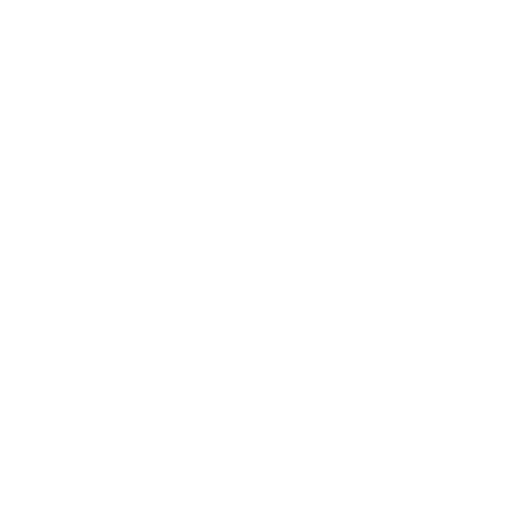
Using a scale range of - 1 and + 1, the extent to which 2 different variables are related can be identified using the correlation coefficient. ‘r’ is the symbol to denote a coefficient of correlation between 2 ratio variables or for 2 intervals. So, r denotes the level of relationship which means, if the r’s value is closer to zero (0), then there is a minimal correlation between the intervals. And if the value of r is higher, then it denotes a greater correlation between each variable, regardless of positive or negative direction. From learning a few applications to understanding its features, this module covers all about the important basics you need to know about the correlation coefficient.
Defining What Coefficient Correlation is
Coefficient of the correlation is used to measure the relationship extent between 2 separate intervals or variables. Denoted by the symbol ‘r’, this r value can either be positive or negative. Some of the other names of coefficient correlation are:
Pearson’s r
Pearson product-moment correlation coefficient (PPMCC)
Pearson correlation coefficient (PCC)
Bivariate correlation
Cross-correlation coefficient
The value expressed will tell us the extent to which the 2 entities are interlinked. Sometimes, r value can 0 also, hence symbolizes that there is an absence of a relationship between the 2 given variables.
The Standard Formulas of Coefficient of Correlation
Let us consider 2 different variables ‘x’ and ‘y’ that are related commonly
To find the extent of the link between the given numbers x and y, we will choose the Pearson Coefficient ‘r’ method. In the process, the formula given below is used to identify the extent or range of the 2 variables’ equality.
Pearson Correlation Coefficient
r = \[\frac{n(Σxy) - (Σx)(Σy)}{\sqrt{[nΣx² - (Σx)²] [nΣy² - (Σy)²]}}\]
The Keys:
“Σx” denotes the number of First Variable Value
“Σy” represents the count of Second Variable Value
“Σx2” gives us the addition of Squares for the First Value
“Σy2” mentioned the sum of the Second Value’s square
“n” is the total number of data quantity which is available
“Σxy” symbolizes the addition of the First & Second Value’s products
Check out the Following Formula:
r = \[\frac{\sum_{i=1}^{n} (X_{i} - \overline{X})(Y_{i} -\overline{Y})} {\sqrt{\sum_{i=1}^{n}(X_{i} - \overline{X}})^{2} \sqrt{\sum_{i=1}^{n}(Y_{i} - \overline{Y}})^{2}}\]
The equation which is given above is termed the linear coefficient correlation formula, “xi” and “yi” denote the 2 different variables and “n” is the total number of observations.
2 of the other important formulas include the following ones.
Population Correlation equation: ρxy = σxy/σxσy (the population standard deviations are “σx” and “σy”. “Σxy” is the population variance)
Sample Correlation equation: rxy = Sxy /SxSy (“Sx” and “Sy” and 2 sample standard deviations. Sample covariance is denoted as “Sxy”)
Simple Examples for Coefficient Correlation with Applications
As we read before, the value of coefficient correlation can be evaluated using - 1 and + 1 respectively. Following 3 are scenarios using these 2 ranges.
When r is + 1: With some fixed proportional value, the variable is said to increase positively by 1 and this increases the other as well. When the size of a fabric material increases, together with the growth and height of an individual is the best example.
When r is 0: Zero represents the complete absence of a relationship between 2 variables. This means there is no recorded history for increase or decrease in its value of extent/range.
When r is - 1: In a standard parameter of fixation, the positive increase in 1 variable will lead to a negative decrease in the other variable. When you drive your car faster than usual, then the upcoming distance to be covered gets reduced. This is a classic example of a negative-valued coefficient correlation.
Speaking of its applications, the coefficient of correlation is majorly preferred in the field of finance and insurance sectors. For instance, the correlation between any 2 different quantities is comparable when the price of an oil product increase, giving better advantages to the oil-producing brand and agencies such as ROI and enhancing consumer behaviour.
Conclusion
The correlation coefficient is the method of calculating the level of relationship between 2 different ratios, variables, or intervals. The symbol is ‘r’. The value of r is estimated using the numbers - 1, 0, and/or + 1 respectively. - 1 denotes lesser relation, + 1 gives greater correlation and 0 denotes absence or NIL in the 2 variable’s interlink. Pearson’s r, Bivariate correlation, Cross-correlation coefficient are some of the other names of the correlation coefficient.
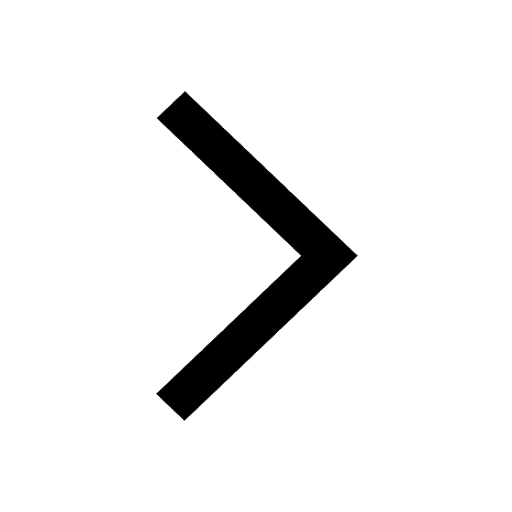
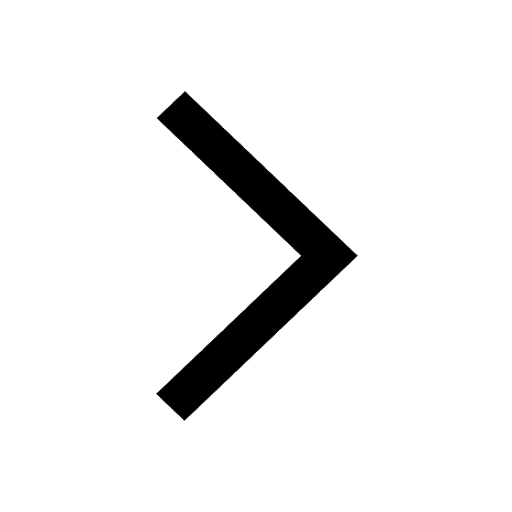
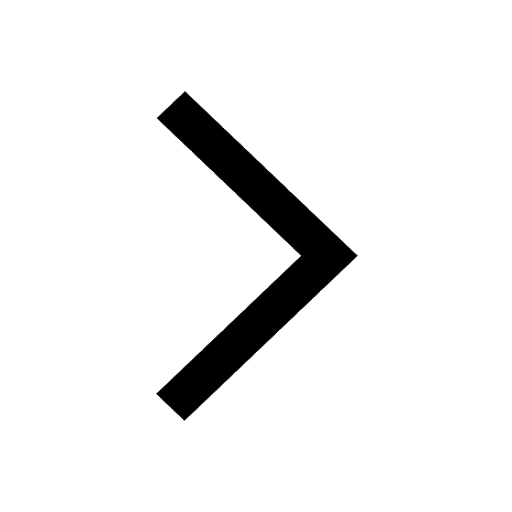
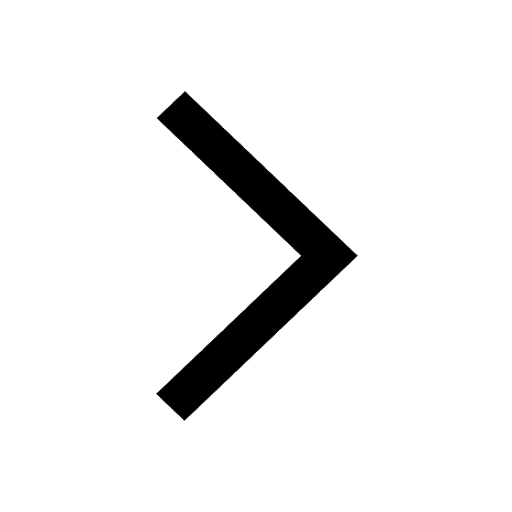
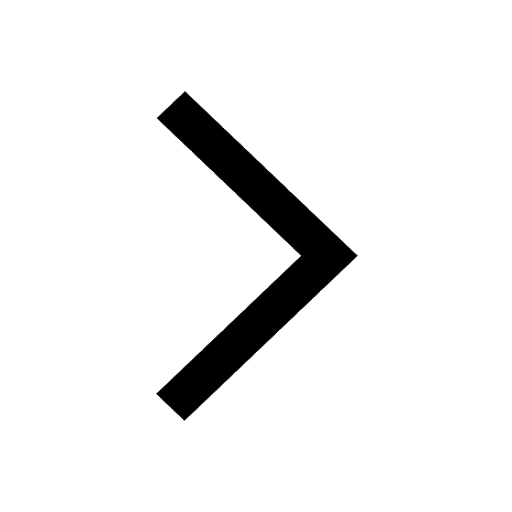
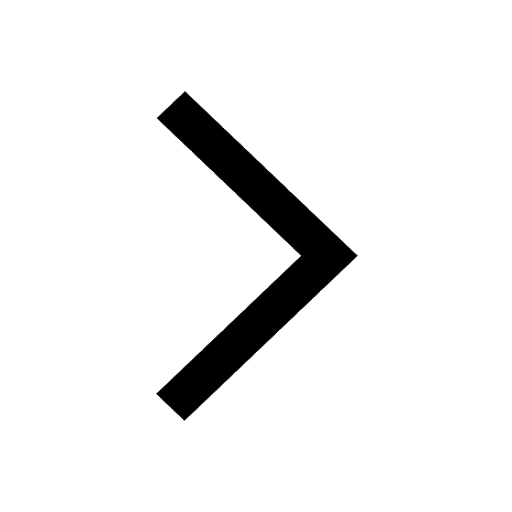
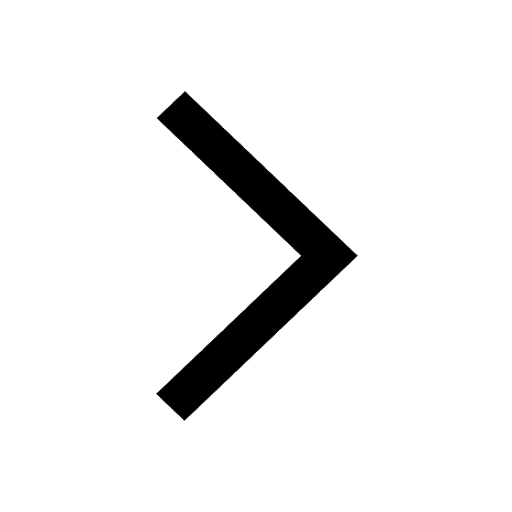
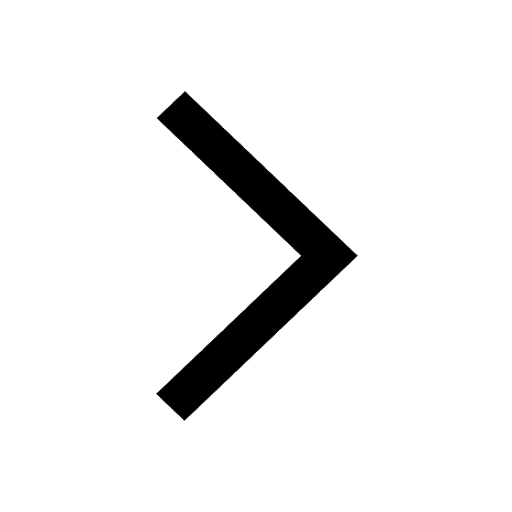
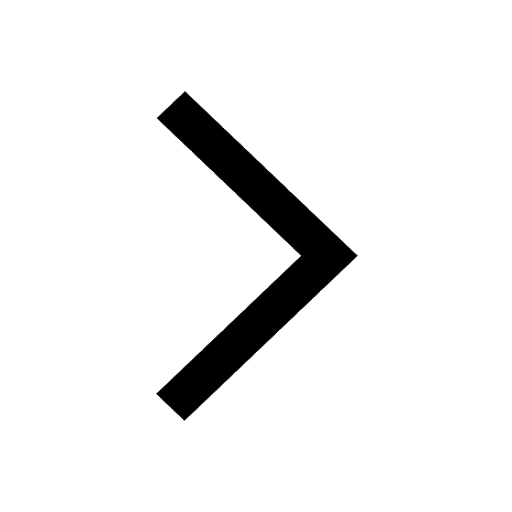
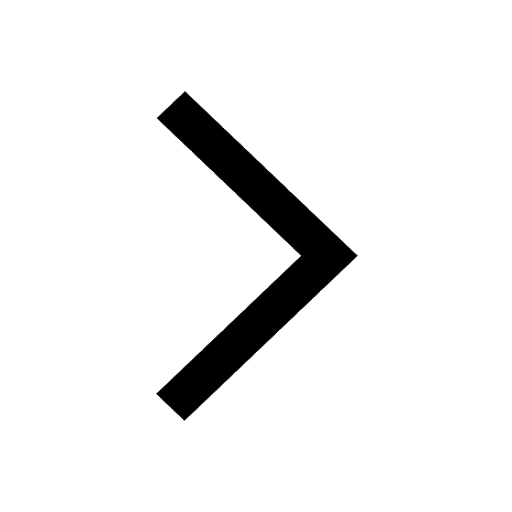
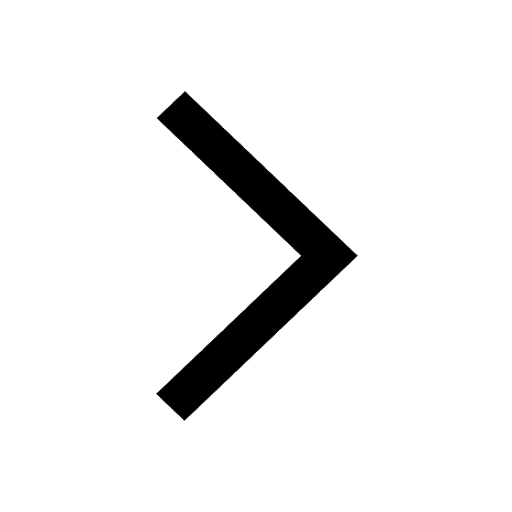
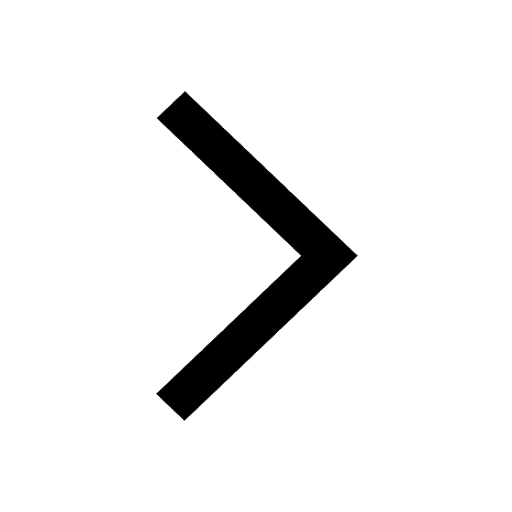
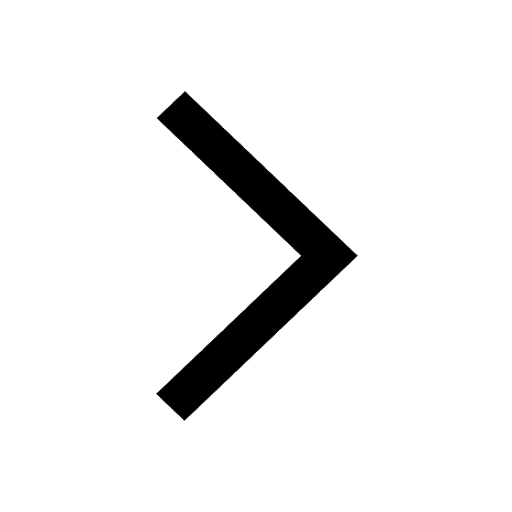
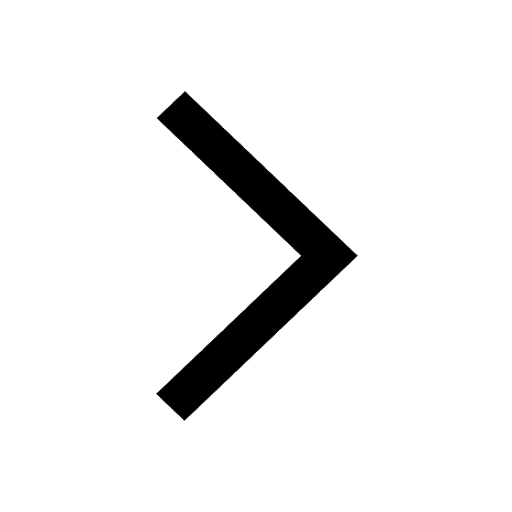
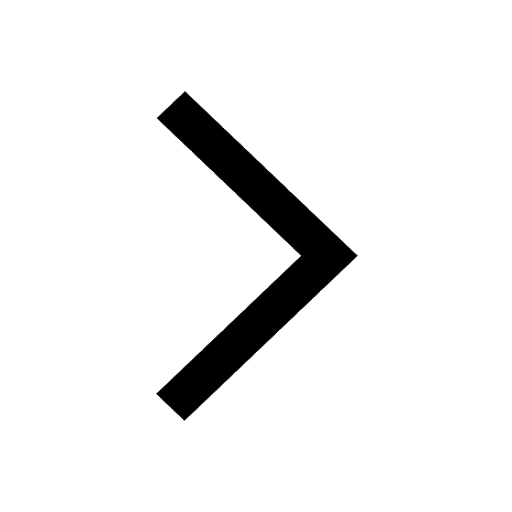
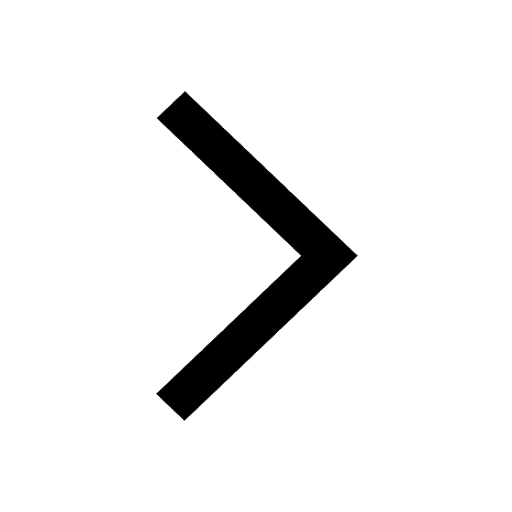
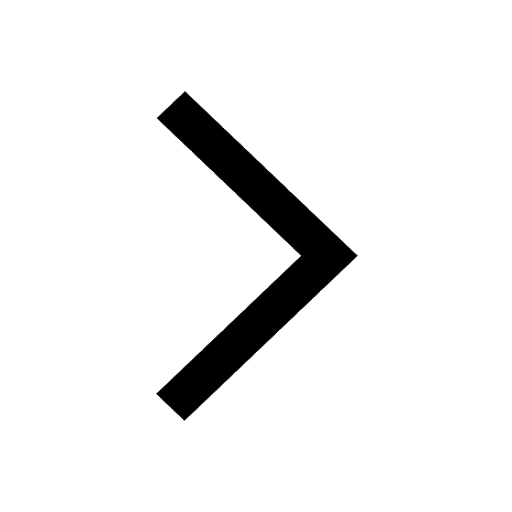
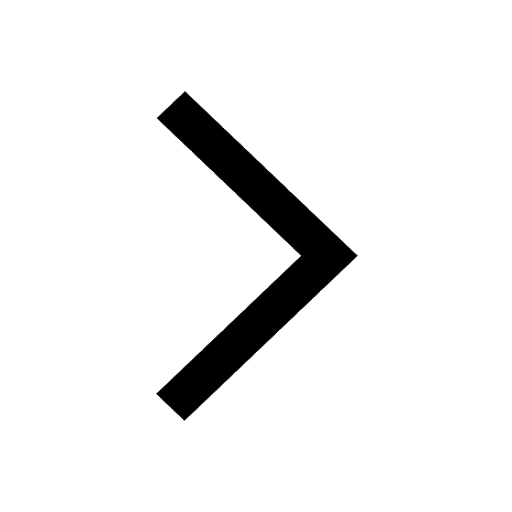
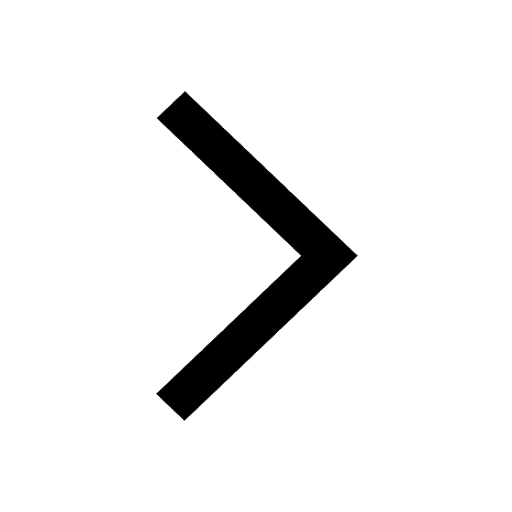
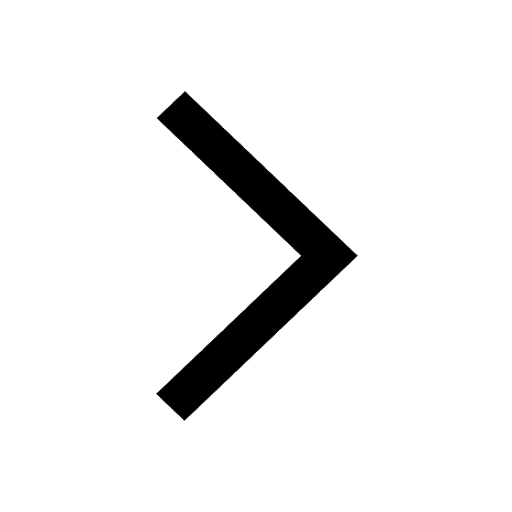
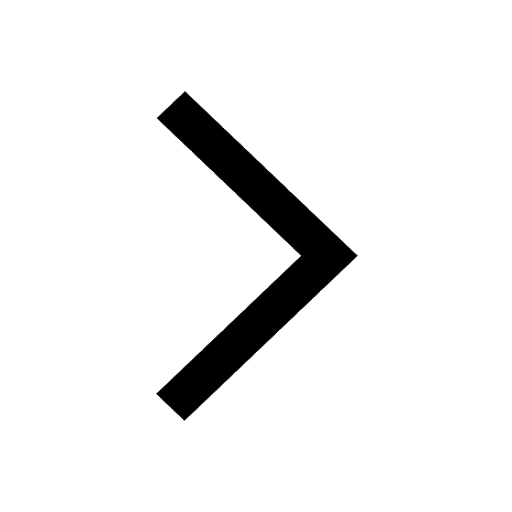
FAQs on Correlation Coefficient
1. What is the Definition of the Term Intraclass Correlation?
Intraclass correlation (ICC) is one of the important descriptive statistical measures that use quantitative measurements from an organized group of units. This is preferred in the applications of finding and explaining how different units of the group are resembling a few similarities to each other.
2. What are the Examples of a Positive Correlation of Coefficient Found in Real-life?
Bodily developments such as weight and height, temperature and the level of water consumption by individuals, sales and marketing changes, the grades of a student and his or her study time, public experience and consumer awareness, are some of the examples of a positive correlation of coefficient found in real-life.
3. Is the Case of a Person Drunk and Driving an Example of a Positive Correlation Coefficient?
No. The case of a person drunk and driving is an example of a negative correlation coefficient since the level of alcohol consumption affects his or her ability, speed, and potential to drive properly.
4. What are the 5 Major Assumptions Under the Linear Regression Model?
The 5 major assumptions under the Linear Regression Model are namely, Errors have a ‘constant variance’, errors posses a ‘mean of zero’, the expected value for the ‘term error will be zero’ (o), errors are generally ‘normally distributed’, and errors are ‘independent’ to each other.
5. State the Homoscedasticity Assumption of Regression.
The Homoscedasticity Assumption of Regression states that every new observation made will possess a variance of error term that is equal and same for all the trials.
6. What is Meant by Regression Analysis?
The mathematical measure of Regression Analysis represents the average relationship or interlinkage between any 2 variables (or even more), concerning the original units derived from the data itself. Multiple Regression and Simple Regression are the 2 classic forms of regression analysis.