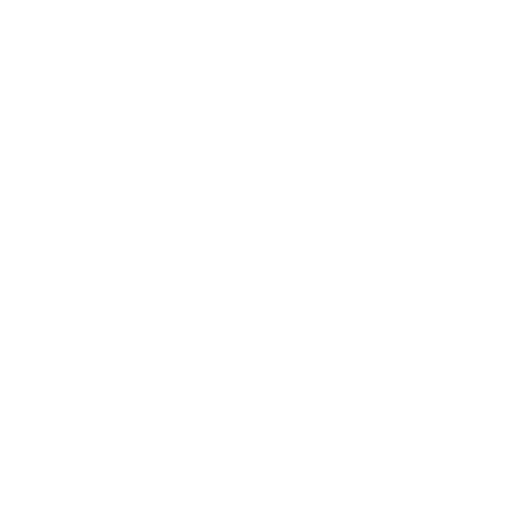
What is Multiple Regression?
Multiple Regression is a set of techniques that describes-line relationships between two or more independent variables or predictor variables and one dependent or criterion variable. A dependent variable is modeled as a function of various independent variables with corresponding coefficients along with the constant terms. Multiple regression requires multiple independent variables and, due to this it is known as multiple regression. In multiple regression, the aim is to introduce a model that describes a dependent variable y to multiple independent variables.In this article, we will study what is multiple regression, multiple regression equation, assumptions of multiple regression and difference between linear regression and multiple regression.
Multiple Regression Equation
There is only one dependent variable and one independent variable is included in linear regression whereas in multiple regression, there are multiple independent variables that enable us to estimate the dependent variable y.
Multiple regression equation is derived by:
Y = a + b1*1 + b2*2 + b3*3……………. bk*k
Here, y is an independent variables whereas b1, b2 and bk
Multiple Regression Analysis Definition
Multiple regression analysis provides the possibility to manage many circumstances that simultaneously influence the dependent variable. The aim of regression analysis is to design the relationship between a dependent variable and multiple independent variables. Let k represent the number of variables and represented by b1, b2, b3, ……, bk. Such an equation is useful for the estimation of value of dependent variable i.e, y when the values of x are determined.
Multicollinearity
Multicollinearity is a term used to describe the case when the inter-correlation of independent variables are high.
Multicollinearity Testimony
The high correlation between pairs of independent variables.
The magnitude or symbols of regression coefficients do not make substantial sense.
Non-significant regression coefficients on significant independent variables
The utmost sensitivity of magnitude or sign of regression coefficients leads to the insertion or deletion of an independent variable .
Multiple Regression Assumptions
There should be systematic specification of the model in multiple regression. It implies that only relevant variables should be included in the model and the model should be accurate.
Assumption of linearity is necessary.
The multiple regression model should be linear in nature.
Assumption of normality is necessary in multiple regression. It implies that in multiple regression, variables must have normal distribution.
Assumption of Homoscedasticity is necessary in multiple regression
The variance is constant across all levels of the independent variable.
The independent variables are not highly correlated with each other.
There are various terminologies that help us to understand multiple regression in a better way. These terminologies are as follows:
The beta value is used in measuring how effectively the independent variable influences the dependent variable. It is measured in terms of standard deviation.
R, is the measure of linkage between the observed value and the predicted value of the dependent variable. R Square, or R², is the square of the measure of association which represents the percentage of overlap between the independent variables and the dependent variable. Adjusted R² is an estimate of the R² if you make use of multiple regression models with a new data set.
Quiz Time
1. Multiple Linear Regression is a Kind of _________ of Statistical Analysis
Bivariate
Univariate
Multivariate
2. In Multiple Linear Regression, the Square of the Multiple Correlation Coefficient or R2 is Known as the
Variance
Covariance
Cross product
Big R
Coefficient of determination
3. In Multiple Linear Regression, a Residual is the Difference Between Estimated Dependent Variables and Actual Dependent Variables.
True
False
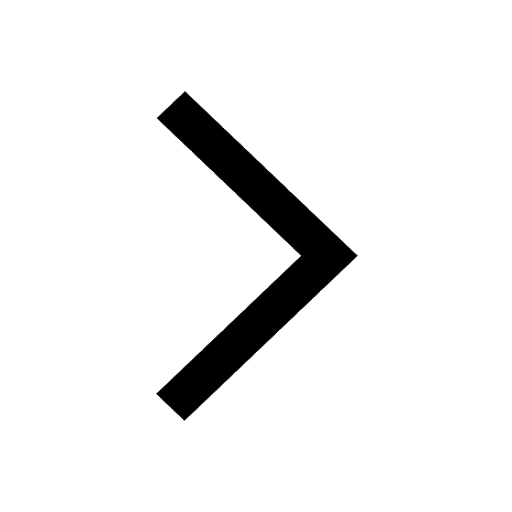
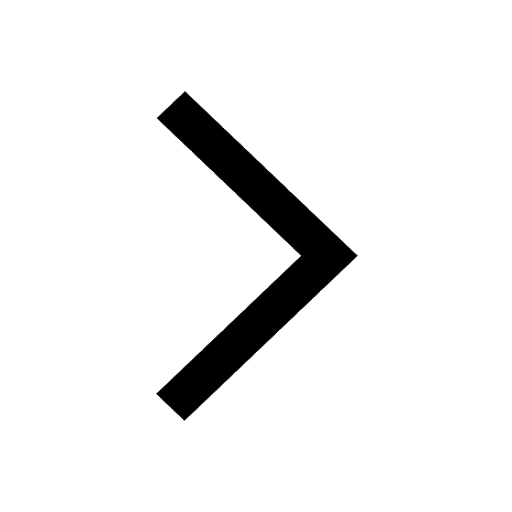
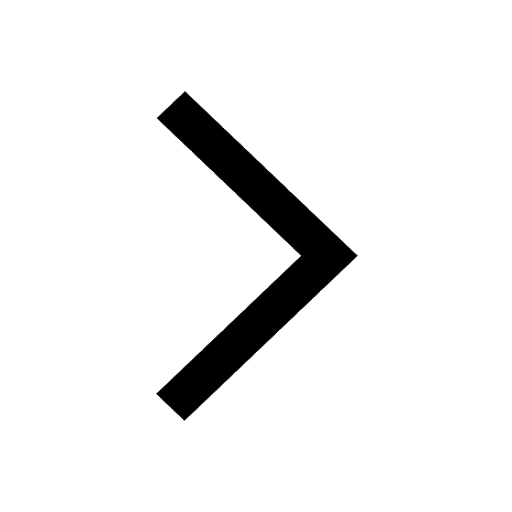
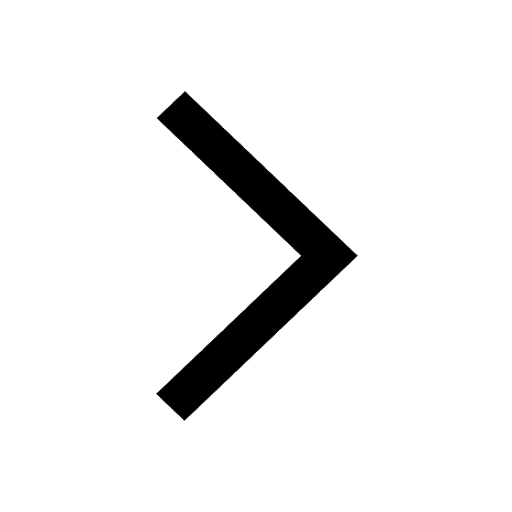
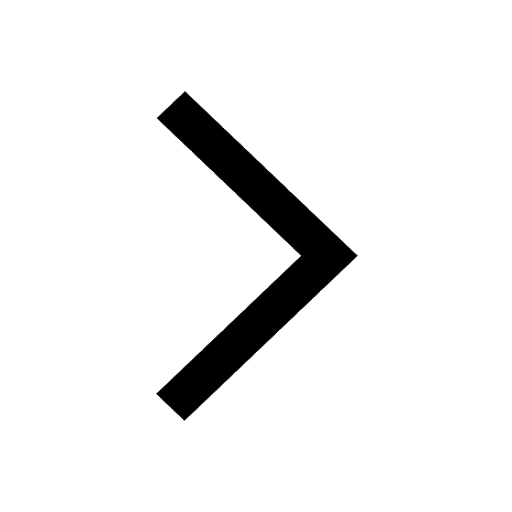
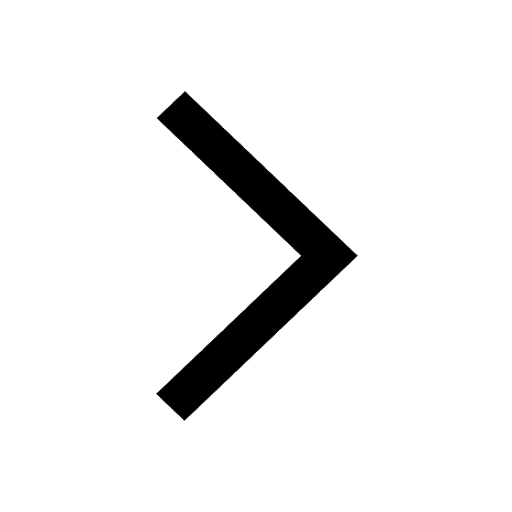
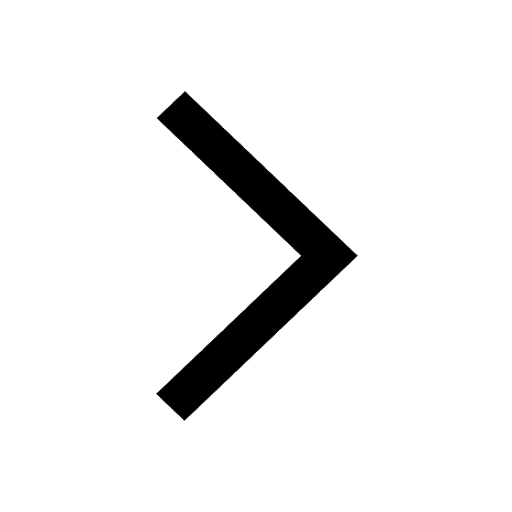
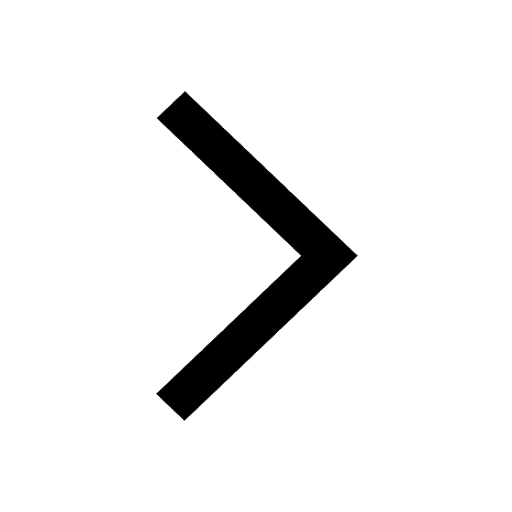
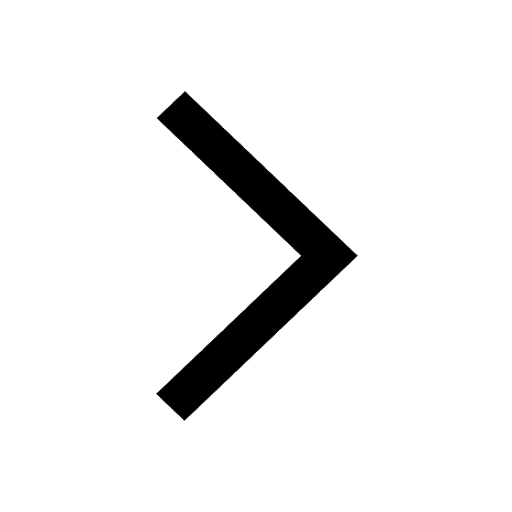
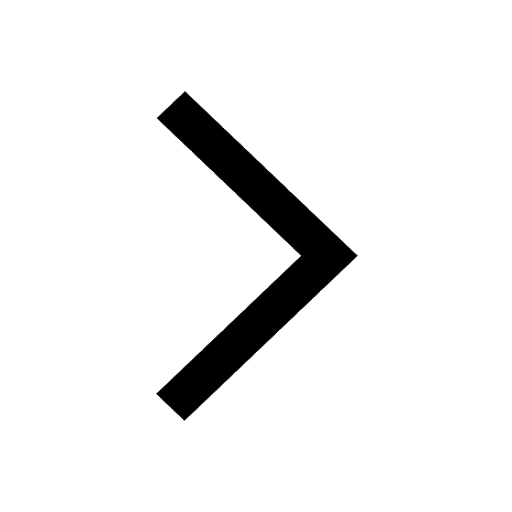
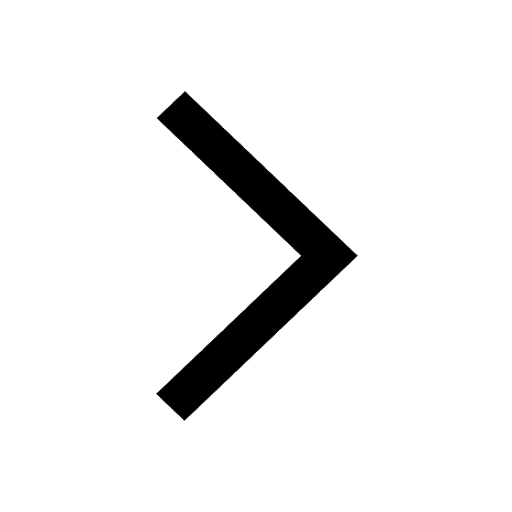
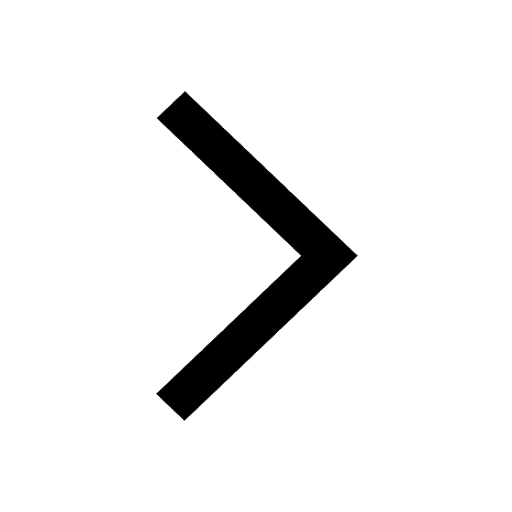
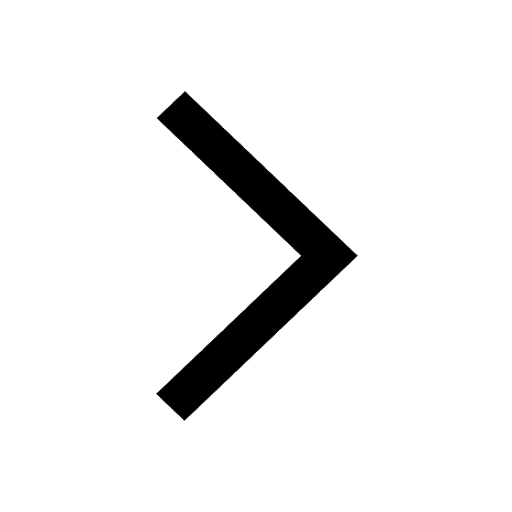
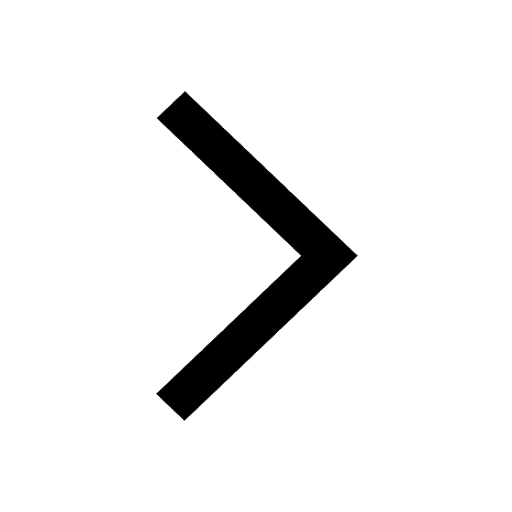
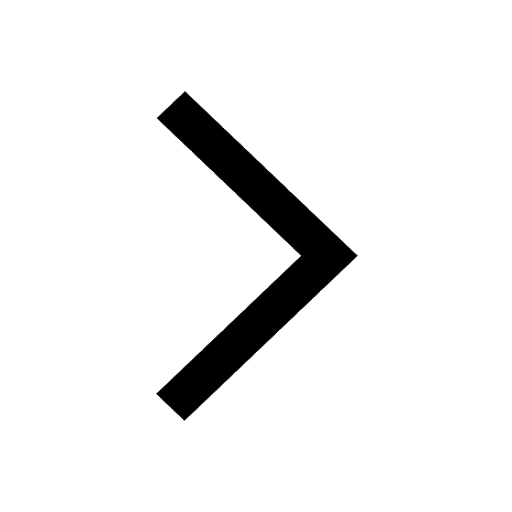
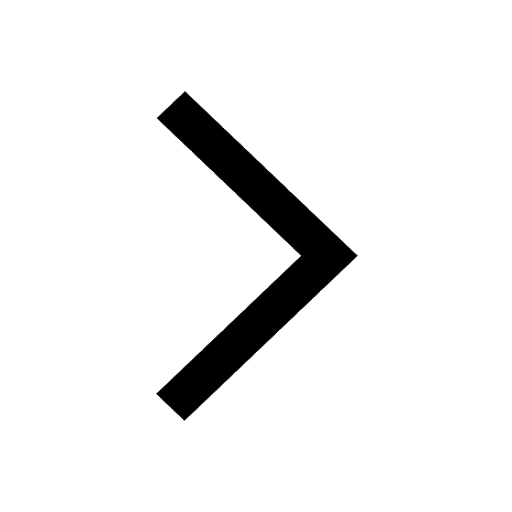
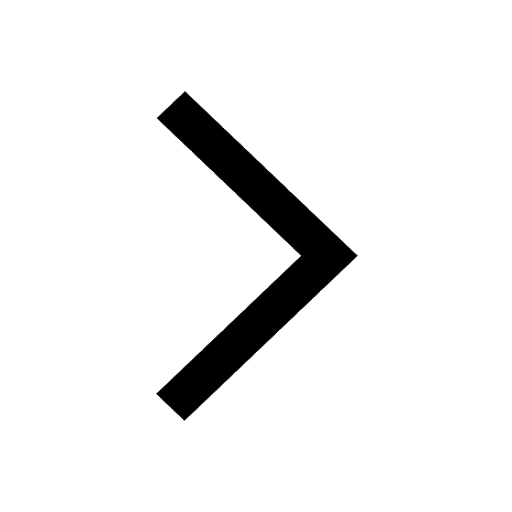
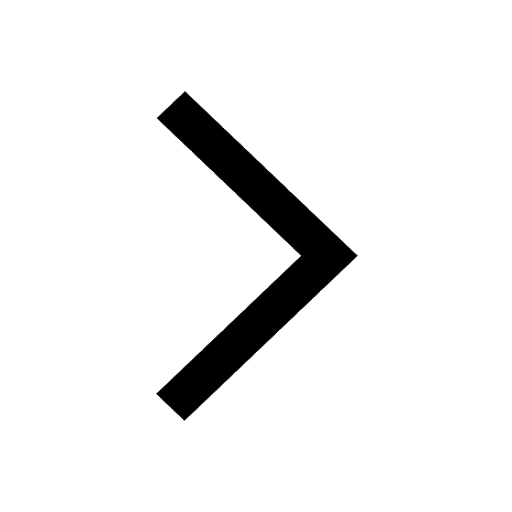
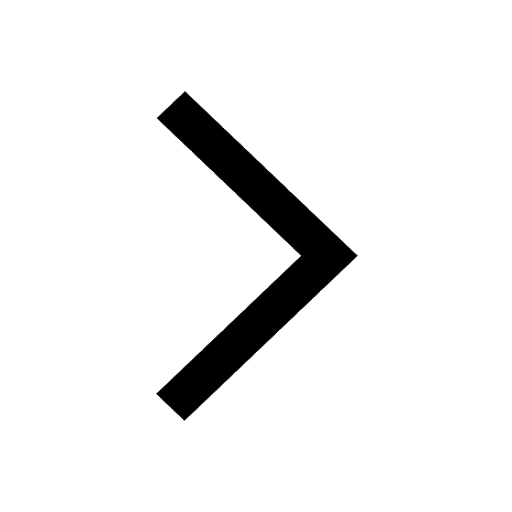
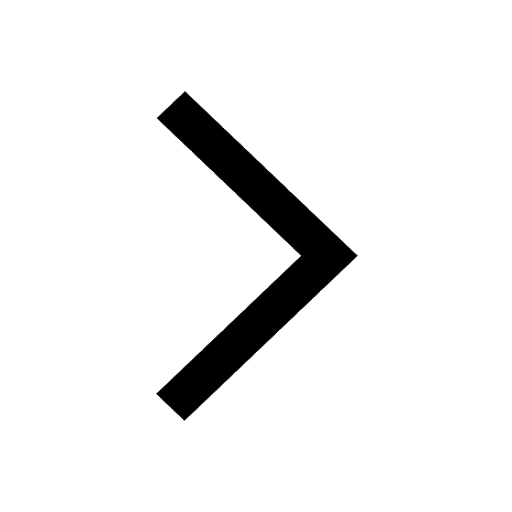
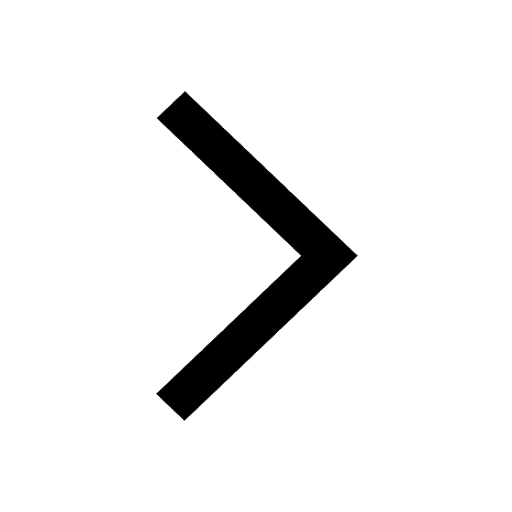
FAQs on Multiple Regression
1. What is the Difference Between Linear and Multiple Regression?
Linear regression differentiate the responses of dependent variables given a change in some descriptive variable. However, it is infrequent that dependent variable is described by only one variable.In this situation, analysts make use of multiple regression which attempts to describe a dependent variable through more than one independent variable. Multiple regression can either be linear or nonlinear.
Multiple regression is based on the assumption that there exists a linear relationship between both the dependent variables and independent variables.It also assumes no major correlation between the independent variables.
2. What are the Advantages of Multiple Regression
There are two important advantages to analyse data using a multiple regression model. These are:
It has the ability to determine the relative influence of more than one independent variable to the criterion values. The real estate agents could find that the room size and the number of bedrooms have a strong correlation to the cost of a house while the proximity to schools has zero correlation or even negative correlation. It is mainly a retirement community.
The second advantage of multiple regression is the ability to identify outliers or anomalies.For example, while examining the data related to management salaries, the human resource manager could find the number of hours worked, the size of the department and its budget. All have a strong correlation to salaries while seniority did not. In other words, it can be said that the independent variables were correlated to each of the salaries being examined, excluding the manager who was being overpaid in comparison with others.