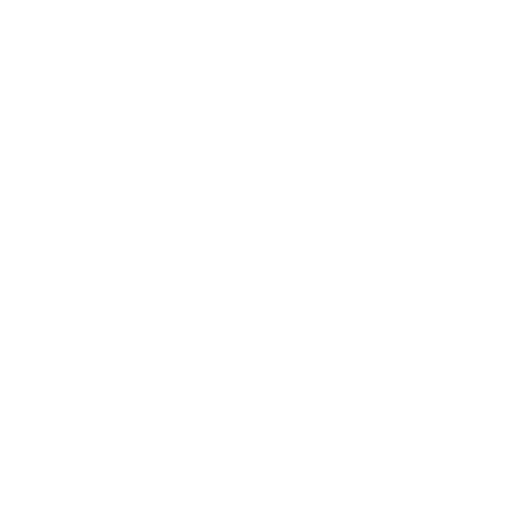

What is a Non Parametric Test?
Non parametric tests are mathematical methods that are used in statistical hypothesis testing. This method is used when the data are skewed and the assumptions for the underlying population is not required therefore it is also referred to as distribution-free tests. In other words, if the given population is uncertain or when the data are not distributed normally, nonparametric tests are used. The word nonparametric method does not indicate that there are absolutely no parameters but it tells us that the characteristics and number of parameters are not predefined but flexible. Usually, we use a nonparametric test when we have noncontinuous data which has a large sample size.
Parametric Test and Nonparametric Test
The only difference between parametric test and the nonparametric test is that parametric test assumes the underlying statistical distributions in the data whereas nonparametric tests do not rely on any distribution. In a parametric test, there are several conditions of validity that must be met to make the result reliable whereas nonparametric tests can be applied even if parametric conditions of validity are not met.
Non Parametric Test Formula
In Kruskal-Wallis H-Test, we use a formula to calculate the results. The formula can be written as:
H =
For more information on the formula download non parametric test pdf or nonparametric test ppt
Types of Non Parametric Test
When we talk about parametric in stats, we usually mean tests like ANOVA or a t test as both of the tests assume the population data to be a normal distribution. But this is not the same with non parametric tests. Non parametric tests do not take the data to be normally distributed. The only non parametric test in the elementary stats is the chi-square test. However, there are different types of non parametric tests such as the Kruskal Willis test which is a non parametric alternative to the One way ANOVA and the Mann Whitney which is also a non parametric alternative to the two sample t test. We have listed below a few main types of non parametric tests.
1-sample Sign Test: This test is used to estimate the median of a population followed by comparing it to a reference value or target value.
1-sample Wilcoxon Signed Rank Test: This test is the same as the previous test except that the data is assumed to come from a symmetric distribution.
Friedman Test: Friedman tests examine the difference between groups with ordinal and dependent variables.
Kruskal-Wallis Test: this test finds out if two or more medians are different. The ranks of the data points are utilized for the calculations, rather than the data points themselves.
The Mann-Kendall Trend Test: This test checks the trends in time-series data.
Mann-Whitney Test: This test judges the differences between two independent groups on a condition that the dependent variables will either be ordinal or continuous.
Mood’s Median Test: This test is used instead of the sign test when we have two independent samples.
Spearman Rank Correlation: We use this test to find the correlation between two sets of data.
Non Parametric Test Advantages and Disadvantages
There are few nonparametric test advantages and disadvantages.Some of the advantages of non parametric test are listed below:
The basic advantage of nonparametric tests is that they will have more statistical power if the assumptions for the parametric tests have been violated.
There are many assumptions in the nonparametric tests as compared to parametric tests.
Nonparametric tests include short calculations which are easily understandable.
It is applicable to all types of data such as nominal variables, interval variables etc irrespective of small sample sizes or large sample sizes.
The Disadvantages of Non Parametric Test are as follows
The basic disadvantages of non parametric test inon parametric tests are less powerful than parametric tests if the assumptions haven’t been violated.
In non parametric tests, calculation by hand becomes tough.
Computer software packages do not include critical value tables for many non parametric tests.
The results of non parametric tests may or may not come true as it is based on distribution free data
Hypothesis Significance
Hypothesis is a process to assess the significance of the difference between two samples or two items whether they are really significant or not. The test of hypothesis can be laid in various fields. Hypothesis testing is carried not only in statistics but also in other sectors like for deriving population samples for childbirth or or even for testing a program whether it is effective or not. If the sample shows very small difference the hypothesized value is correct and if the statistical test shows the difference is significant the hypothesis gets rejected. There are two types of hypothesis in statistics -null hypothesis and alternative hypothesis.
Hypothesis Testing
In hypothesis testing, statistical tests help to determine if the null hypothesis is rejected or not. These statistical tests begin with the null hypothesis that there are no relationships or differences between groups.
This article describes statistical tests for hypothesis tests, including both parametric and nonparametric tests.
Table of Contents
What are Parametric Tests?
What are Non-parametric Tests?
Parametric Tests for Hypothesis testing
T-test
Z-test
F-test
ANOVA
Non-parametric Tests for Hypothesis testing
Chi-square
Mann-Whitney U-test
Kruskal-Wallis H-test
Parametric Tests
In parametric tests, we have a fixed set of parameters that are used to found a probabilistic model that can be used in machine learning. Parametric tests are the tests for which we have proper information of the population distribution, or if not then we can take the approximate value which is possible with the help of the Central Limit Theorem.
Parameters for using the normal distribution include Mean and Standard deviation.
(Image will be uploaded soon)
Thus, the classification of a test to be parametric is dependent on the population assumptions.
Non-Parametric tests in Statistics
In non-parametric tests we do not make any assumptions about the parameters for the given population. Thus, there is no fixed set of parameters available, and also there is no distribution (normal distribution, etc.) of any kind available for use.
This is also the reason that the nonparametric tests are also referred to as distribution-free tests as they don't have fixed parameters to be followed.
Chi-square
Mann-Whitney U-test
Kruskal-Wallis H-test
Applications of Non-Parametric Test
The conditions in which non-parametric tests can be used are:
When parametric tests do not give satisfactory results
When hypothesis is tested without distribution
For quick data analysis.
When unscaled data is available.
FAQs on Non Parametric Test – Formula and Types
1. Where can we use the chi-square test?
Chi-square can be used as a non-parametric test:
Test of goodness
As a test of independence of two variables
2. What are the conditions for the chi-square test?
Following are the conditions for chi square test
Randomly collect and record the Observations.
In the sample, all the elements should be independent.
None groups should contain a very few items
The reasonably large overall number of items.
3. What is the U- test also called?
It is also known as:
Mann-Whitney Wilcoxon Test.
Mann-Whitney Wilcoxon Rank Test.
4. What is the common application of the chi-square test?
As an alternative test to find the significance of the difference in two or more than two proportions
To compare the values of two binomial samples if they are small
To compare the frequencies of two multinomial samples
As a test of association between two events in Binomial or multinomial samples
As a test of goodness of fit.
5. What are the Advantages and Disadvantages of Non-Parametric Tests?
They can be understood easily
They have small calculations
Assumption of distribution is not needed
It is applicable to all kinds of data
The disadvantages of the non-parametric test include the following:
It is less efficient than the parametric test
The results may or may not give an accurate answer because they are distribution free.
6. What are the applications of non parametric tests?
Non parametric tests are used when the data fails to satisfy the conditions that are needed to be met by parametric statistical tests. Non parametric tests are also very useful for a variety of hydrogeological problems. The non parametric tests such as Kruskal‐Wallis and Mann‐Whitney are the two tests that are used to judge the difference between two medians or two independent groups on a condition that the dependent variables will either be ordinal or continuous. Apart from this, non parametric tests are also used for quick data analysis, for testing hypotheses when there is no distribution available. It is also used when we have unscaled data.
7. Is it a chi square test? List the basic assumptions of the chi square test?
In statistics, Chi Square tests are usually used for testing relationships between categorical variables. In a Chi Square test, a null hypothesis results in no relationship on the categorical variables in the population; they are alway independent. The basic Chi square assumptions are:
The data in the Chi Square cell must be a frequency instead of percentages.
The categories or the variables must be mutually exclusive.
The subject must contribute the data to only one cell in the x2
For two different groups that are related, we need to use two different tests. Therefore, the study groups must be independent.
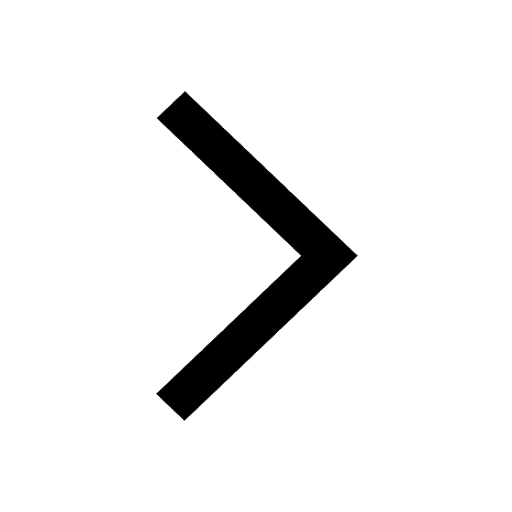
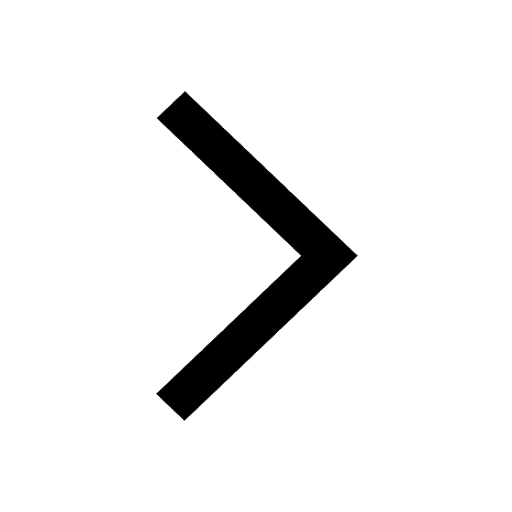
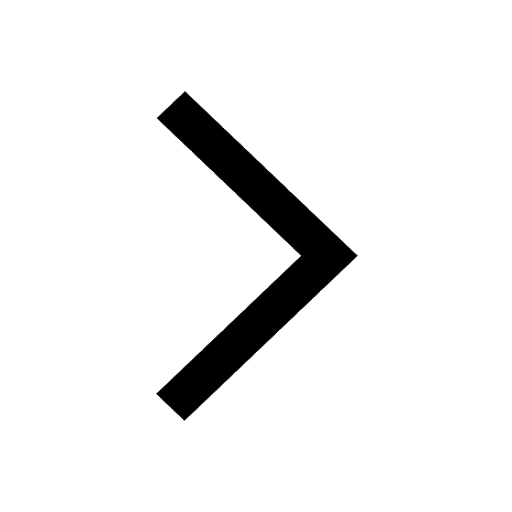
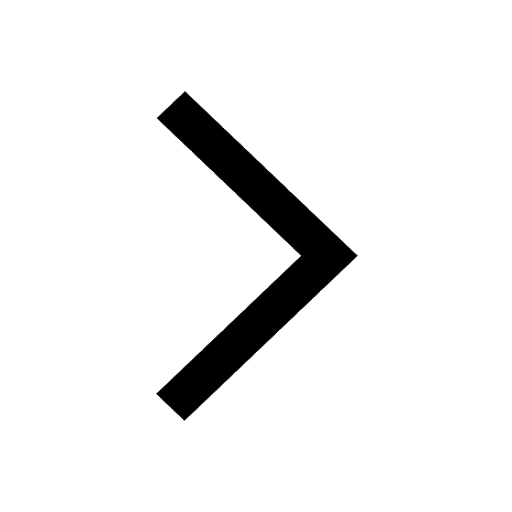
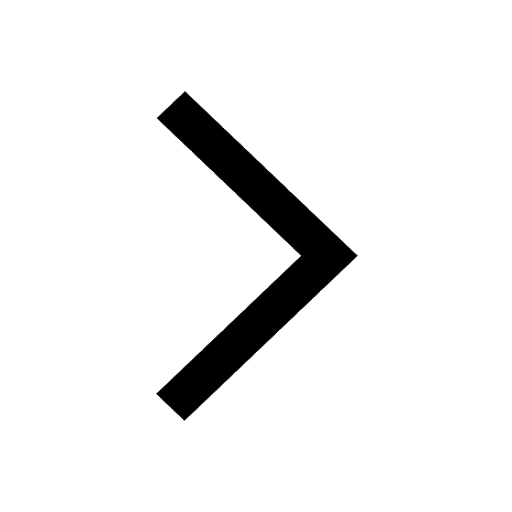
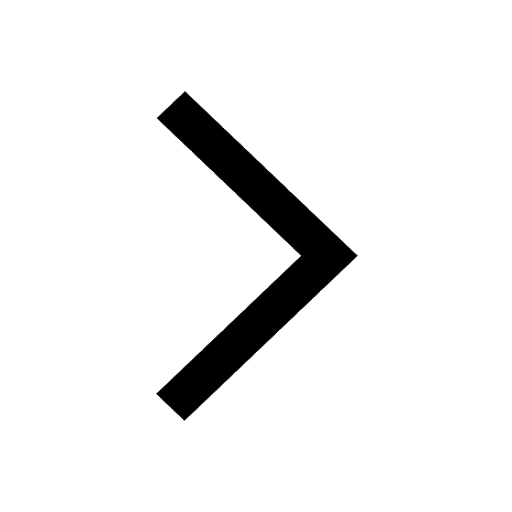
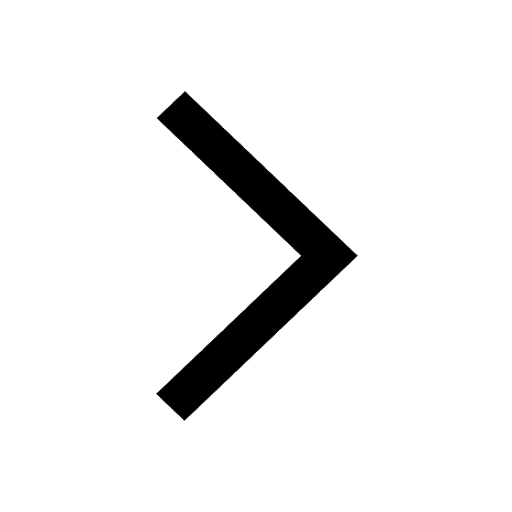
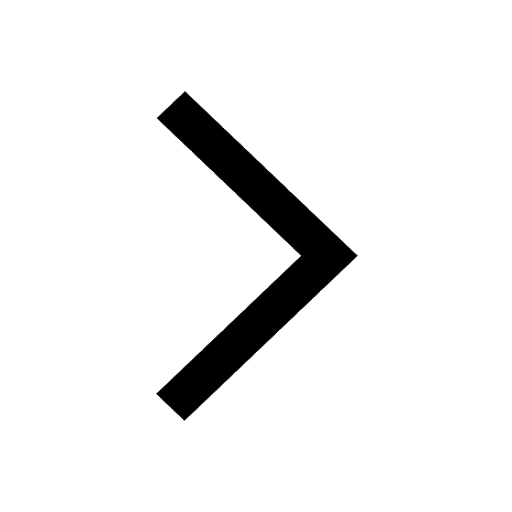
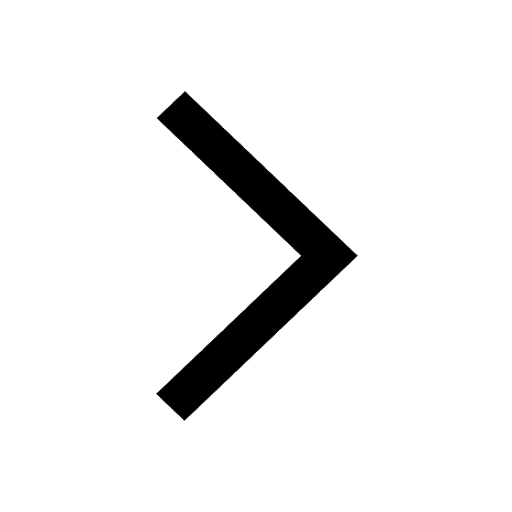
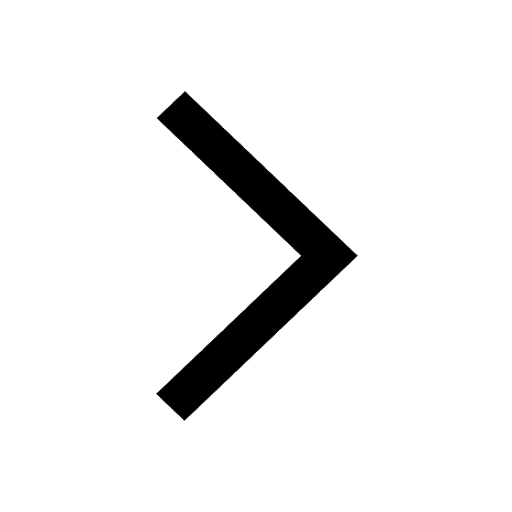
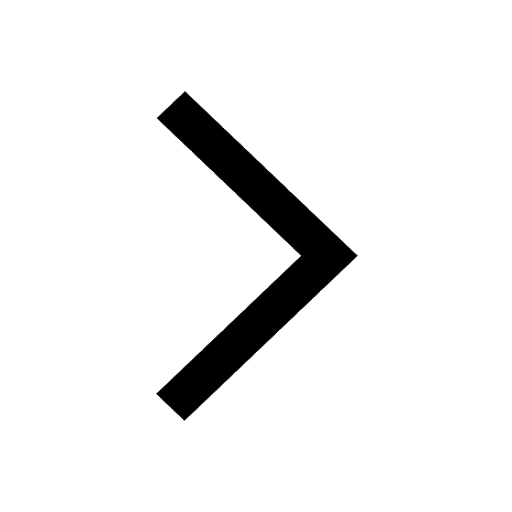
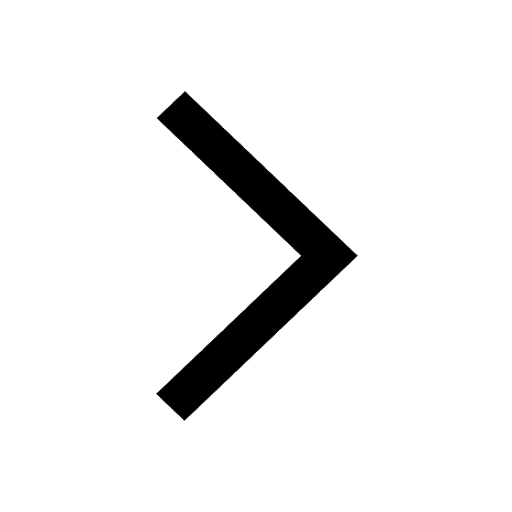
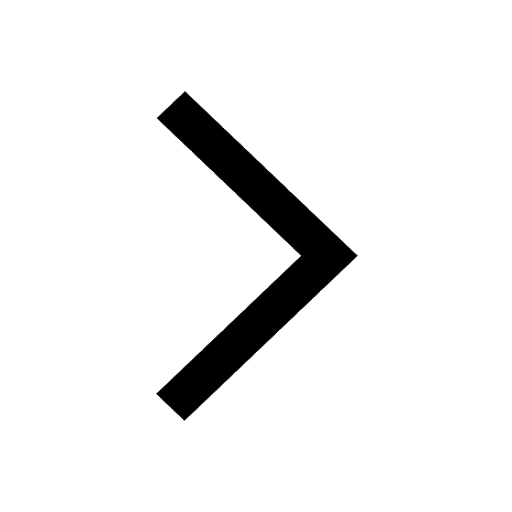
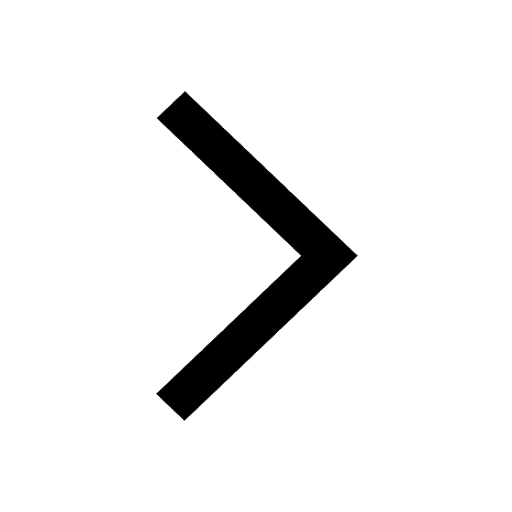
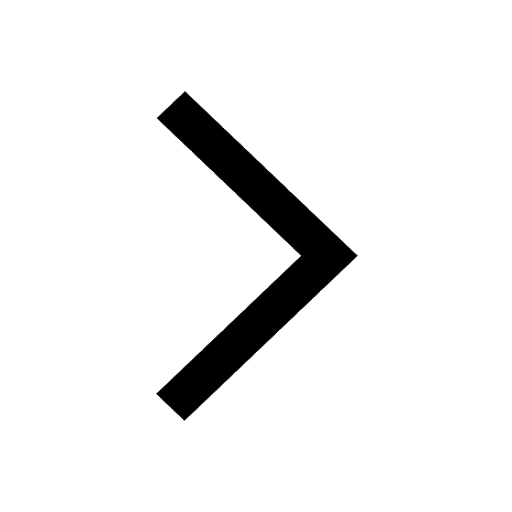
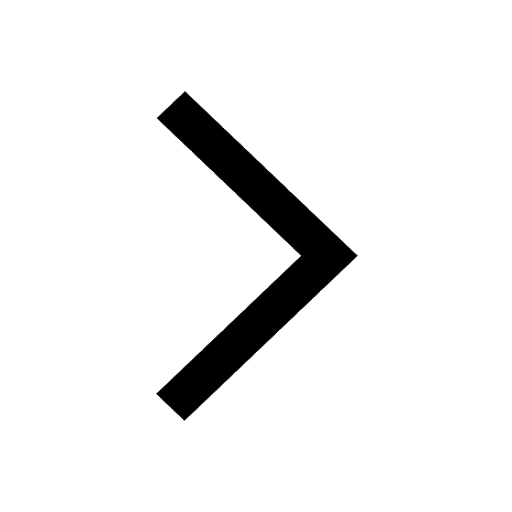
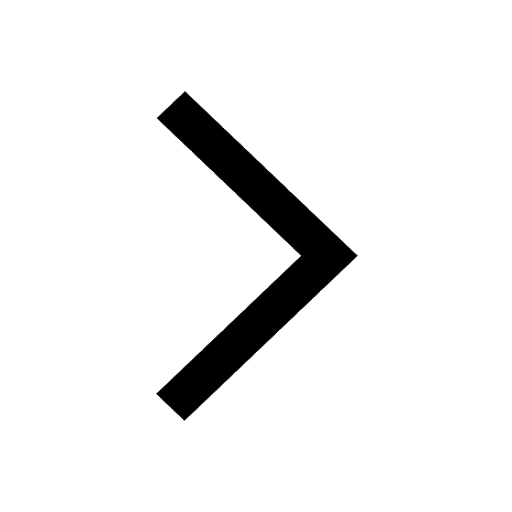
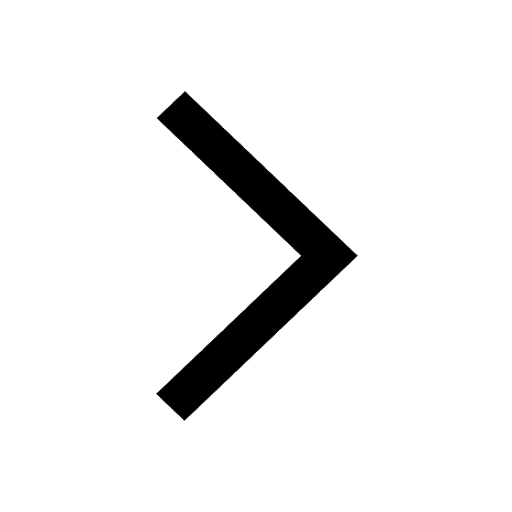