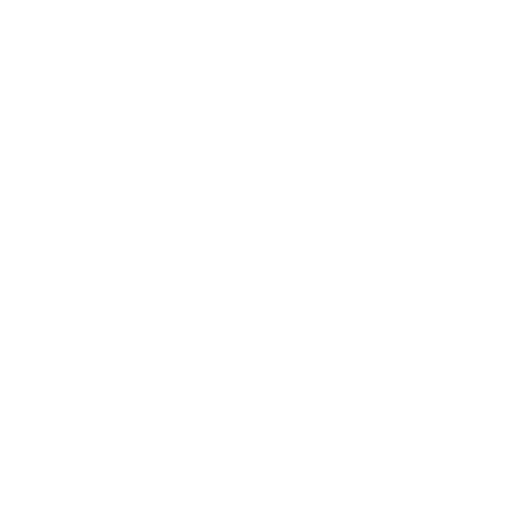
Bivariate Analysis Definition
Bivariate data help you in studying two variables. For example, you are studying a group of college students. To find out their average SAT score and their age, you have two pieces of the puzzle to find (SAT score and age). Both SAT and age are variables. Now suppose you need to find a relation between the weights and heights of college students, then also you have bivariate data. Bi means two, variate means variables. Analysis of the changes in the two variables is called bivariate analysis. Out of the two variables, one is dependent and the other is independent.
Data in statistics are sometimes classified according to how many variables are in a particular study. For example, “height” and “weight” might be two different variables. Depending on the number of variables being looked at, the data might be univariate, or it might be bivariate.
When you conduct a study that looks at a single variable, that study involves univariate data. Bivariate data could also be two sets of items that are dependent on each other. For example
Sale of Ice cream compared to the temperature of that day.
Traffic accidents along with the weather on a particular day.
Bivariate data has many practical uses in real life. For example, it is pretty helpful to be able to predict when a natural event might occur. One tool in the statistician’s toolbox is bivariate data analysis. Sometimes, something as simple as plotting one variable against another on a Cartesian plane can give you a clear picture of what the data is trying to tell you. For example, the scatterplot below shows the relationship between the time between eruptions at Old Faithful vs. the duration of the eruption.
What is Bivariate Analysis?
Bivariate analysis is an analysis of two variables to determine the relationships between them. They are often reported in quality of life research. It is one of the simplest forms of quantitative (statistical) analysis. It involves the analysis of two variables (it is often denoted as X, Y), for the purpose of determining the empirical relationship between them.
Bivariate analysis is extremely helpful in testing simple hypotheses of association. It is very helpful in determining to what extent it becomes easier to know and predicts a value for one variable (possibly a dependent variable) if the value of the other variable (possibly the independent variable) is known (see also correlation and simple linear regression). There can be a contrast between bivariate analysis and univariate analysis in which only one variable is analyzed. Both univariate analysis and bivariate analysis can be descriptive or inferential. We can say, it is the analysis of the relationship between the two variables. Bivariate analysis is a simple (two-variable) and special case of multivariate analysis (where simultaneously multiple relations between multiple variables are examined).
Bivariate analysis can be defined as the analysis of bivariate data. It is one of the simplest forms of statistical analysis, which is used to find out if there is a relationship between two sets of values. Usually, it involves the variables X and Y.
The univariate analysis involves an analysis of one (“uni”) variable.
The bivariate analysis involves the analysis of exactly two variables.
The multivariate analysis involves the analysis of more than two variables.
The results we get from the bivariate analysis can be stored in a two-column data table. For example, you might be eager to find out the relationship between caloric intake and weight (of course, the two are related very strongly). Caloric intake will be your independent variable, X, and weight will be your dependent variable, Y.
Bivariate analysis and two sample data analyses are not the same. With two sample data analysis (like a two-sample is a test in Excel), X and Y are not directly related and there will also be a different number of data values in each sample. With bivariate analysis, there is a Y value for each X. For example, suppose you had a caloric intake of 3,000 calories per day and a weight of 300lbs. You will have to write that with the x-variable followed by the y-variable: (3000,300).
Here are Two sample data analysis
Sample 1: 100,45,88,99
Sample 2: 44,33,101
Bivariate analysis
(X,Y)=(100,56),(23,84),(398,63),(56,42)
Types of Bivariate Analysis
Some of the common types of bivariate analysis include:
Scatter Plots: Scatterplot provides you with a visual idea of the pattern that your variables follow.
Graph-A simple scatterplot
Regression Analysis: Regression analysis is a catch-all term for a wide variety of tools that can be used to determine how your data points might be related. The points in the image above seem like they could follow an exponential curve (as opposed to a straight line). Regression analysis not only provides you with an equation for that curve or line but also gives you the correlation coefficient.
Correlation Coefficients: Calculation of values for correlation coefficients are performed using a computer, although here, you can find the steps to find the correlation coefficient by hand. This coefficient acknowledges you if the variables are related. Basically, by ‘0’ means they aren't correlated (i.e. related in some way), while a ‘1’ (either positive or negative) means that the variables are perfectly correlated (i.e. they are perfectly in sync with each other).
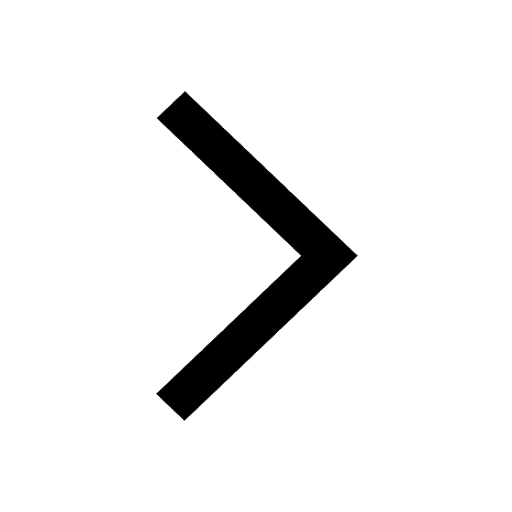
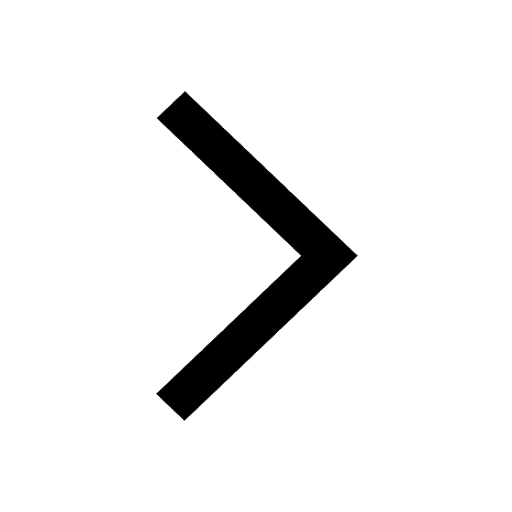
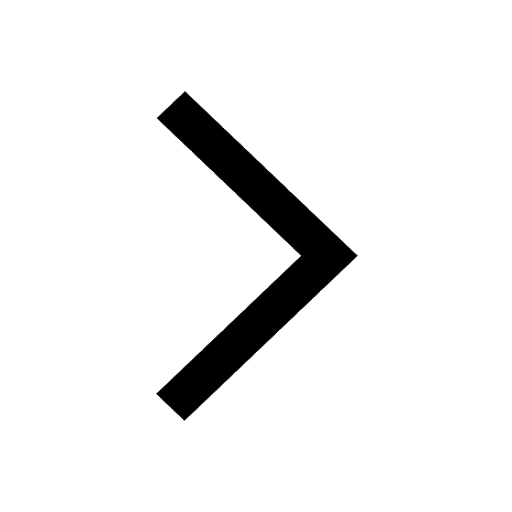
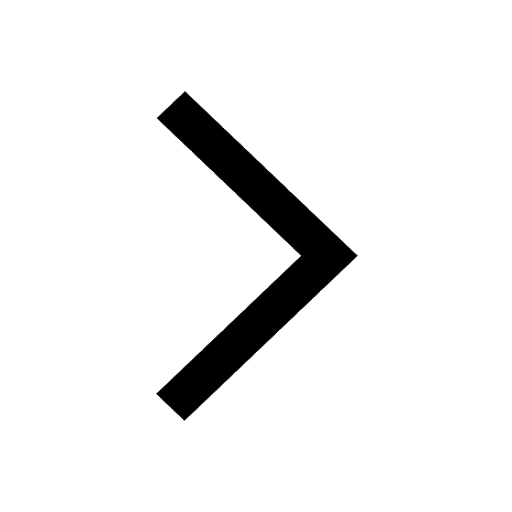
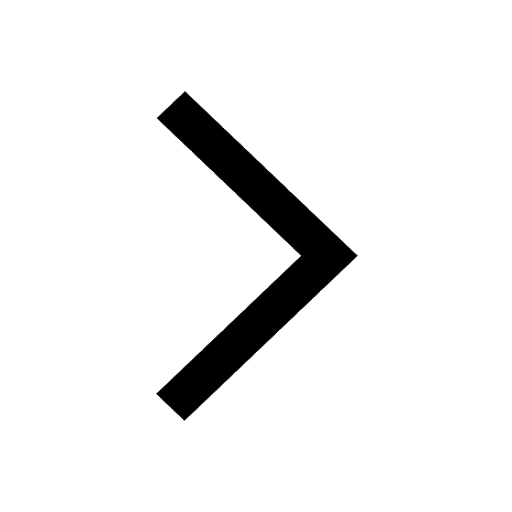
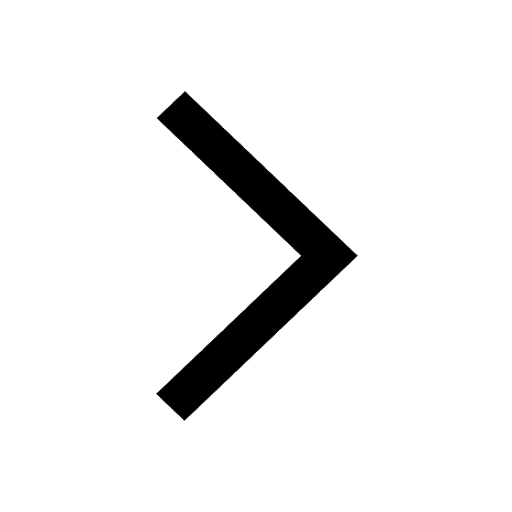
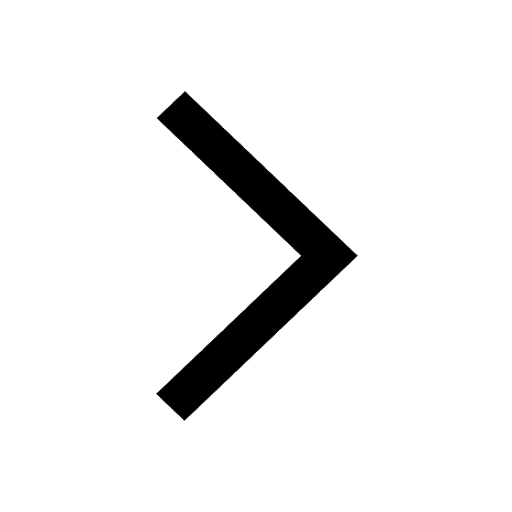
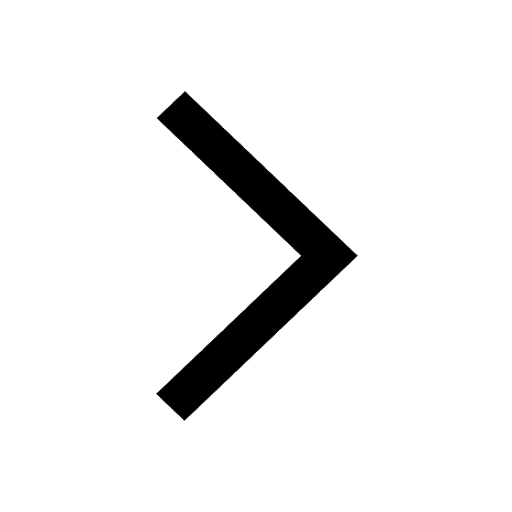
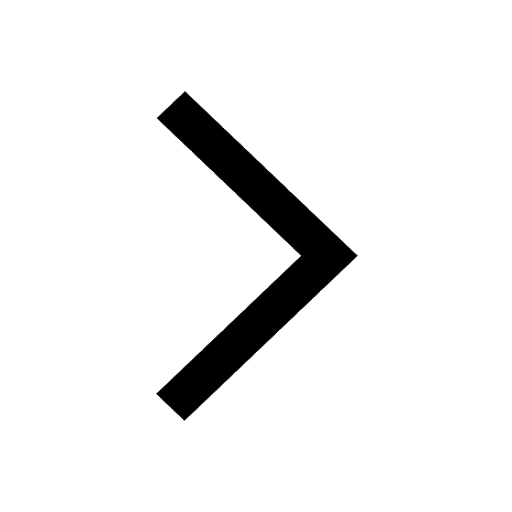
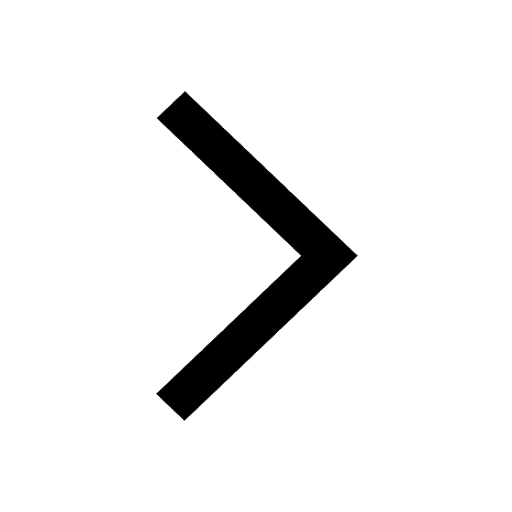
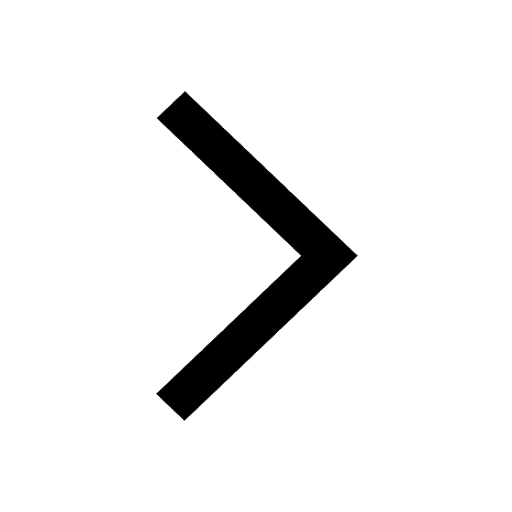
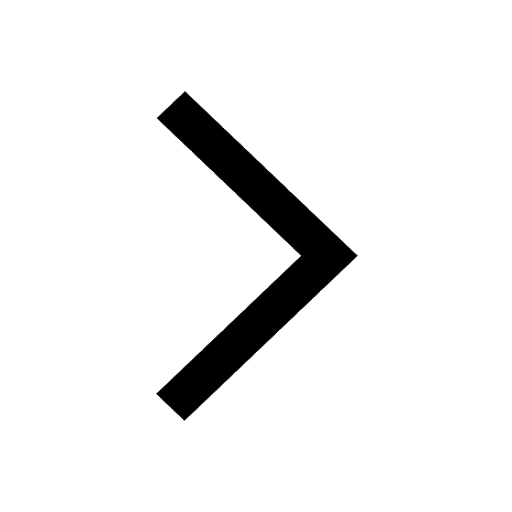
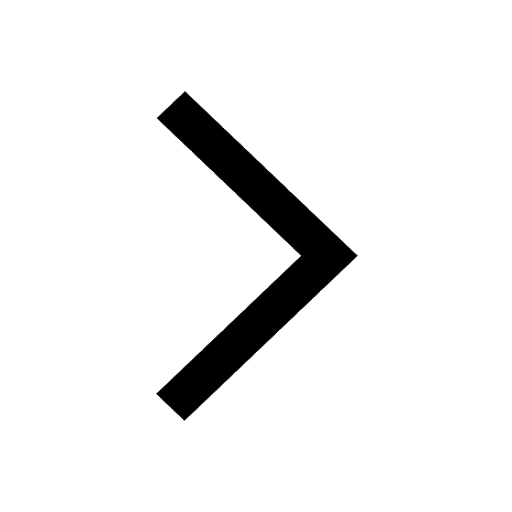
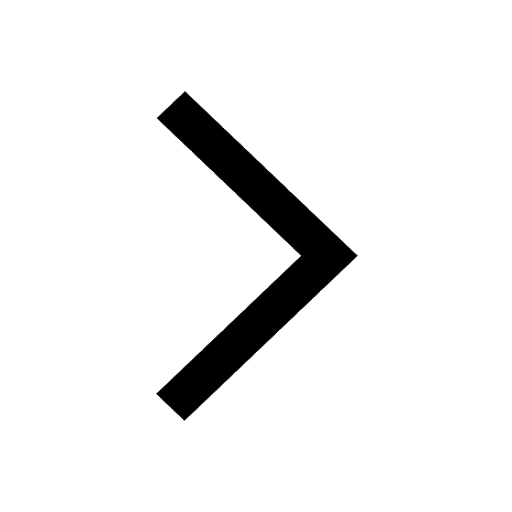
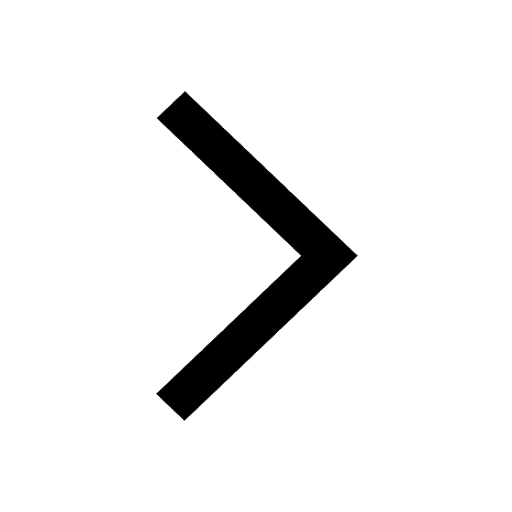
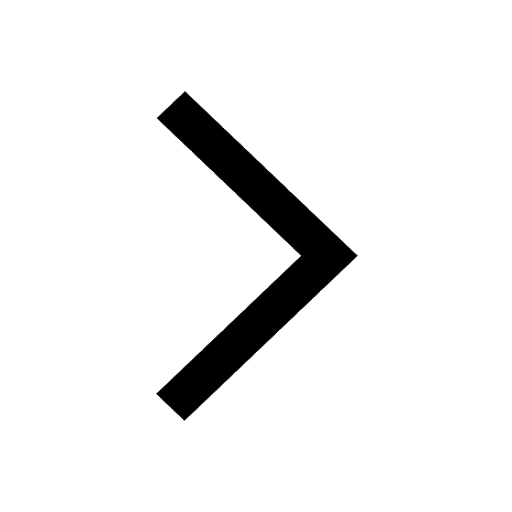
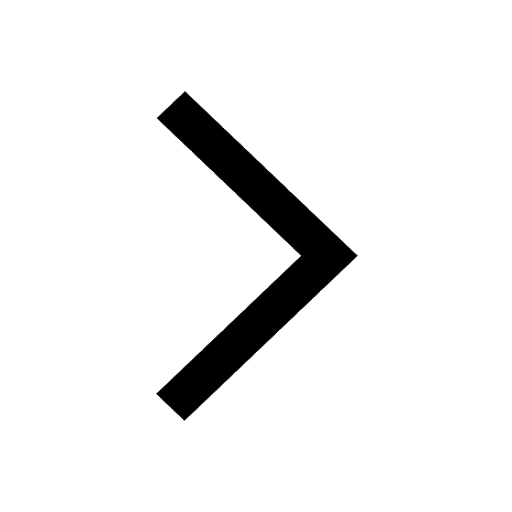
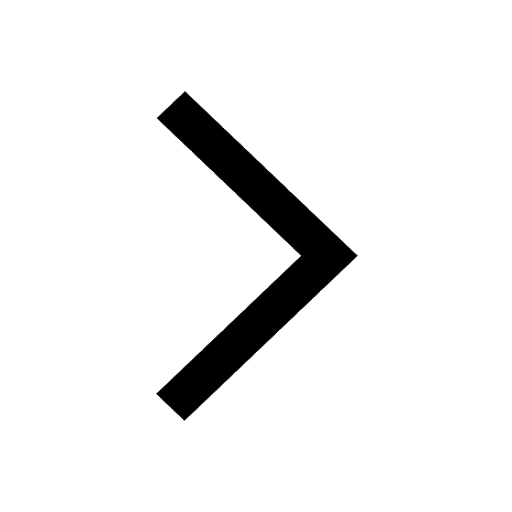
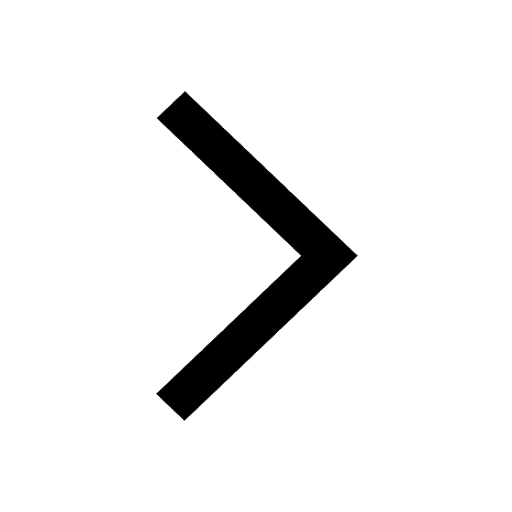
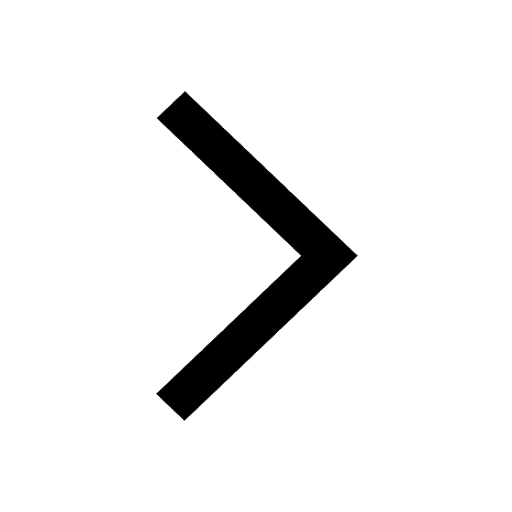
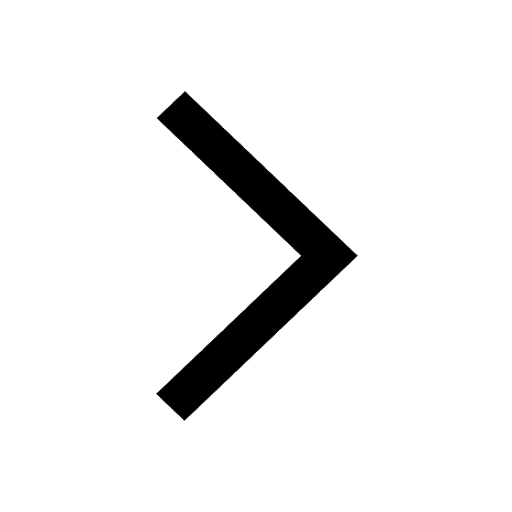
FAQs on Bivariate Analysis
1. What is bivariate analysis? List its types.
The analysis of two specific variables to determine the empirical relationship present between them is referred to as bivariate analysis and it is considered to be one of the simplest forms of quantitative analysis. It is of utmost help when it comes to testing simple hypotheses of association and determining the extent to which it becomes easier to predict the value of one particular variable, given the value of the other variable is already known.
There are three main types of bivariate analysis. They are as follows:
Scatter Plots: It makes use of dots to represent the values for two different numeric variables. In other words, it provides us with a visual idea of what pattern the variables are following.
Regression Analysis: This involves a wide range of tools that can be utilized to determine just how the data points might be related. It tends to provide us with an equation for the curve/line along with giving us the correlation coefficient.
Correlation Coefficients: This shows how one particular variable moves about with relation to another.
2. What are bivariate variables?
In certain cases of bivariate data, one variable is said to determine or influence the other one. These two types of variables are distinguished as independent and dependent variables. The former refers to a situation wherein neither of the variables is considered to be dependent on each other. In this case, regression isn’t appropriate but correlation to a certain extent might just be. In the situation of the latter though, if the dependent variable is a categorical one then regression could be used. And if both of the variables are ordinal, then a rank correlation coefficient could be computed but if it’s just the dependent variable that is the ordinal one, then ordered logit or ordered probit could be utilized.
A certain type of causality, called Granger causality, tends to appear in a situation wherein both the variables are time series. You can learn more about Bivariate Analysis and variables in Vedantu. You will get different study materials on the Vedantu website and app which will help you to understand this topic better.
3. What is univariate analysis? What is the difference between univariate and bivariate data?
The type of analysis that is only carried out on a single variable is referred to as univariate analysis. And since it only deals with one variable, there are neither any causes nor any relationships. It is the simplest form of statistical analysis and can be either descriptive or inferential, based on the situation. The central purpose of the univariate analysis is to describe the data and look for patterns that tend to exist within it.
The difference between univariate and bivariate data is as follows:
Univariate data | Bivariate data |
It only tends to involve one variable. | It involves two variables. |
It does not deal with either causes or relationships. | It tends to deal with causes and relationships. |
For example, how many of the students present in the lecture are females? | For example, find out the relationship that exists between teenagers reading and their scores in English. |
4. What is multivariate analysis?
The data that tends to involve more than one type of observation or measurement, is analyzed with the help of a specific statistical procedure known as multivariate analysis. It is more of a subdivision of statistics that encompasses the simultaneous observation as well as analysis of more than just a single variable. Its practical application may involve some kinds of univariate and multivariate analyses to further comprehend the relationship between variables and their relevance to the said problem that is being studied.
5. Which statistical tools tend to fall under bivariate analysis?
Bivariate statistics is a kind of inferential statistic that tends to deal with the relationship there is between two particular variables. In simpler words, the examination of just how one variable is compared with another, or how one particular variable ends up influencing the other, is known as bivariate statistics.
Communication research in correlation is easily one of the most common types of bivariate statistics to be used. The measure of the strength of an association between two particular variables, correlation. This type of bivariate statistical analysis tends to involve the creation of a scatter graph along with the calculation of Pearson’s correlation coefficient. And bivariate data is used when bivariate statistics examines how a relationship between two variables tends to work.
6. What is the importance of doing a Univariable analysis of variables before doing Multivariate analysis given the fact that outcomes of Multivariate analyses are more accurate?
There is no need to do both. In fact, for a given research question, only one of the two is appropriate.
Analyzing the data in different ways is quite understandable, especially when doing “hypothesis-generating” research without having a clear hypothesis in mind. However, there lies a risk of p-hacking: if you analyze the data in 10 different ways.
A multivariable analysis is never more accurate than a simple t-test. In fact, the more simple the test would be more powerful. The main reason multivariable analysis is done is that you get to identify causal effects.