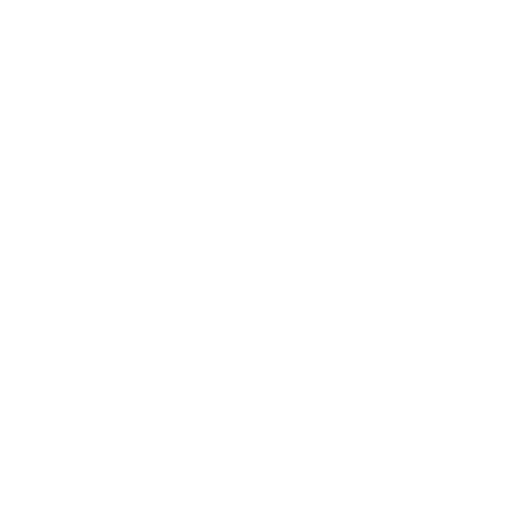

About Standard Deviations
The Standard Deviation is the positive square root of the variance. One of the most basic approaches of Statistical analysis is the Standard Deviation. The Standard Deviation, abbreviated as SD and represented by the letter ", indicates how far a value has varied from the mean value. A low Standard Deviation indicates that the values are close to the mean, whereas a large Standard Deviation indicates that the values are significantly different from the mean. Let's look at how to determine the Standard Deviation of grouped and ungrouped data, as well as the random variable's Standard Deviation.
What is Standard Deviation?
In descriptive Statistics, the Standard Deviation is the degree of dispersion or scatter of data points relative to the mean. It is a measure of the data points' Deviation from the mean and describes how the values are distributed over the data sample. The Standard Deviation of a sample, Statistical population, random variable, data collection, or probability distribution is the square root of the variance.
When we have a certain amount of observations and they are all different, the value's mean Deviation from the mean is then calculated.
On the other hand, the sum of squares of deviations from the mean does not appear to be a reliable measure of dispersion. When the average of the squared differences from the mean is low, the observations are close to the mean. This is a less dispersed level of dispersion. If this number is large, it implies that the observations are dispersed from the mean to a greater extent.
Standard deviation is the measurement of the dispersion of the data set from its mean value. It is always measured in arithmetic value. Standard deviation is always positive and is denoted by σ (sigma). Standard Deviation is very accurate and is preferred from other measures of dispersion.
The Standard Deviation is calculated as The square root of variance by determining each data point's deviation relative to the arithmetic mean. In case the data-points are far from the mean, it denotes a higher deviation within the set of data. Hence, it indicates more spread out the data, the higher is the standard deviation. The formula to calculate Standard Deviation is:
s = \[\sqrt{\frac{\sum (x_{i}-\overline{x})^{2}}{n-1}}\]
where:
x(i) = value of the i’th point in the set of data
x(bar) = the mean-value of the set of data
n = the number of data-points in the set of data
(Image to be added soon)
Properties of Standard Deviation
Standard Deviation is only used in measuring dispersion or spread around the mean value of the data set.
Standard deviation is always in positive value.
It determines the dispersion or variation that exists from the average value.
Standard deviation is a very sensitive outlier. Any single outlier can distort the picture of dispersion.
For the data set with an approximately same mean value, the greater the dispersion or spread, the greater the Standard deviation.
Standard deviation is zero when the values of a particular data set are the same.
While analyzing the normally distributed data, the Standard Deviation is used in conjunction along with the mean to calculate the data intervals.
If \[\overline{x}\] = mean, S = Standard Deviation, and x = Value in the Data set, then
around 68% of the Data is in the interval:- \[\overline{x}\] - S < x < mean + S.
around 95% of the Data is in the interval:- \[\overline{x}\] - 2S < x <mean + 2S.
around 99% of the Data is in the interval:- \[\overline{x}\] - 3S < x < mean + 3S.
(Image to be added soon)
Standard Deviation Calculation
Before calculating the Standard Deviation, it is essential to underline the three types of data distribution. These are:
Individual Series
A single column denoting the observation is available here.
Discrete Series
Two columns represent different data. One column shows the observation, while the other column is for frequency corresponding to the observation column.
Frequency Distribution
It has two columns, one representing the observations, and the other is corresponding frequency.
Here the observations are classified further into intervals or classes.
Sigma for Individual Series
Three methods can calculate the Standard deviation for individual series; these are:
A Direct Method to Calculate Standard Deviation
Use the formula ∑X/N to calculate the arithmetic mean. After this, we calculate the deviations of all the observations from the mean value using the formula D= X-mean.
Now, the deviations, x, are squared and summed. The resultant value is then divided by the total number of observations. The square root of the above-derived value = Standard deviation
The formula is - σ = √[∑D²/N]
Here, D = deviation of an item that is relative to mean. It is calculated as D = X- mean.
N = Number of observations
Short-Cut Method
In this method, any random value is assumed to calculate deviation. It is believed that the assumed value is in the Middle of the Range of Values. The short cut method is derived using the formula;
σ = √[(∑D²/N) – (∑D/N)²]
Step-Deviation Method
It is a simple form of the short-cut method. Here, we select a common factor C, among the deviations. All the deviation values reduce when divided by C, simplifying the calculations. The formula is;
Standard deviation D (σ)= √[(∑D’²/N) – (∑D’/N)²] × C
D'= step-deviation of Observations relative to an Assumed mean. It is calculated as D'= (X-A)/C
C= Common Factor chosen.
Sigma for Discrete Series
There are two ways to calculate Standard Deviation in discrete series, theses are:
Direct Method
We know that in the discrete series, another frequency column is added; the direct method formula to calculate SD is:
Standard deviation (σ) = √(∑fD²)/N)
Short-Cut Method
Standard deviation (σ) = √[(∑fD²/N) – (∑fD/N)²]
Sigma for Frequency Distribution
Three different methods can be used to calculate standard deviation in frequency distribution series; these methods are:
Direct Method
The direct method employed to derive standard deviation in a frequency distribution is very similar to the discrete series done above. The value of observation (when used) in the frequency distribution is the only difference between the two series. Here, the mid-value of the class is determined by dividing the sum' of the upper value of the class and the lower value of the class. The value thus derived is used for calculation. The formula is;
Standard Deviation (σ) = √(∑fD²)/N)
In the calculation, D = Deviation of an item that is relative to mean value and is calculated as,
D = Xi – Mean
F = frequencies corresponding to the Observations
N = The summation of the frequency.
Step-Deviation Method
The step-deviation method is the shortcut method to determine the Standard Deviation. The formula is
Standard Deviation (σ) = √[(∑fD’²/N) – (∑fD’/N)²] × C
In the above calculation, D'= Step-Deviation of the observations relative to the assumed value. It is calculated as- D'= (Xi-A)/C
N = The Summation of Frequency.
C = Common Factor chosen
Did You Know?
Without Standard Deviation D, one can't compare two sets of data effectively. Suppose there are two data sets having the same average. Does that imply that the sets of data are exactly the same? No. For ex. the data sets - 199, 200, 201, and other 0, 200, 400 have the same 200 average, yet they have different standard deviations. Here, the first data has a small standard deviation (s=1) in comparison to the second set of data (s=200).
FAQs on Standard Deviation: Calculation and Applications
1. What does SD or Standard Deviation indicate?
The standard deviation (SD) indicates the amount of variability on an average in your set of data. On an average, it tells us how far each score is available from the mean value. The Standard Deviation is the most accurate measurement compared to other dispersion measures available and can never be negative. The symbol Sigma or σ denotes standard deviation.
In normal distributions, a higher standard deviation implies that the values are further away from the mean. Similarly, a lower standard deviation means the values are clustered very close to arithmetic mean value.
2. What is the difference between the variance and the Standard Deviation?
The difference between the standard deviation and the variance is as follows:
Variance means the average squared deviations that are measured from the mean, whereas Standard Deviation is calculated as the Square root of this number. Although both the measurements indicate variability in the distribution, however, their units differ:
The standard deviation (SD) is expressed as the same unit that is available in the original value (example - meters, grams, or minutes)
The variance is denoted in larger units in comparison, such as a square meter.
Although the units measured of variance are a little difficult to understand initially, the variance is significant in the statistical test.
3. How is Standard Deviation calculated?
The following formula is used to compute the Standard Deviation:
By adding all of the data points and dividing by the number of data points, the mean value is calculated.
Each data point's variance is calculated by subtracting the mean from the data point's value. After that, each of the resulting values is squared, and the results are added together. The result is then divided by the number of data points divided by one.
The square root of the variance is used to determine the Standard Deviation.
4. What makes the Standard Deviation such an effective measure of variability?
Although there are simpler techniques to determine variability, the Standard Deviation formula favours samples that are unevenly distributed over those that are uniformly distributed. A higher Standard Deviation indicates that the distribution is not just wider, but also more unevenly distributed.
This implies it provides a more accurate picture of the variability in your data than simpler measurements like the mean absolute Deviation (MAD).
The MAD is similar to the Standard Deviation but is less difficult to compute. To begin, turn each departure from the mean into positive numbers and represent them as absolute values (for example, -3 becomes 3). The mean of these absolute variances is then calculated.
Unlike the Standard Deviation, the MAD does not require you to calculate squares or square roots of numbers. As a result, it provides you with a less exact estimate of variability.
5. What is a standard error?
When conducting research, it's common to only collect data from a tiny portion of the population. As a result, you're likely to get somewhat different sets of values each time, with slightly different means.
When enough samples are taken from a population, the means form a distribution around the true population mean. The Standard error is the Standard Deviation of this distribution, i.e. the Standard Deviation of sample means.
The Standard error indicates how close any particular sample of that population's mean is likely to be to the genuine population mean. When the Standard error rises, implying that the means are more evenly distributed, it becomes more likely that any given mean is a poor representation of the genuine population means.
6. What are the merits and demerits of Standard Deviation?
The Standard Deviation has the following advantages:
1. It can be calculated analytically and is therefore reliable. As a result, it is used in high-quality research.
2. It is based on every piece of data in a series.
3. It is unaffected by any form of unexpected Deviation.
4. It is a precise indicator of dispersion.
Standard Deviation has the following disadvantages:
1. Its computation method is difficult and inconvenient.
2. Higher values are given greater weight in this metric than lower ones, which affect the S.D. value.
7. What are different methods of Standard Deviation?
The methods are-
Method of step-Deviation
The shortcut method is an expansion of the step Deviation method. Furthermore, it simplifies the shortcut technique by choosing a common factor across Deviations that reduce all Deviation values when divided by this factor. As a result, the calculation is simplified as a result of this decrease.
Short cut method
This method is based on the notion that any random value can be used to calculate Deviation. Because choosing an extreme value would result in substantial Deviations and hence make calculations tiresome, the value is usually considered to be in the middle of the range of values.
Direct method
The arithmetic mean is initially determined using the formula X/N in this manner. Then, using D= X-mean, the Deviations of all observations from this mean value are determined. These variances, x, are then squared and their sum divided by the number of observations in the next step. Finally, the square root of the previous calculation yields the Standard Deviation.
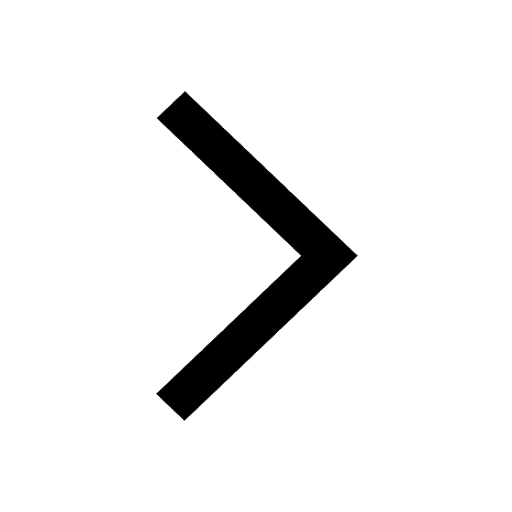
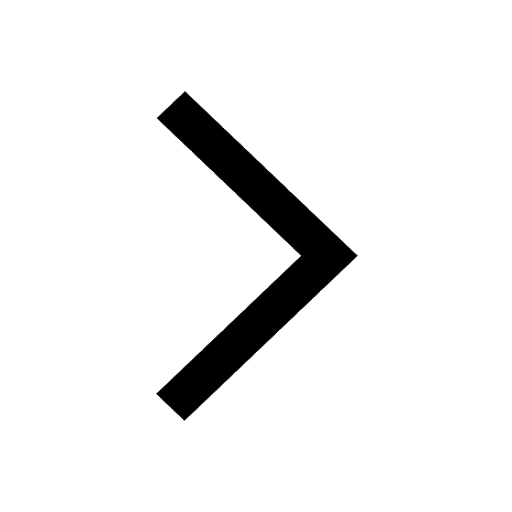
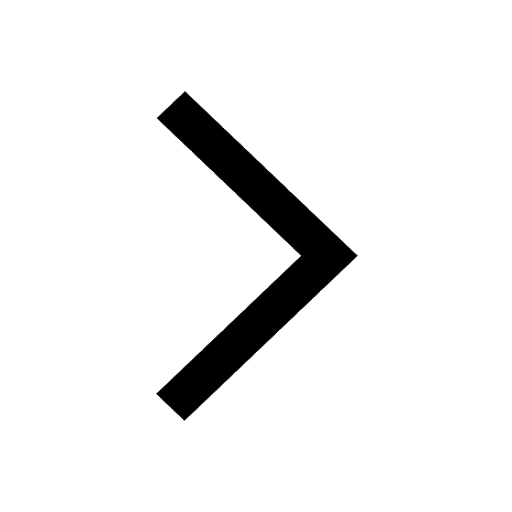
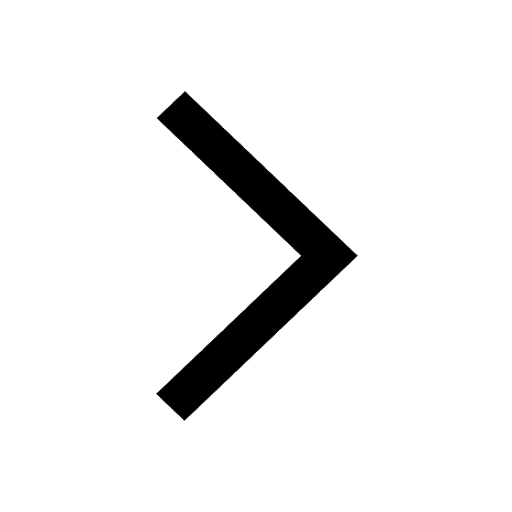
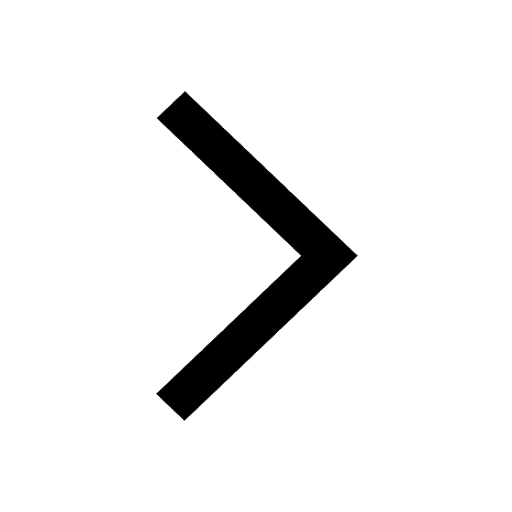
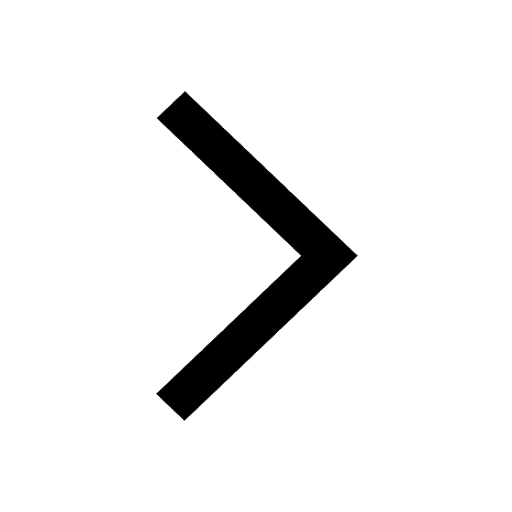