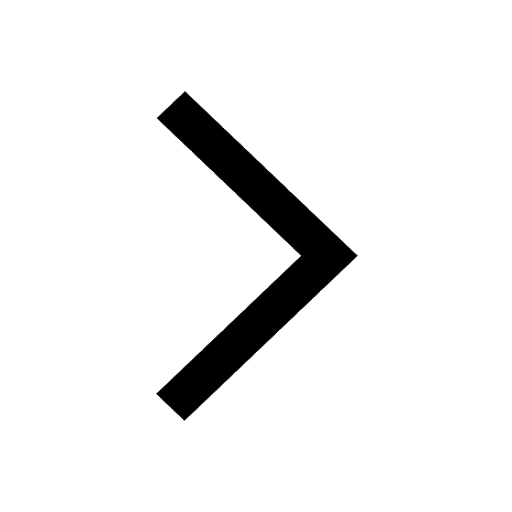
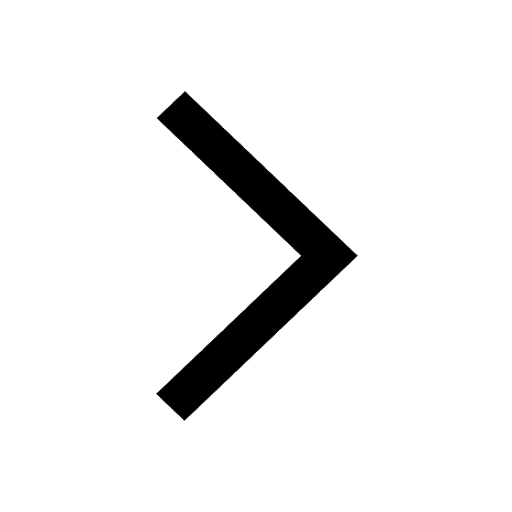
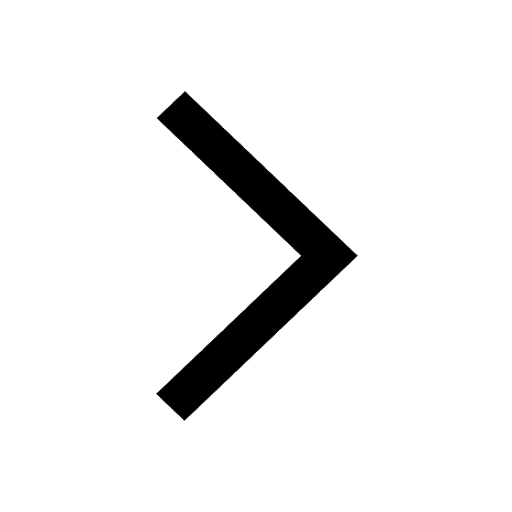
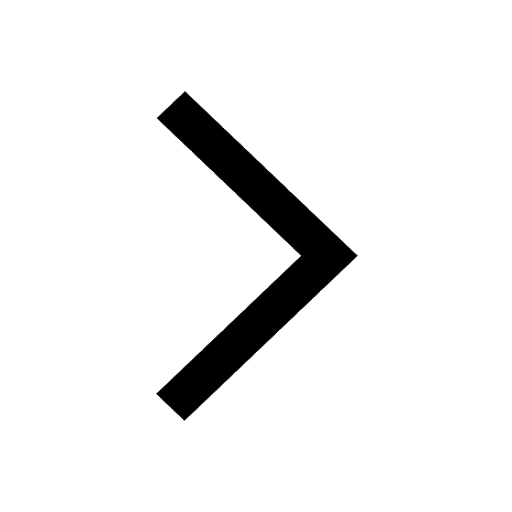
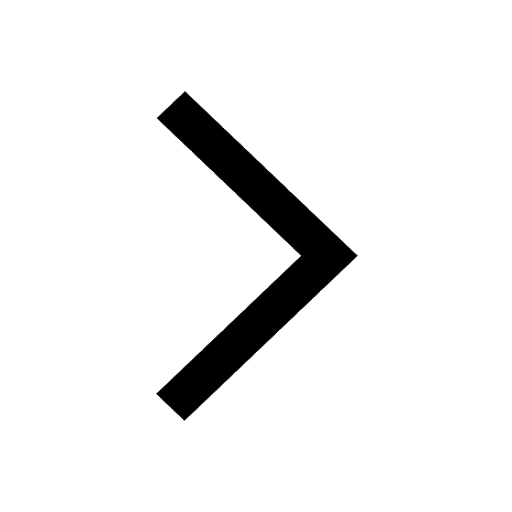
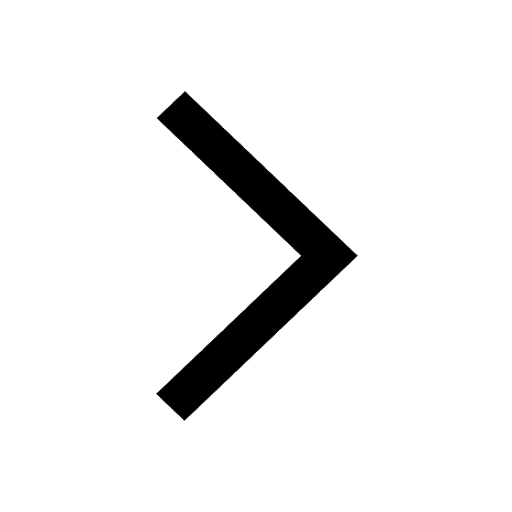
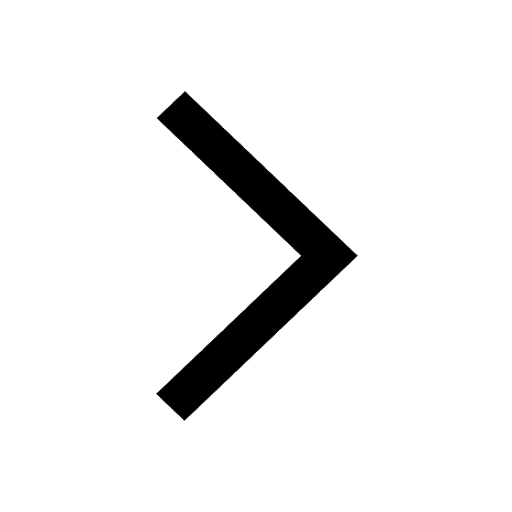
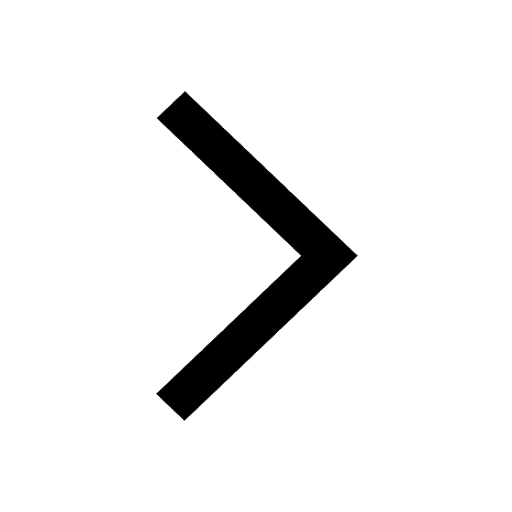
FAQs on Cumulative Distribution Function
1. How is cumulative distribution function used in Statistical applications?
Statistical analysis uses the applications of the cumulative distribution function, this is done in two ways. The value that occurs in less frequency is used in cumulative frequency analysis. Two kinds of statistical hypotheses and their tested results can give evidence regarding sample data analysis that was derived from a given distribution table. A test known as the Kolmogorov-Smirnov test is employed to check whether the empirical data differs in any way from the ideal distribution. In case the data distribution is cyclic in nature, Kiper’s test approach is employed.
2. Is it possible for the cumulative distribution function to be more than 1?
A commonly held hypothesis is used in Statistics and Probability, which states that probability can never be greater than the value of 1. This also applies to the cumulative distribution function. This implies that the value of CF at any point can also not be greater than 1. The integral value of the PDF needs to be less than, or equal to the value of 1 stretched over different intervals. CDF at negative infinity is equal to zero, at infinity its value is equal to 1 and it can never decrease.
3. What is PDF and how does it differ from CDF?
Probability density function, also referred to as PDF is used in close collaboration with CDF. In the case of a continuous function, the PDF assumes that the variate is valued at x. In the case of continuous distributions at any single point, the probability is zero. This is expressed in terms of the integration noticed between the two points. As opposed to this, CDF is firstly valued really. The random variable X has the same value as x or is lesser than it when it is evaluated at x. Probability distributions of random variables in a table can be expressed using this.
4. As a function, why does CDF increase monotonically?
As long as the function in question describes a cumulative distribution, it is compulsorily bounded below by zero and bounded by 1 above. This indicates that having a probability that goes above 1 or goes less than zero would be a very unusual phenomenon and difficult to comprehend. As a result of this, it cannot be placed at these values. These cumulative distribution functions make it increase monotonically. This indicates that it describes a nonincreasing function at all stages of the function.
5. Can CDF be of a different nature?
Probability Density Function or PDF is a derivative of the cumulative derivation function or the CDF. The existence of the PDF is inherently dependent on the nature of the CDF that it is derived from. In case only true functions are considered, and functions such as Dirac deltas are disregarded, then cumulative distribution function is essentially differential in nature. This is the principle on which PDF itself is based, and this is the only essential requirement or condition that it requires.
6. What is the Cumulative Distribution Function?
CDF of a random variable ‘X’ is defined as a function given by, FX(x) = P(X ≤ x)where the x ∈ R. This indicates that CDF is applicable for all the x ∈ R. It helps to calculate the probability of a random variable where the population is taken less than or equal to a particular value.
7. Write Down the Properties of CDF.
The properties of CDF are as follows,
Every CDF function is right continuous and it is non increasing. Where \[\lim\limits_{x \rightarrow -\infty } F_x(x) = 0, \lim\limits_{x \rightarrow +\infty } F_x(x) = 1 \].
If ‘X’ is a discrete random variable then its values will be x1, x2, .....etc and the probability Pi=p(xi) thus the CDF of the random variable ‘X’ is discontinuous at the points of xi. FX(x) = P(X ≤ x) = Σxi ≤ x P(X = xi) = Σxi ≤ x p(xi).
If the CDF of a real-valued function is said to be continuous, then ‘X’ is called a continuous random variable FX(b) - FX(a) = P(a < X ≤ b) = ∫ab fX(x) dx.