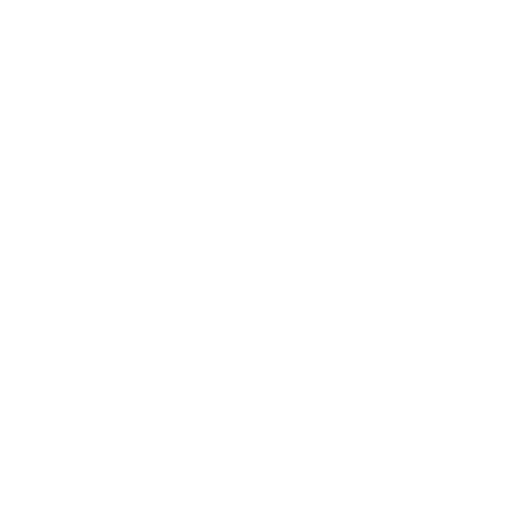
Let’s Unwind Coefficient of Determination!!
The coefficient of determination method in statistical analysis is used to forecast and describe the future outcomes of a model. This method is also referred to as R squared, which acts as a guideline to measure the accuracy of the model.
The coefficient of determination or R squared method is the variance of the dependent variable in the proportion that is predicted through an independent variable. It denotes the level of variation in the provided data.
The coefficient of determination is nothing but the square of the correlation(r), hence it ranges from 0 to 1.
As per linear regression, the coefficient of determination is the same as the square of the correlation between the x and y variables.
If R2 is 0, the independent variable cannot predict the dependent variable.
If R2 is 1, the independent variable without any error cannot predict the dependent variable.
If R2 ranges between 0 and 1, then it denotes the extent that can be predicted by the dependent variable. If R2 is 0.10, then the x variable has predicted 10 percent of the variance in the y variable. If R2 of 0.20, then the x variable has predicted 10 percent of the variance in the y variable, and so on.
Whether the model would fit the given data set or not, can be determined using the R2 value. For any percentage of the variation, the good fit would be different.
Coefficient of Determination Formula
The formula to find the coefficient of determination is classified into two types- The correlation coefficient and the sum of squares.
Formula 1:
\[ r = \frac{n(\sum xy) - (\sum x) (\sum y)}{\sqrt{[n\sum x^{2} - (\sum x)^{2}] [n\sum y^{2} - (\sum y)^{2}]}}\]
Where,
n is the total number of observations.
Σx is the total of the first variable value.
Σy is the total of the second variable value.
Σxy is the sum of the product of the first & the second value.
Σx2 is the sum of the squares of the first value.
Σy2 is the sum of the squares of the second value.
Thus, the coefficient of determination = (correlation coefficient)2 = r2.
Formula 2:
\[R^{2} = 1 - \frac{RSS}{TSS}\]
Where,
R2 is the coefficient of determination.
RSS is the residual sum of squares.
TSS is the total sum of squares.
Properties of Coefficient of Determination
It helps to determine the variation in the ratio of how a variable is predicted from the other.
It helps to determine how to make predictions from the given data by using this measurement.
It helps to determine the explained variation / total variation
It helps to understand the strength of the association (linear) between the variables.
If the value of R2 is close to 1, The values of y will be close to the regression line, and similarly, if it is nearing 0, the values move away from the regression line.
It helps to determine the strength of association between different variables.
Steps to Find the Coefficient of Determination
Find r, Correlation Coefficient
Square ‘r’.
Change the value to a percentage.
Conclusion:
This article focuses on the Coefficient of Determination and its application. You need to be thorough with the topic to be able to apply it in practice. Mathematics requires you to repeatedly do sums to perfect the concepts.
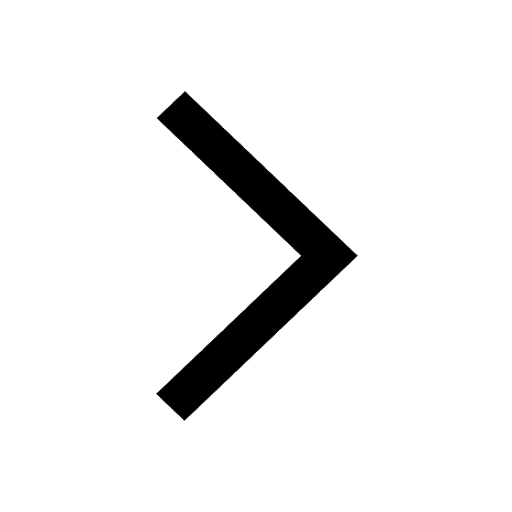
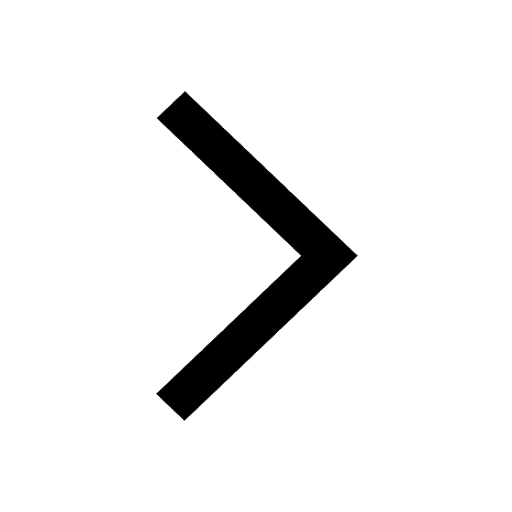
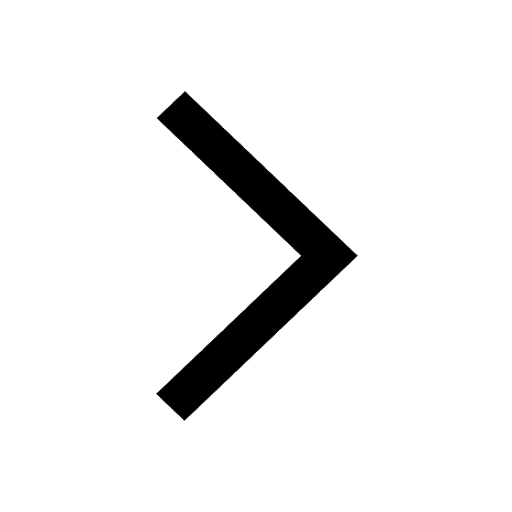
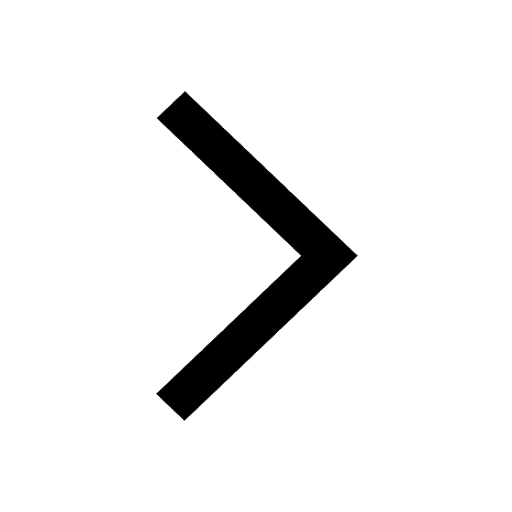
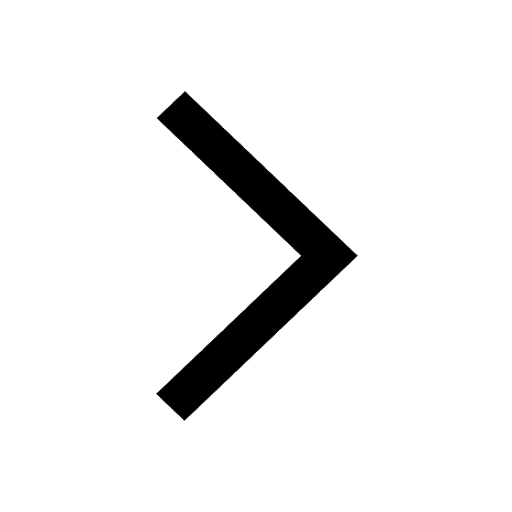
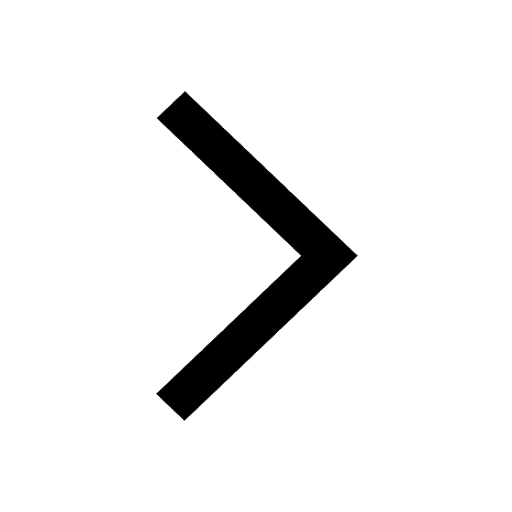
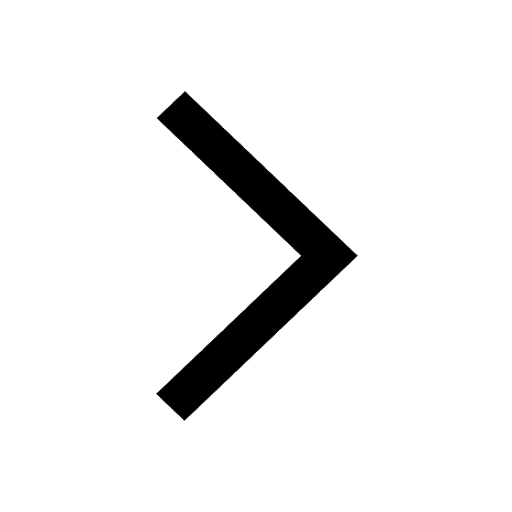
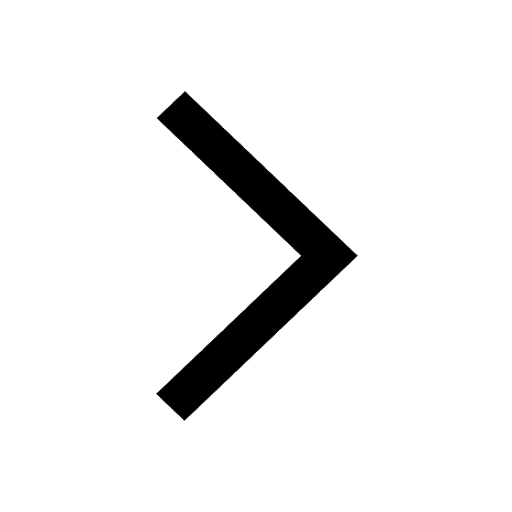
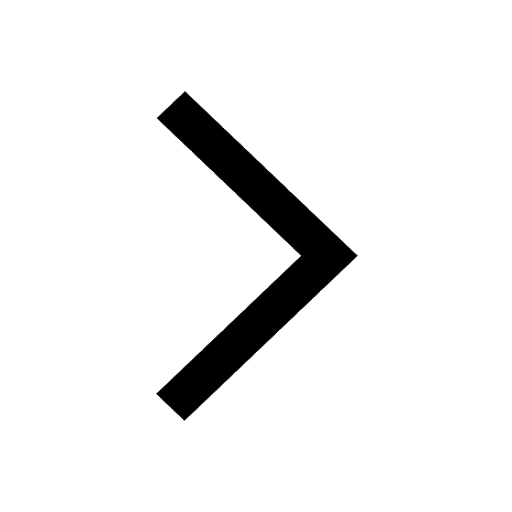
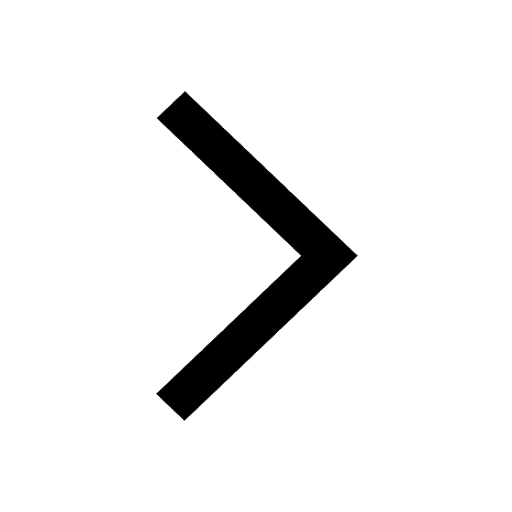
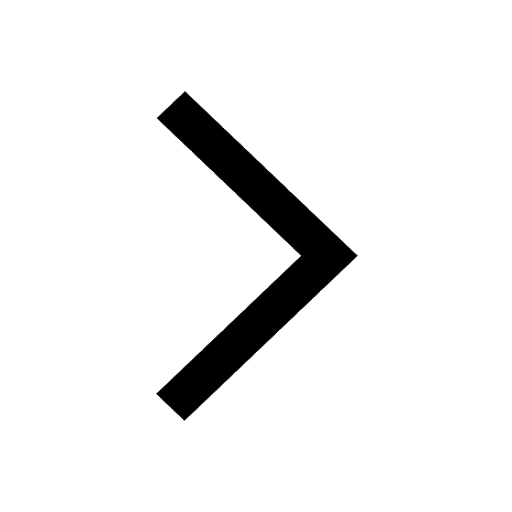
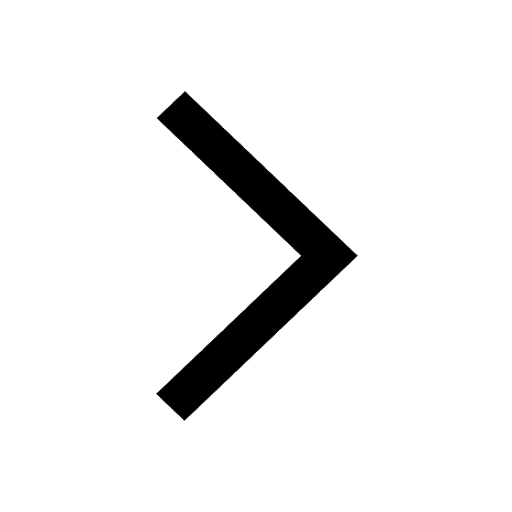
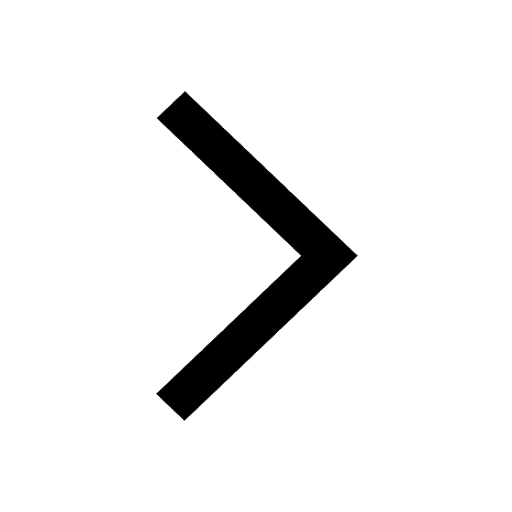
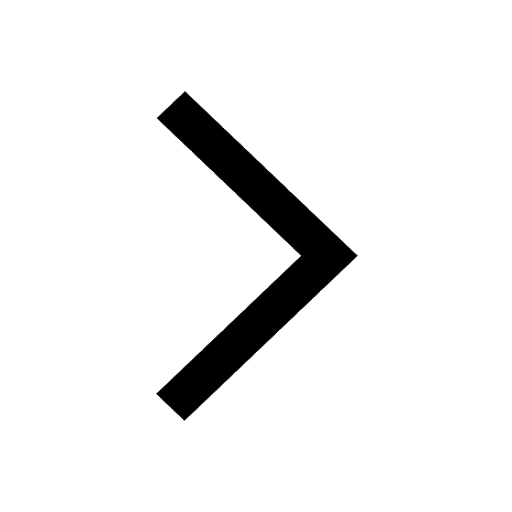
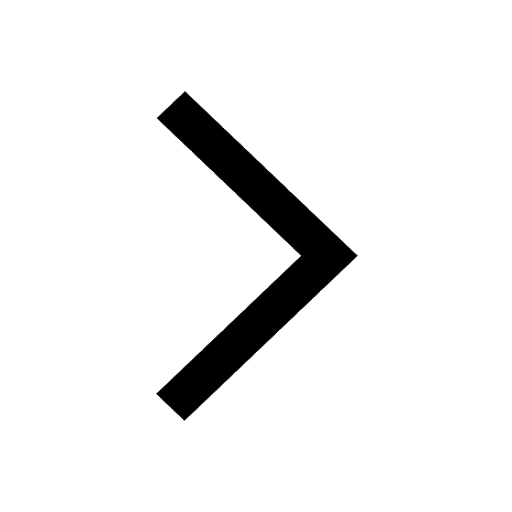
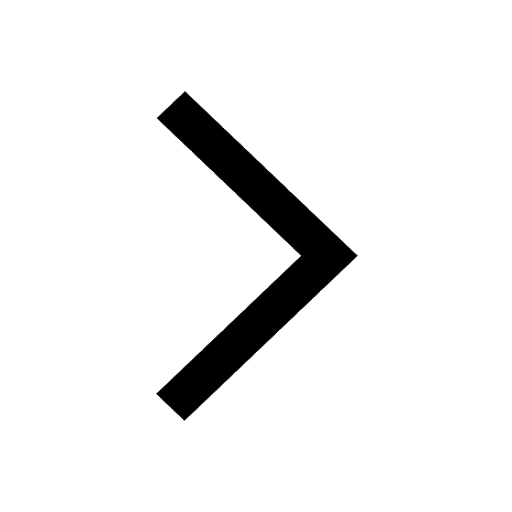
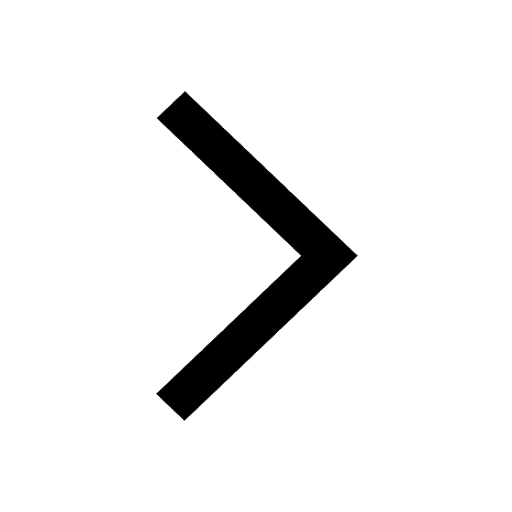
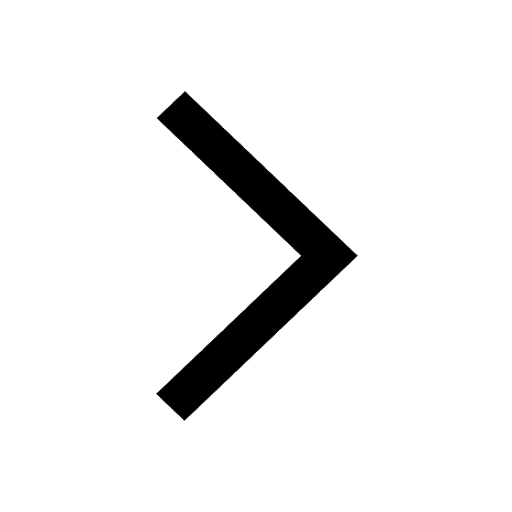
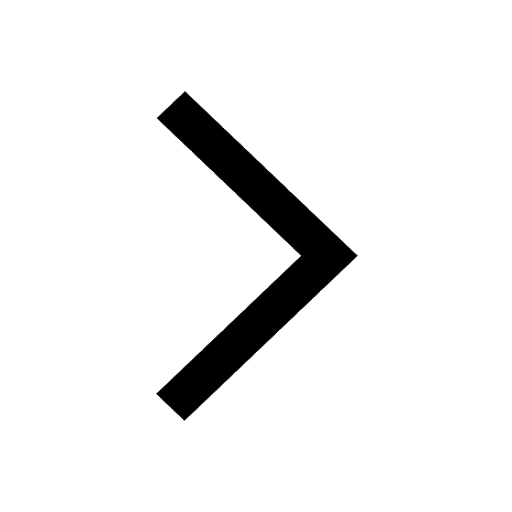
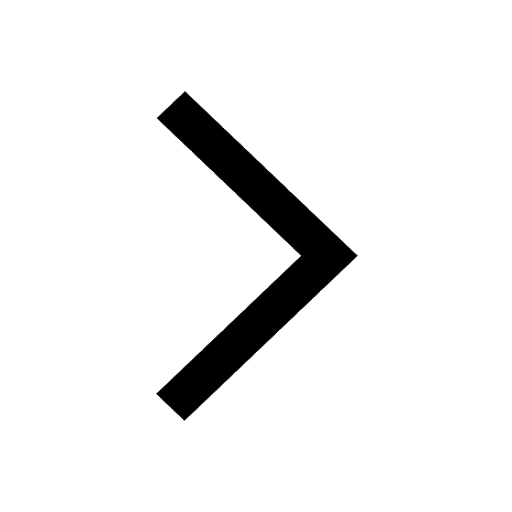
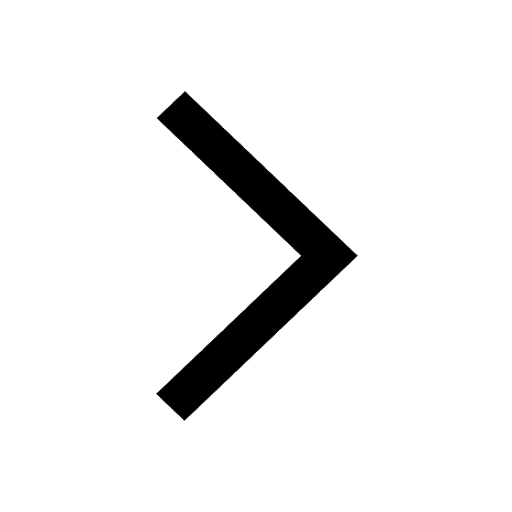
FAQs on What are the Discrete Facets of Coefficient of Determination?
1. What does Adjusted Coefficient of Determination mean?
The Adjusted Coefficient of Determination denoted as (Adjusted R-squared) is a sort of rearrangement for the Coefficient of Determination that considers the number of variables in a data set. It also inflicts a penalty for points that don’t accommodate the model.
You may know that some values in a data set (particularly, a too-small sample size) can result in deceptive data, but you might not know that excessive data points too can induce certain issues. That is to say, each time you add a data point in regression analysis, R2 will show an increase and then never decrease. Thus, the more points you add, the better the regression will appear to “accommodate” your data. If your data doesn’t quite seem to accommodate a line, it can be irresistible to keep on adding data until you obtain a satisfactory fit.
2. What is the best use of the Coefficient of Determination?
The most common usage of (R²) is perhaps how well the regression model accommodates the assessed data. For example, an R² of 80% exhibits that 80% of the data “accommodate” the regression model. Usually, a larger coefficient signifies a better fit for the model. Though it does not make for a universal truth that a large r-squared is superlative for the regression model. Having said that, the quality of the coefficient is dependent upon several factors, including the units of the variables, the characteristic of the variables executed in the model, and the used data transformation. Therefore, even a large coefficient can sometimes induce problems with the regression model.