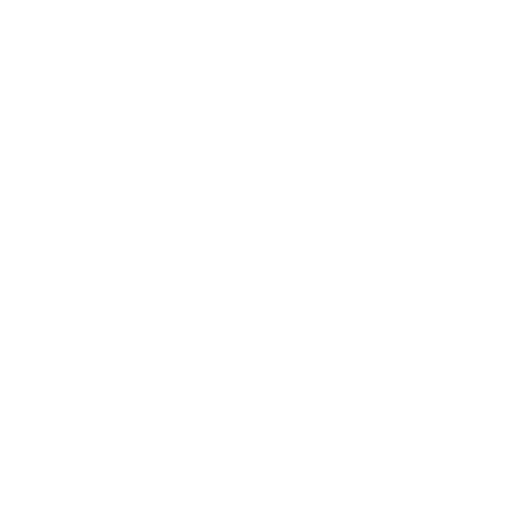
Types of Data
One of the important concepts in statistics is the types of data being collected and analysed. It needs to be understood thoroughly to apply appropriate statistical measurements to your data and to conclude specific assumptions about it correctly. The two processes of data handling and classification involve a multitude of tags and labels to define data, confidentiality, and integrity. In this chapter, you will learn about different data types you need to know to carry out proper exploratory data analysis (EDA).
Classification of Data in Statistics
Introduction to Types of Data
One must have a good understanding of measurement scales, also known as different data types. It is a vital requirement to perform Exploratory Data Analysis (EDA). Here, you can use specific statistical measurements only for certain types of data. Also, you must know to deal with which data type must be appropriate to choose the right visualization method. A data type is a way to categorize different types of variables.
Here we will take an in-depth look into the classification of data in statistics and also explain the example for each. We will refer to them as measurement scales.
Qualitative or Categorical Data
Qualitative data, otherwise known as categorical data, is the data that fits into the categories. Qualitative data does not deal with numerical. Information related to group relates to variables that describe the features like individuals, gender, language, hometown, etc. categorical measures are not defined in terms of numbers but in terms of the natural language specification. Sometimes it may hold numerical values, but the values do not have mathematical sense. For instance, (0 for male and 1 for female) some other examples are favourite sport, birthdate, school postcode, etc. school postcode and birthdate hold the quantitative value but do not have numerical meaning.
Nominal Data
Nominal scales without any quantitative value are used for labelling variables. Nominal scales are also known as labels. Nominal data has no order; therefore, it changes the order of its value, meaning it does not change. Sometimes nominal data can be quantitative as well as qualitative.
Example of Nominal Data:
A person’s gender would be called dichotomous which is a type of nominal scales that contain only two categories.
The examination of nominal data is done using grouping method, where data is grouped into categories and only then the percentage of the data or the frequency is calculated. Pie charts visually represents this data.
A person's gender would be called dichotomous, a type of nominal scale containing only two categories.
The examination of nominal data is done using the grouping method, where data is grouped into categories, and only then the percentage of the data or the frequency is calculated. Pie charts visually represent this data.
Ordinal Data
In ordinal data scales, the order of the value is significant and vital, but the difference between them is not known yet. When referring to the example given below, in each case, number 4 is better than number 3 or 2, but it cannot be quantified how much better it is. For instance, we cannot predict the difference between ‘unhappy’ and ‘ok’ and the difference between ‘very happy’ and ‘happy.’
Ordinal scales usually the measure of non-numeric concepts like happiness, satisfaction, discomfort, etc. it is easy to remember ordinal scales as it sounds like an order, and that is the main thing to remember with ordinal scales. Orders are what matters, and that is what you get from these.
The prominent way to determine the central tendency on a set of ordinal data is using mode or median. A purist will tell you that it cannot be defined from an ordinal set.
Example of Ordinal Scale:
Numerical or Quantitative Data
Quantitative data are also known as numerical data representing numerical value like how many, how often, etc. it provides information on quantities of specific things. Some unique examples of numerical data are length, weight, height, size, and so on. Based on the data set, quantitative data is classified into two different types; those are discrete data and continuous data.
Discrete Data
Discrete data has values that are separate and distinct. Discrete data is useful if taken only on specific values. This data cannot be measured, but it can be counted. It typically represents information that is categorized into classification. The best example is the number of heads in 100-coin flips. Discrete data can be known by asking the following question.
Can you count the data?
Can it be divided into smaller and smaller parts?
Example: number of employees in an organization
Continuous Data
Continuous data represent measurements. Its values can be measured and not counted. For example, the height of a person is described using intervals on the real number line.
Interval Data
Ordered units that have the same difference represents interval values. Interval data is used when variables that contain numeric values are ordered and where we know the exact difference between the values.
Example of interval data: a feature that contains the temperature of the given place
Temperature?
-10
-5
0
+ 5
+10
+15
Interval values do not have true zero. In the example above, there is no such thing or option as no temperature. We can add and subtract in interval data, but cannot divide, multiply, and calculate the ratio. You cannot apply inferential and expressive statistics as there is no zero.
Ratio Data
Like interval data, even ratio values have ordered units that have the same difference. The only difference ratio value has is that they have an absolute zero.
Examples are weight, length, height, etc.
Length (inch)?
0
5
10
15
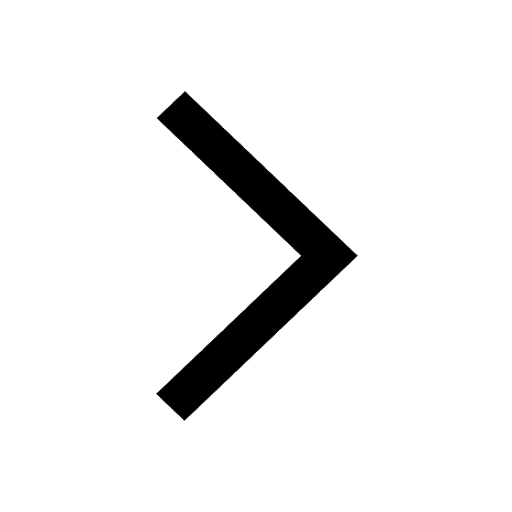
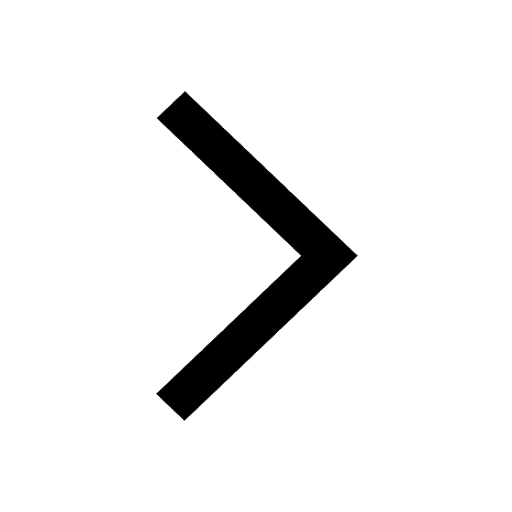
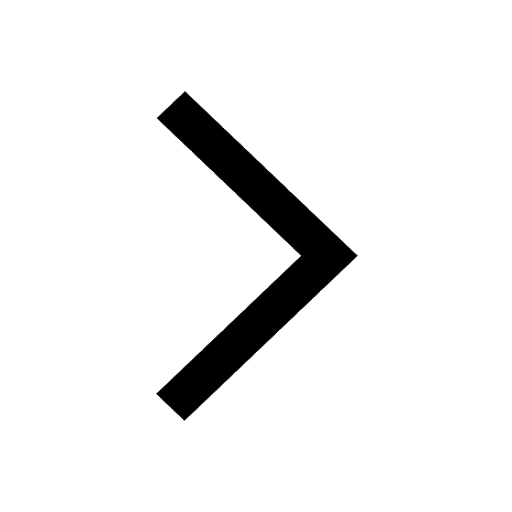
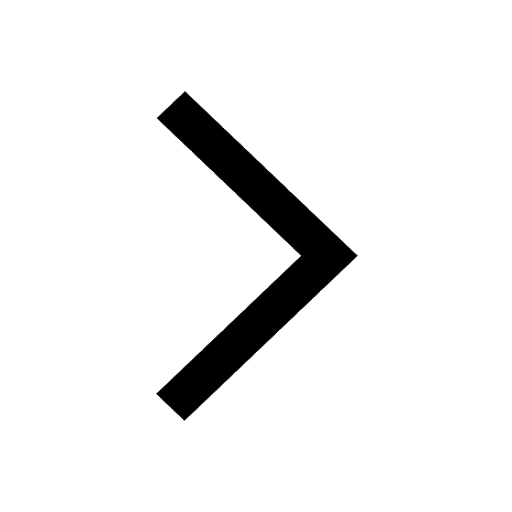
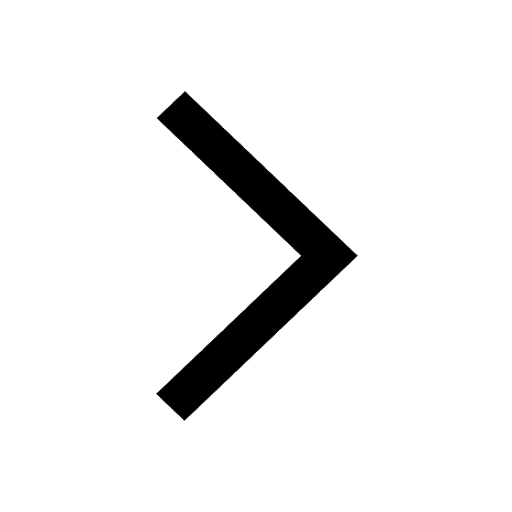
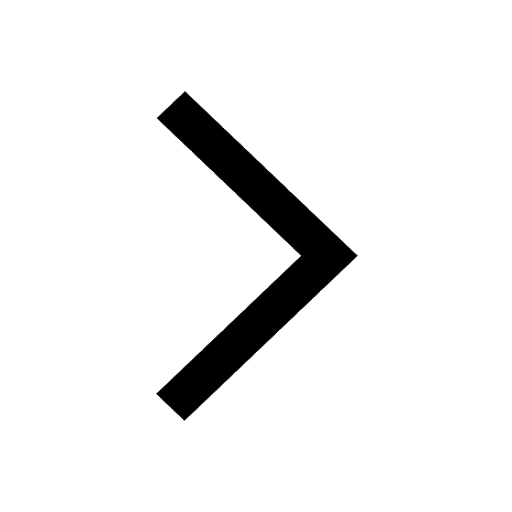
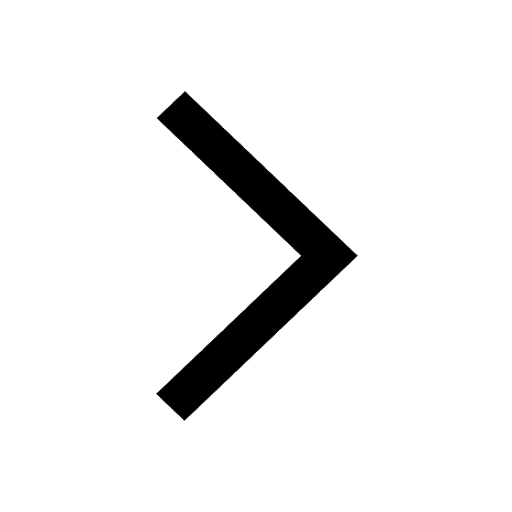
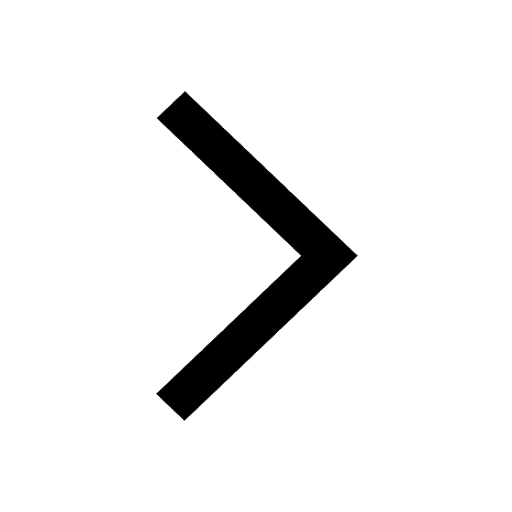
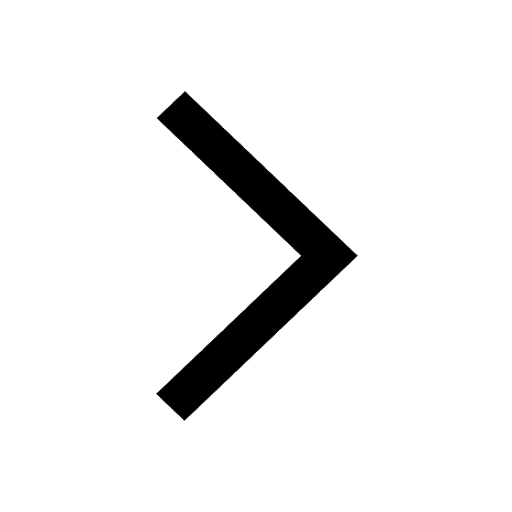
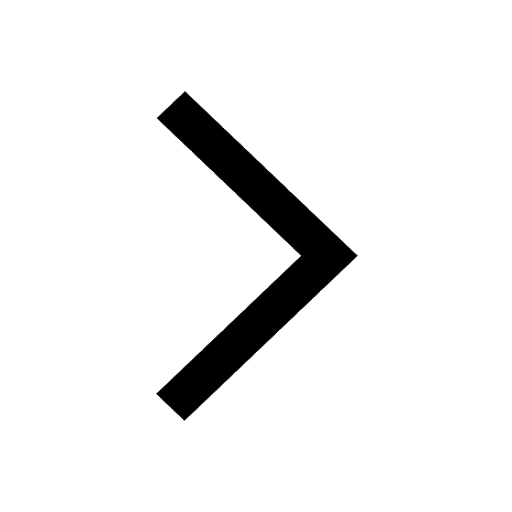
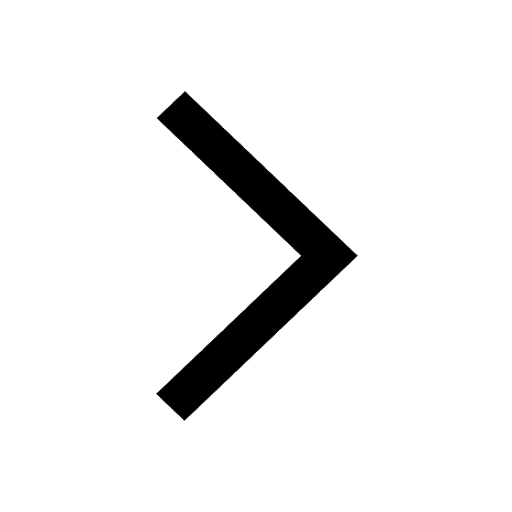
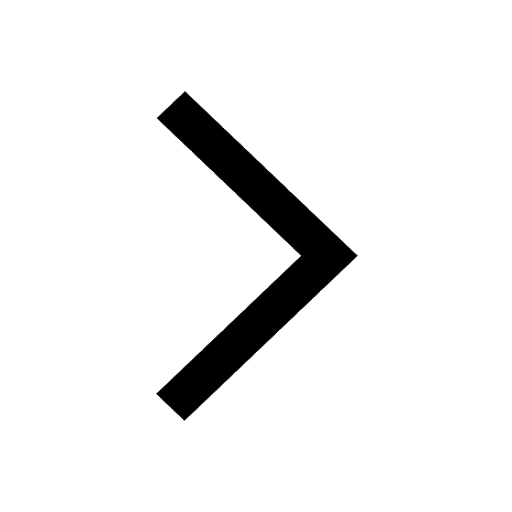
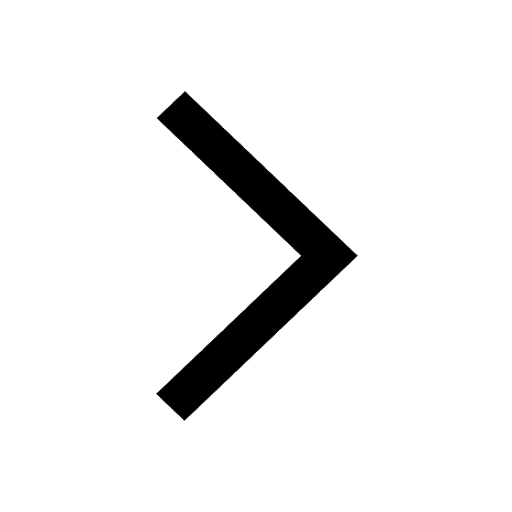
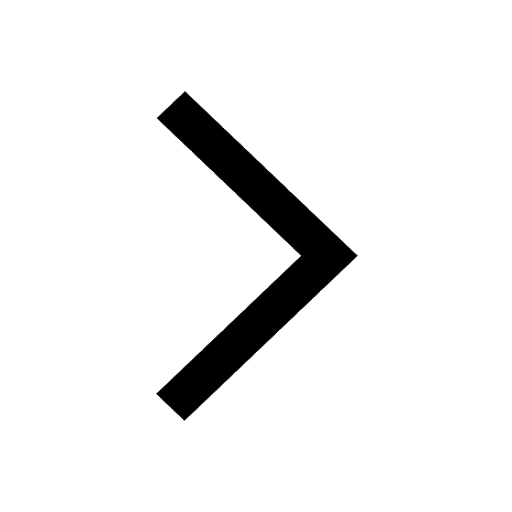
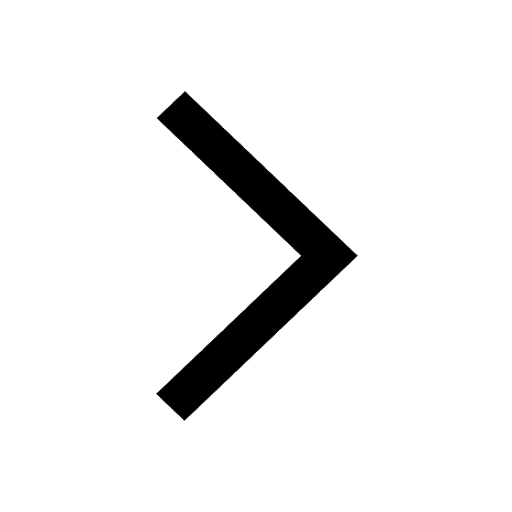
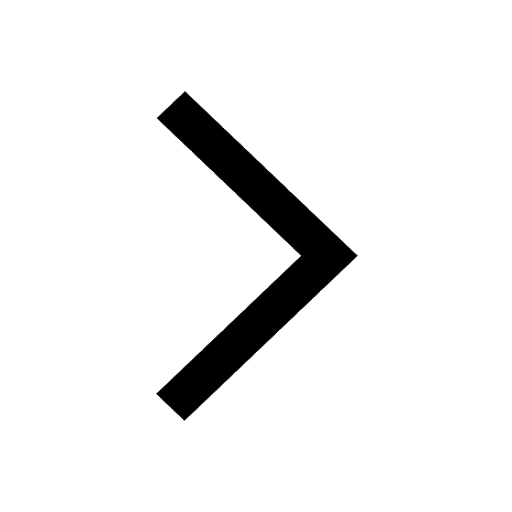
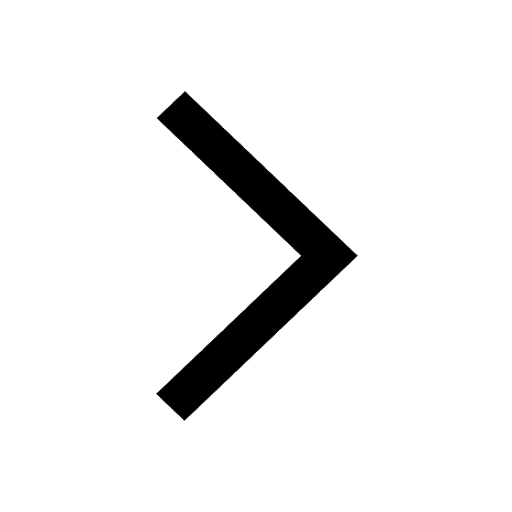
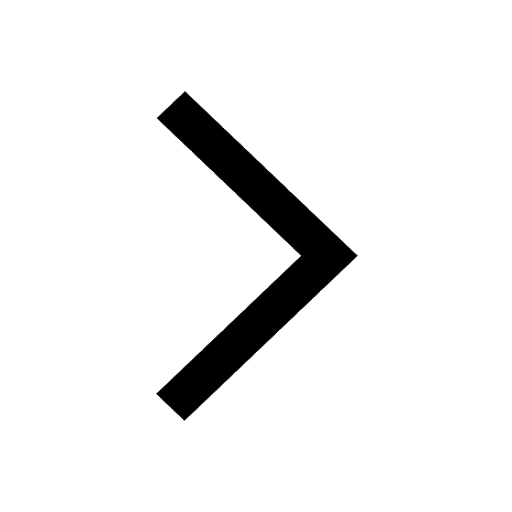
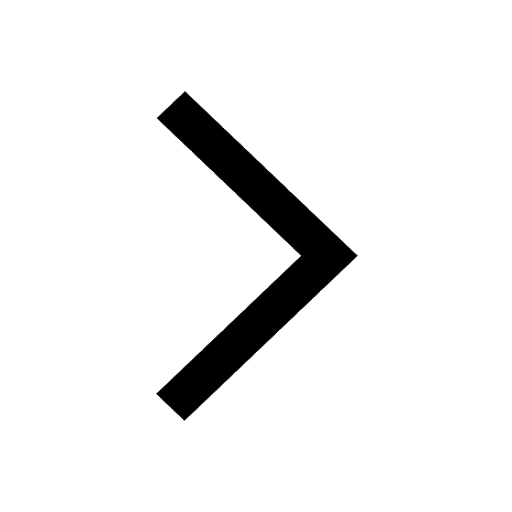
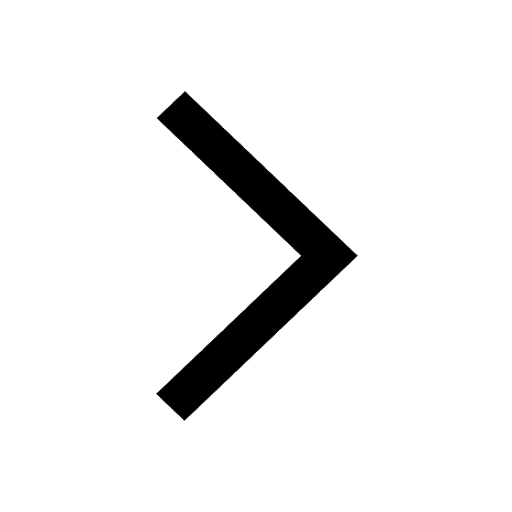
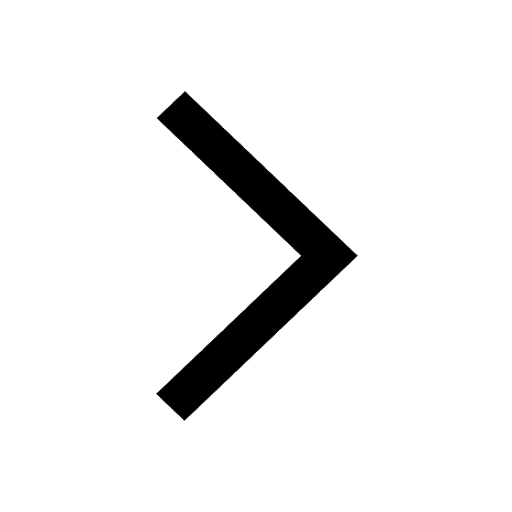
FAQs on Types of Data in Statistics
1. Explain the classification of data into two main terms
At the highest level, data can be classified into two broad forms. The two main types of data are qualitative and quantitative. Qualitative data represent attributes or characteristics. It also represents descriptions that we can observe but cannot calculate or compute. Examples include smell, taste, attractiveness, or intelligence. So, when you judge or classify something, you create qualitative data. On the other hand, quantitative data can be measured and not observed. It is represented numerically, and we can also perform calculations. Examples include age, prices, amount, height, and length. So, when you measure something within a number value, you create quantitative data.
2. What is discrete data?
As we saw, the broadest categories of data are qualitative and quantitative. Now, under quantitative there are two more categories – continuous and discrete data. In discrete data, we can take into consideration only specific values instead of a range of values. An excellent example of discrete data is the data in a population’s blood group or on their genders. A common way to represent this data is bar charts. These values cannot be more precise and whole. So, for example, a person can’t say that he has 2.3 children. So basically, discrete data counts whole indivisible things or units.