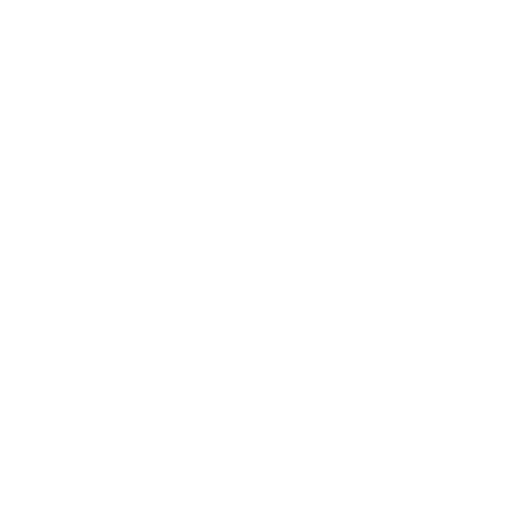
What is T-Distribution?
T-distribution, also known as student’s t-distribution, is a statistical methodology of evaluating or calculating the mean of a data set that is normally distributed.
A normally distributed data is the one with a “bell-shaped” or “an inverted U shaped” curve. The shape of the distribution implies that slope is concentrated at the center, at the mean value, and slopes downwards towards either side of the curve where the extreme values are. T-distribution and t-test form a part of inferential statistics. The concept of t-distribution was developed by William Sealy Gosset.
The aim of t-distribution is used to test the hypothesis and whether it should be accepted or rejected. It is used to estimate the mean of a population that is normally distributed. It is commonly used when the sample size is small (not less than 20) and when the variance or standard deviation is unknown. It is used to compute the probabilities with the sample mean.
the Formula Used to Calculate the T-value is Given Below:
t - is the t-test score,
x̅ - is the mean of the sample,
μ - is the mean of the population,
s - is the calculated or given standard deviation of the sample,
N - is the sample size
When the values of the above-given variables are provided, then one can simply calculate the t-score.
Let’s work on some examples to understand this better.
Example:
Question
There is a class of 25 students and the mean score of their test is 60 out of 100, with standard deviation 4 marks from the mean. While other students of the school have a mean score of 50 on the same test. What will be the t-score for calculating the probability that school students scored not less than 60 in their tests?
Solution
Let us begin assembling the values given in the question. From the question we can infer that the sample here is the class students and the population consists of all the students in the school.
The samples size of the class (N) - 25
Mean score of the class (x̅) - 60
Mean score of the population ( μ) - 50The standard deviation of the sample (s) - 4
Since we have got all the values that are required to calculate the t-score, we can simply insert them in the formula below
t = ( x̅ - μ) ÷ (s / √N),
t= (60 - 50) ÷ (4 / √25)
t= 10 ÷ 0.8
t= 12.5
The t-value obtained here leads to the cumulative probability from the t-distribution table from where you can find the log value of this t-score with the degrees of freedom, the sample means, the population means and standard deviation for this sample.
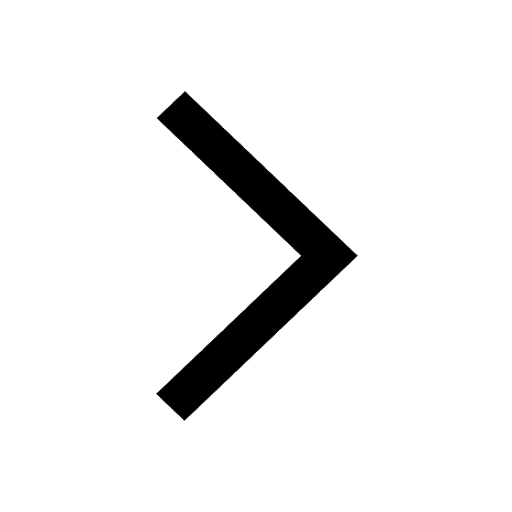
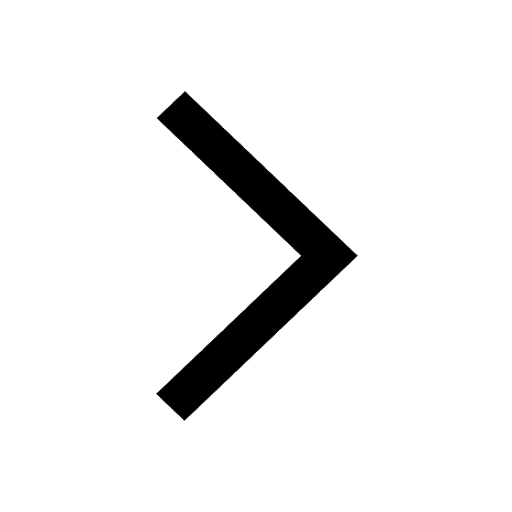
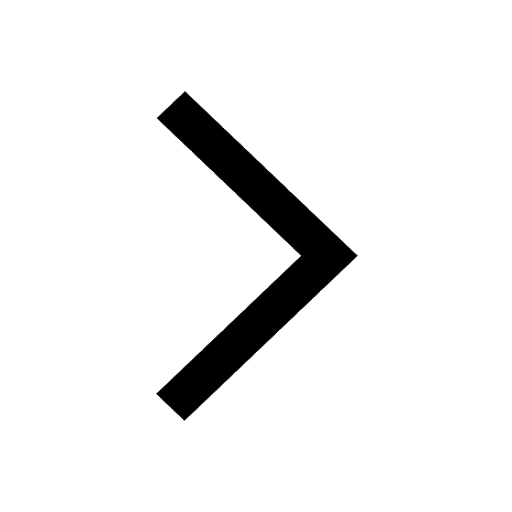
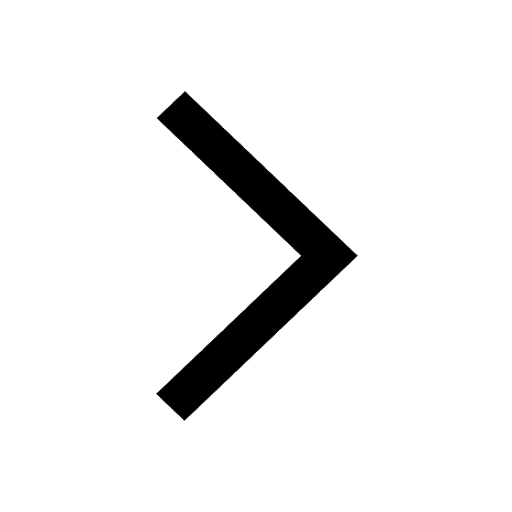
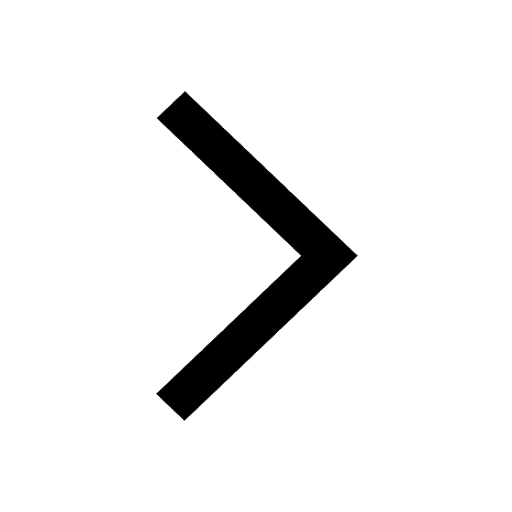
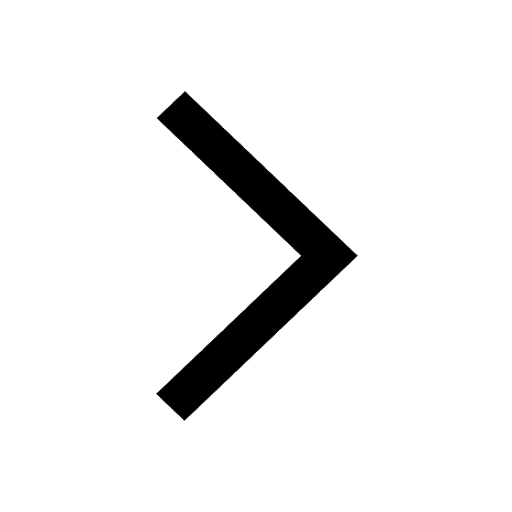
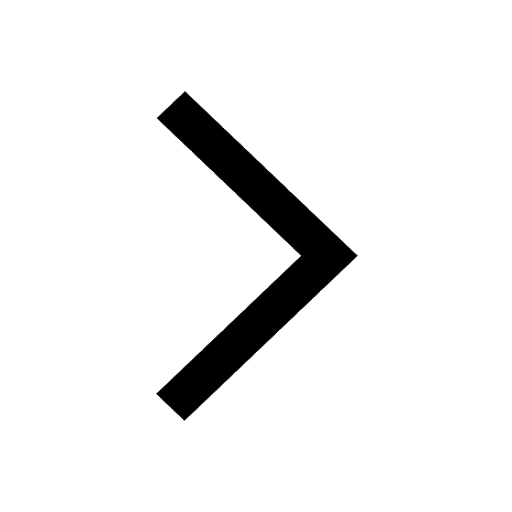
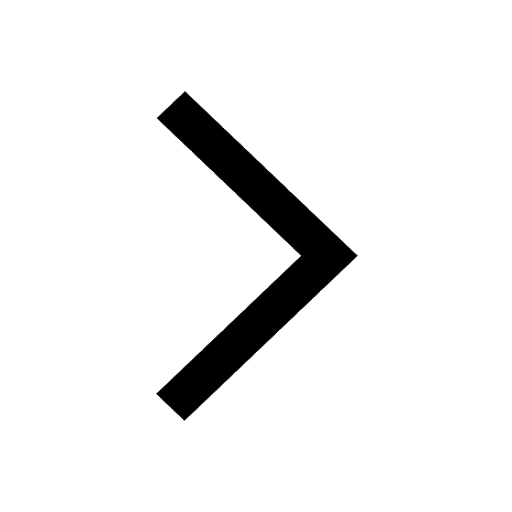
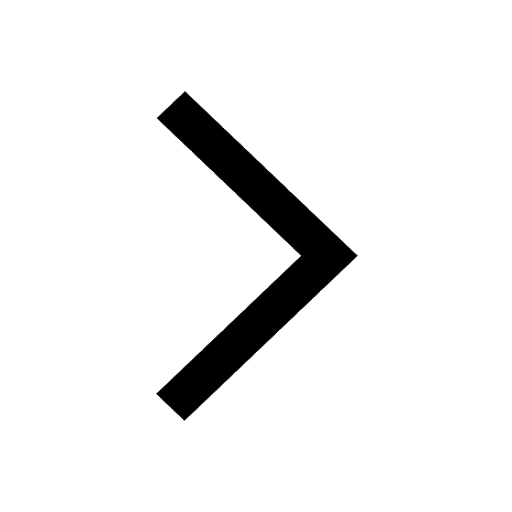
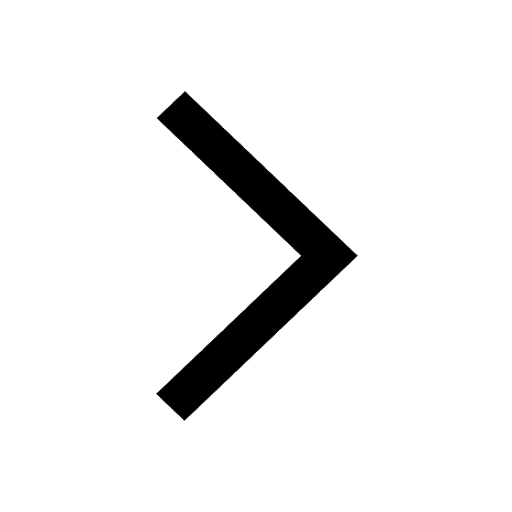
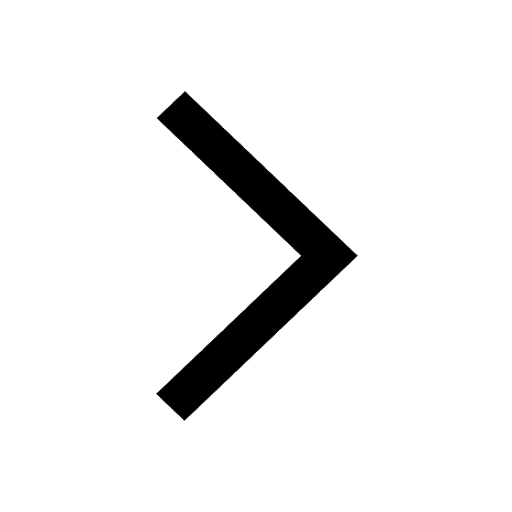
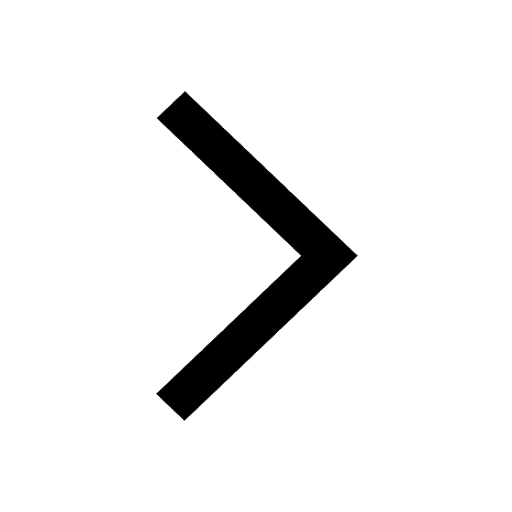
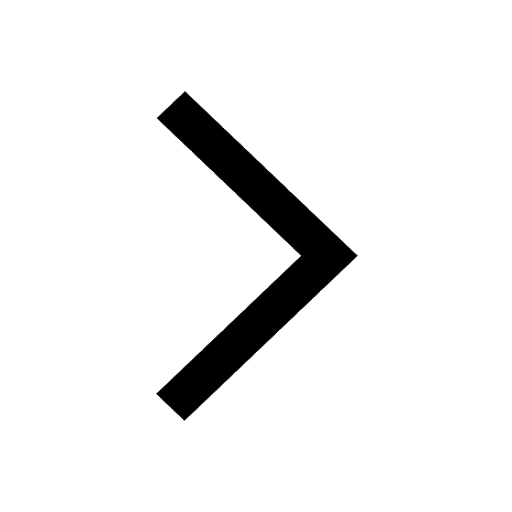
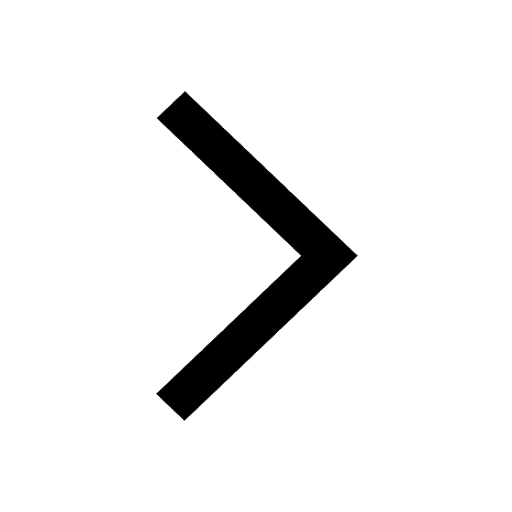
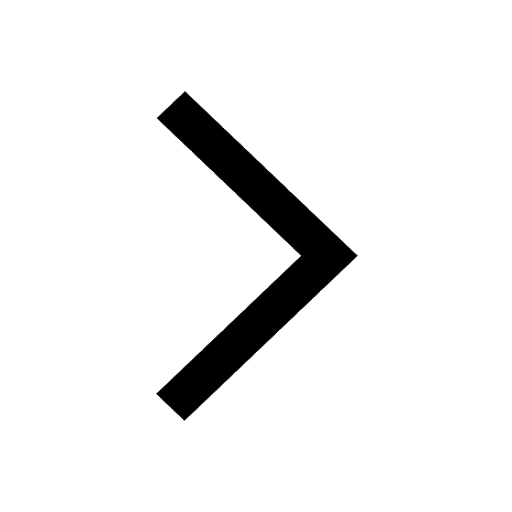
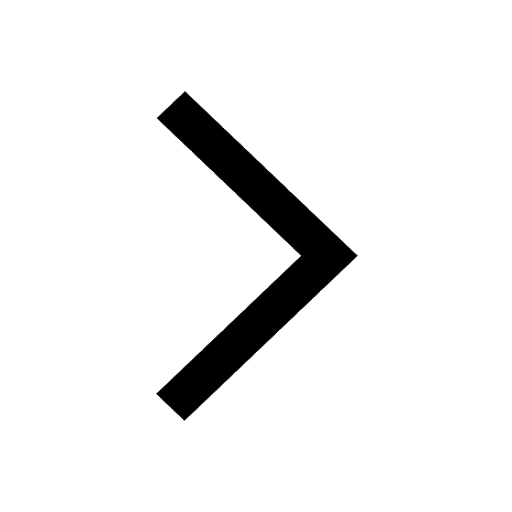
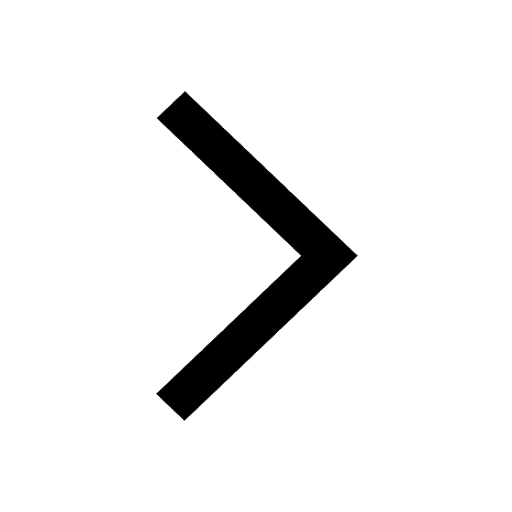
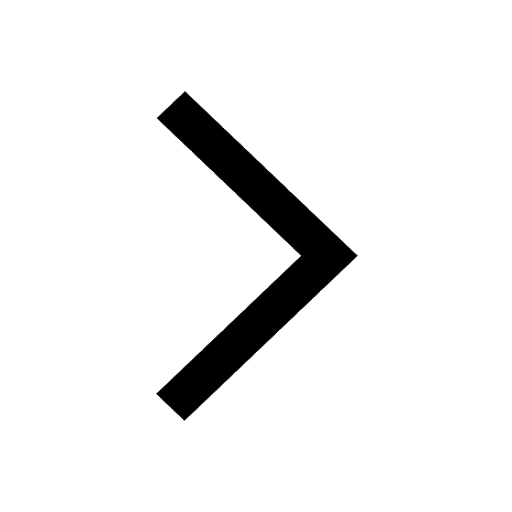
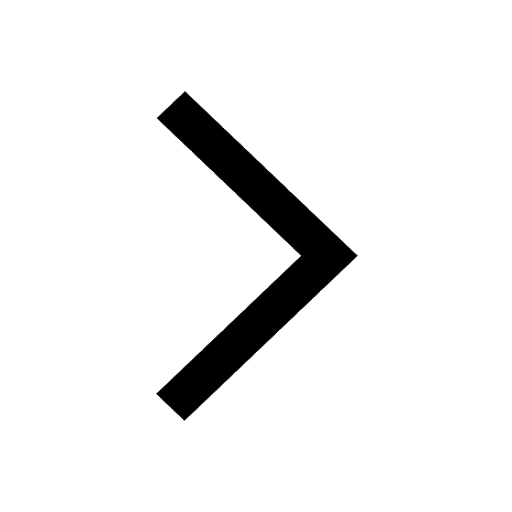
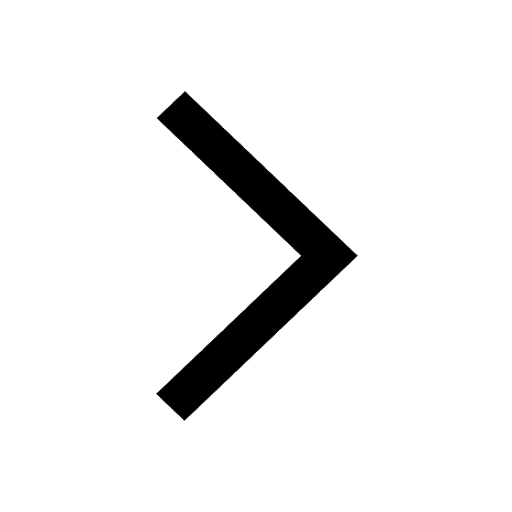
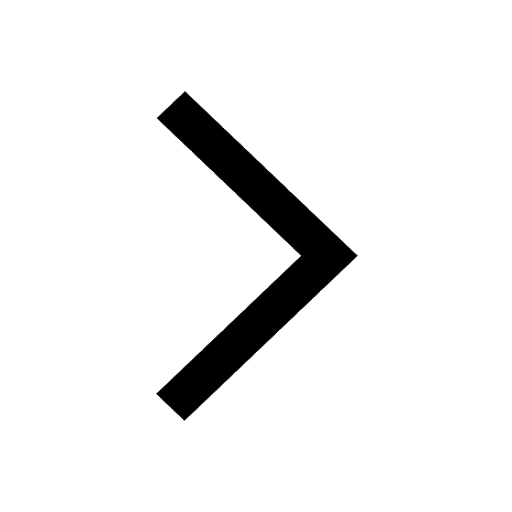
FAQs on T-Distribution
1. What are the Properties of T Distribution?
Answer: It is important to be aware of the prominent properties of t-distribution.
The t-distribution shape is dependent on the sample size.
A bell-shaped curve is observed in at distribution.
The range for t-distribution can be from infinity to infinity.
As compared to a normal distribution, t-distribution is less peaked at the center and more elevated on either side.
The standard deviation for t-distribution has to be more than 1.
In the case of t-distribution, whenever the sample size increases it begins to take up the form of a normal distribution
The total area covered under the t-distribution is considered 1.
The bell-shaped curve obtained in a t-distribution is always symmetric around 0.
2. What are the Key Terms that are Used in T Distribution?
Answer: Some key concepts of t distribution are -
Mean - In statistics, mean is the central point or value derived from the average of all the values in the data set.
Standard Deviation - The purpose of standard deviation is to reflect how well spread the numbers are from the center or the mean value.
Sample Size - It is the record of the total participants or items of a particular data set. For example, if a data set has 10 males and 20 females, then the sample size of the set is 30 participants (10+20)
Degree of Freedom - It refers to the number of values that can be allowed to vary in the final calculation. Usually, the degree of freedom is (n-1), where “n” is the sample size