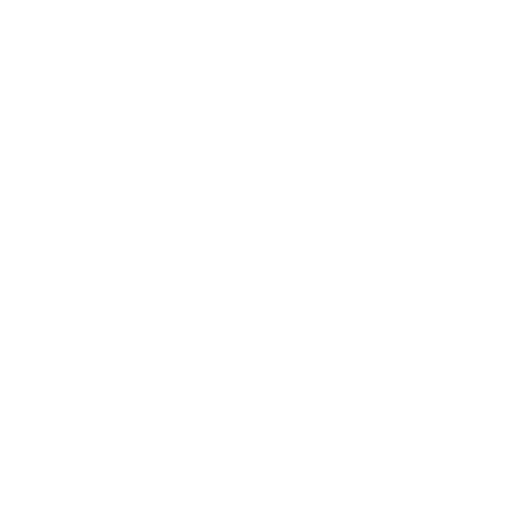
What is Mean Square Error?
A mean-squared error is an essential part of the estimation of the statistics. It helps in measuring the discrepancies between the estimated value and the actual value giving insight through its unique formula to calculate the square of the errors and their average. The square and the average gives the value of the MSE.
The calculation of errors is based on the difference between the value being estimated and the estimated value. As it forecasts the value of the loss, it is a risk function. This quantification helps in analyzing the reason for the loss that can be the estimator as well. MSE is mostly positive.
There are other ways to calculate what is MSE. For instance, if the estimator is not biased, then the variance and the mean-squared error is the same. The unit varies according to the primary measure of the quantity.
Mean Square Error Formula
The MSE formula is pretty easy to understand.
MSE = (1/n)\[\sum_{i=1}^{n}\] (Xobs,i -Xmodel,i)²
The summation sign denotes the sum of all the points that are being considered to estimate the error. Starting from the first point to the nth point or the last point of estimation, the sum has to be calculated. Xi represents all the true values meaning that the obs and the model value represent the actual and predicted values, respectively.
The MSE Formula can also be seen in the light of a specific value, which can be estimated with the same formula replacing the obs value with it and the actual value with the model value.
When the mean square formula is used concerning a regression line on the graph, the Xobs, i value is replaced by the y coordinate of the points from which the errors are to be estimated. The Xmodel, i is taken from the regression line, and in this way, the formula is solved.
What is the Root Mean Square Error Formula?
The confusion of root mean square error vs. mean square error can make the task of finding the estimate difficult. So it is better to analyze what root mean square error formula is for better understanding. The RMSE formula, in simple words, would be the root of the MSE formula.
The primary use of the formula is to understand the magnitude of errors between the predicted and actual values. One of the major differences between MSE and RMSE is that in RMSE, the formula is used for only specific variables but not a range of variables. This is not true for the mean square error formula as root mean square errors are scale-dependent. The formula for
RMSE = \[\sqrt{1/n}\] \[\sum_{i=1}^{n}\] (Xobs,i -Xmodel,i)²
Root Mean Square Error:
This formula is responsible for understanding the accuracy of the model better. Understanding root mean square error vs. mean square error will clear the conjectures relating to what is MSE.
Steps to Use the Formula (with Graphs)
On a graph, the MSE can be seen as the distance between the regression line and the set of points given. In this case, understanding the formula is easier. Therefore, let’s understand how to look at the MSE equation from this perspective.
The reference point for the calculation is the regression line. It has to be calculated to go ahead with the other steps using the coordinates mentioned and the line equation.
Find the new value of the Y’s using the X values to find the exact position of the regression line.
The new Y values are to be subtracted from the actual values to find the error.
Use the formula to calculate the sum and squares of the errors and find the mean.
The example given below would help to understand better.
Solved Example
Q. Find the Mean Squared Error or mse Equation for the Following Set of Values: (43,41),(44,45),(45,49),(46,47),(47,44)
A. On calculating the regression line using an online computation tool, it is found to be y= 9.2 + 0.8x.
The new Y’ values are as follows:
9.2 + 0.8(43) = 43.6
9.2 + 0.8(44) = 44.4
9.2 + 0.8(45) = 45.2
9.2 + 0.8(46) = 46
9.2 + 0.8(47) = 46.8
The error can be calculated as (Y-Y’):
41 – 43.6 = -2.6
45 – 44.4 = 0.6
49 – 45.2 = 3.8
47 – 46 = 1
44 – 46.8 = -2.8
Adding the squares of the errors: 6.76 + 0.36 + 14.44 + 1 + 7.84 = 30.4. The mean of the squares of the errors are: 30.4 / 5 = 6.08
Regression line =
y=9.2+0.8x
Facts about Mean Squared Error
The efficacy depends on the proximity of the value to zero.
The ideal value for MSE is zero. However, that is not very probable.
Score functions like Brier score are used in forecasting, and further prediction is based on what is mean square error.
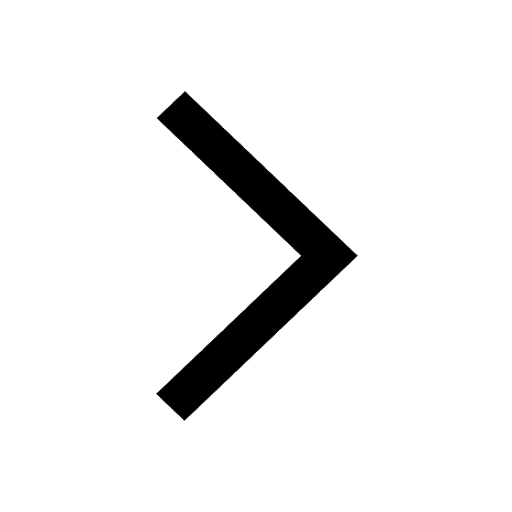
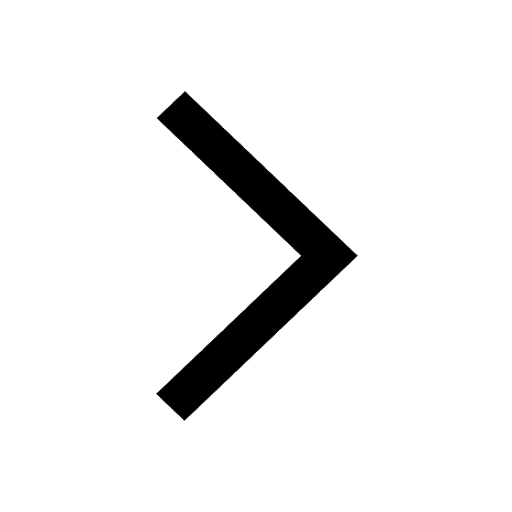
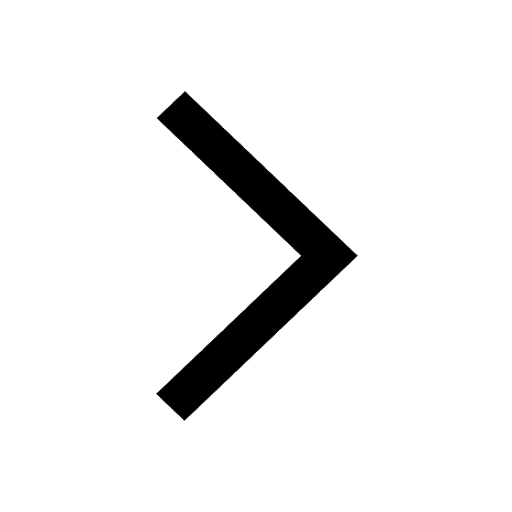
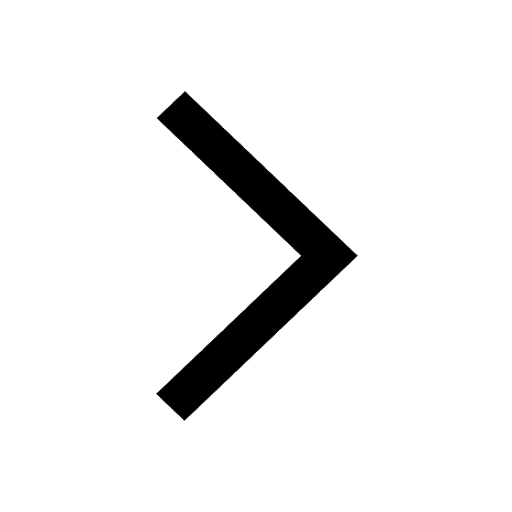
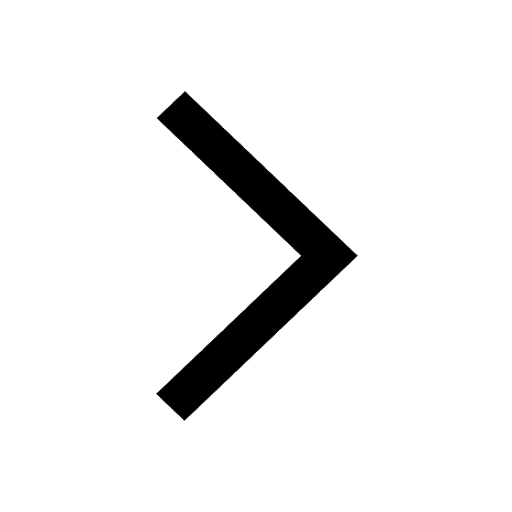
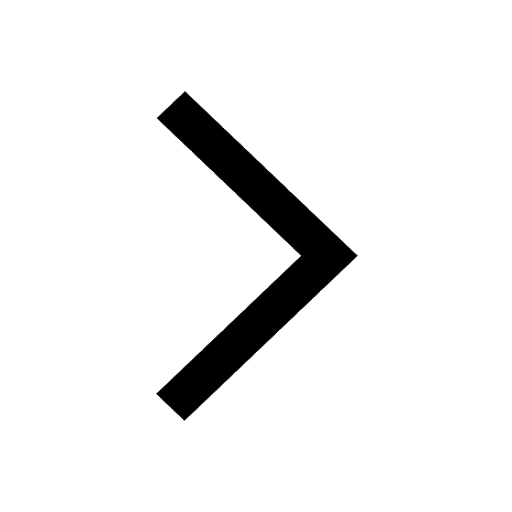
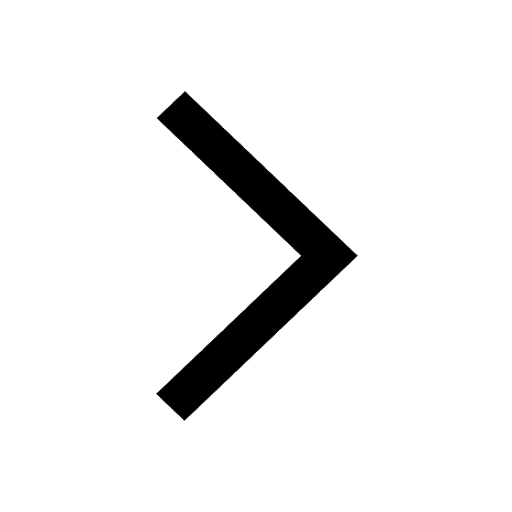
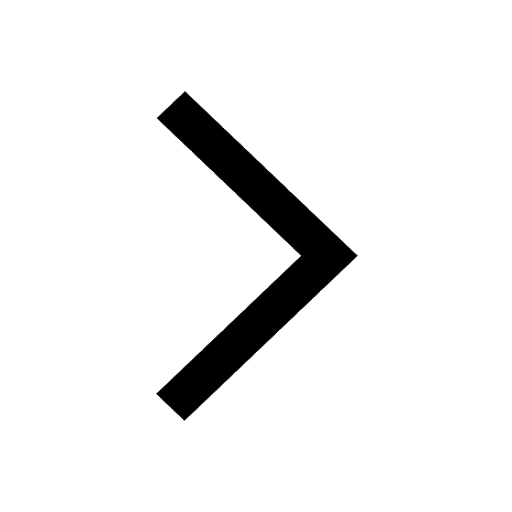
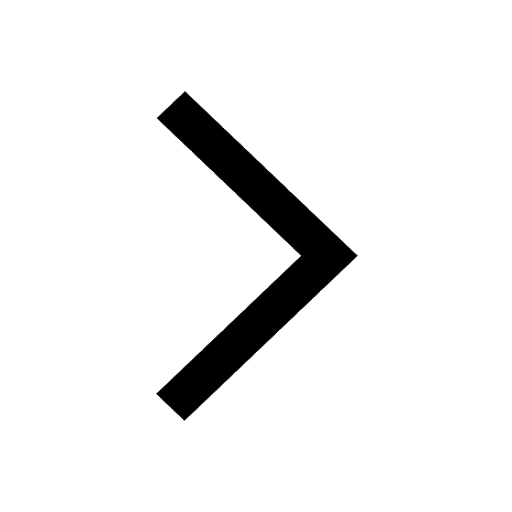
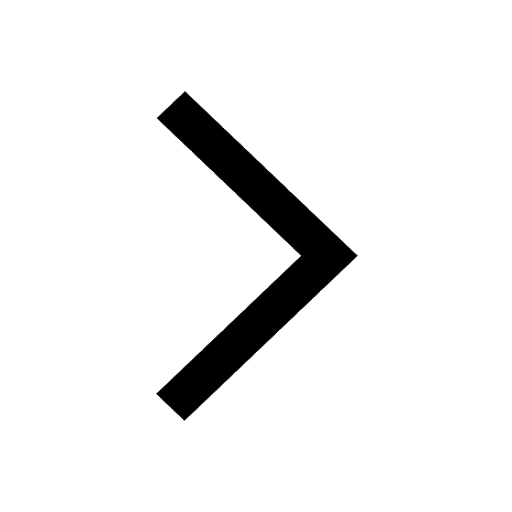
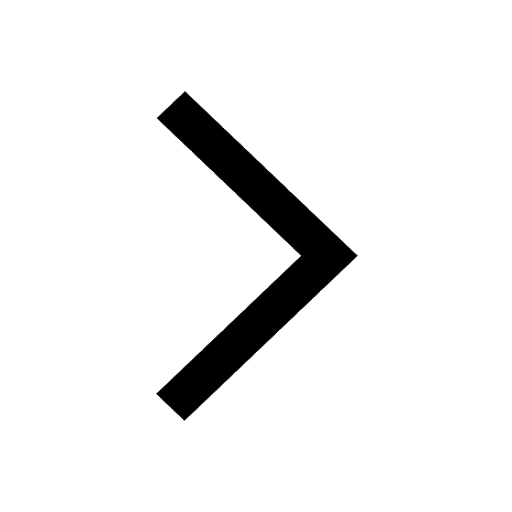
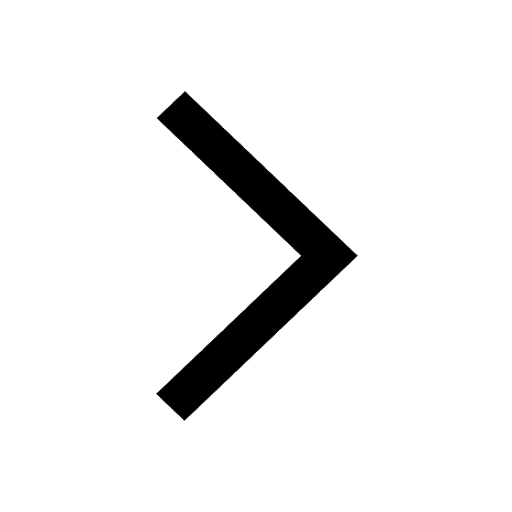
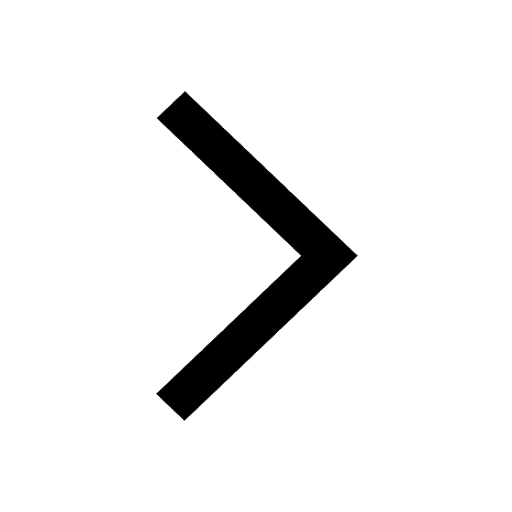
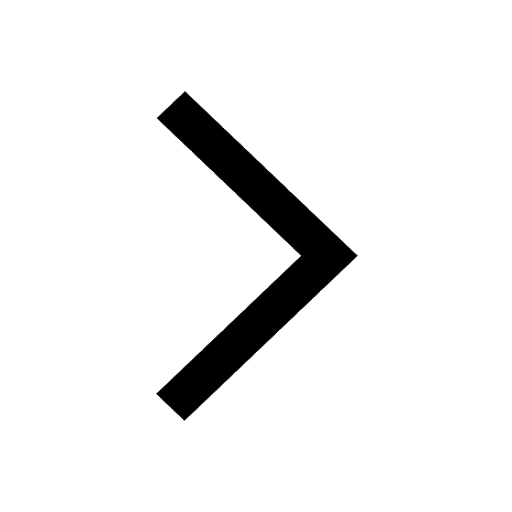
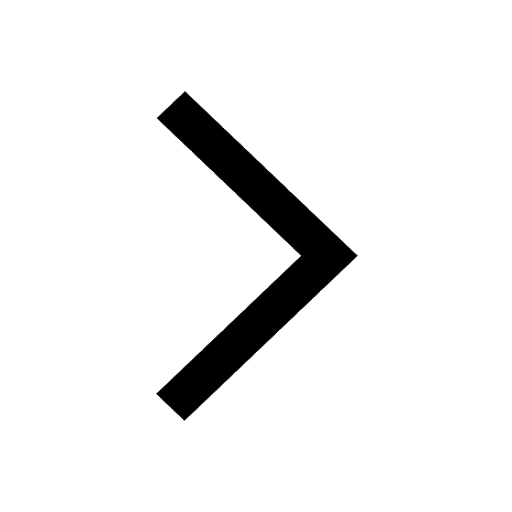
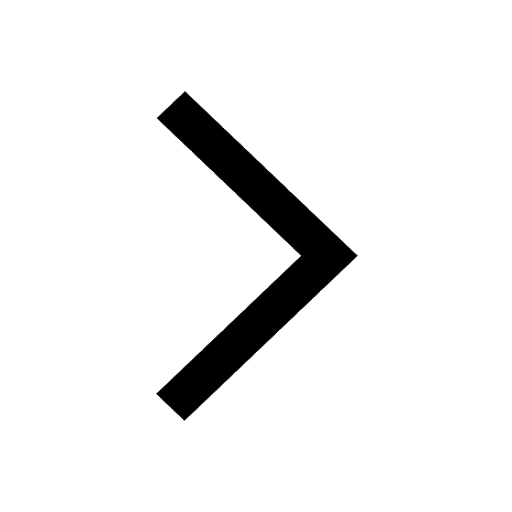
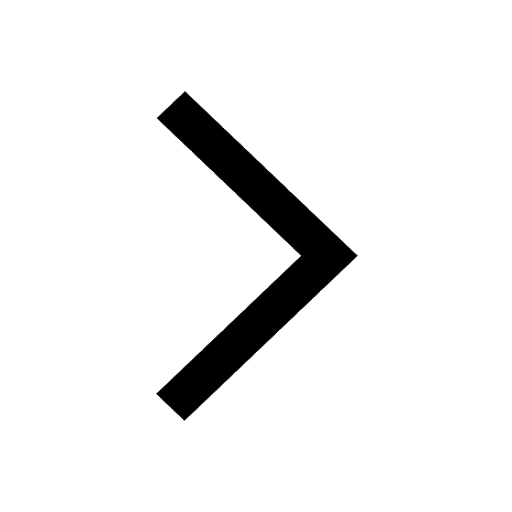
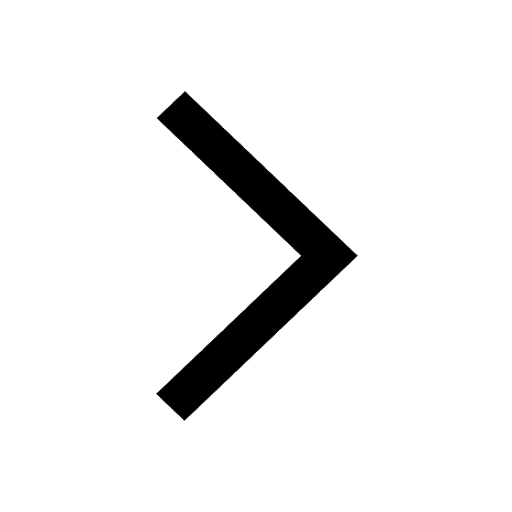
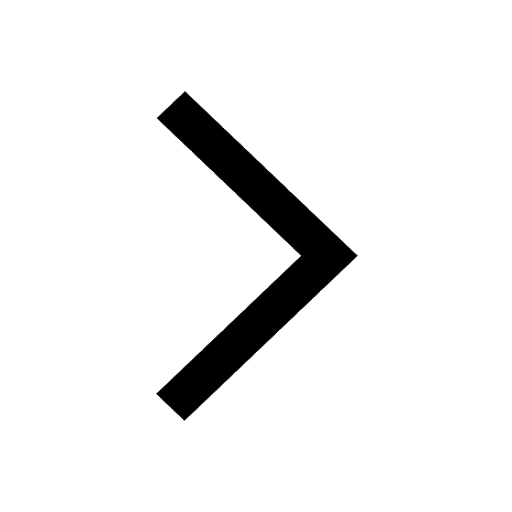
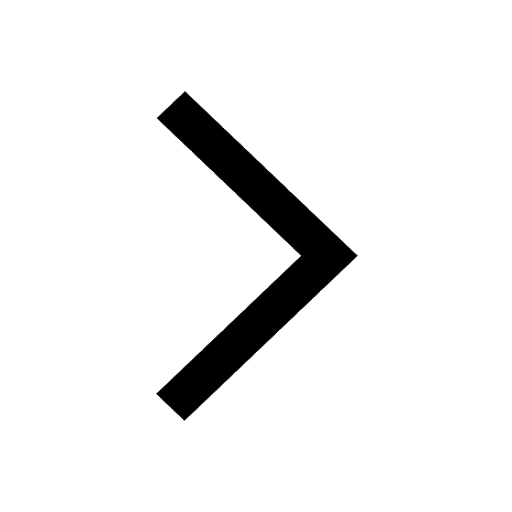
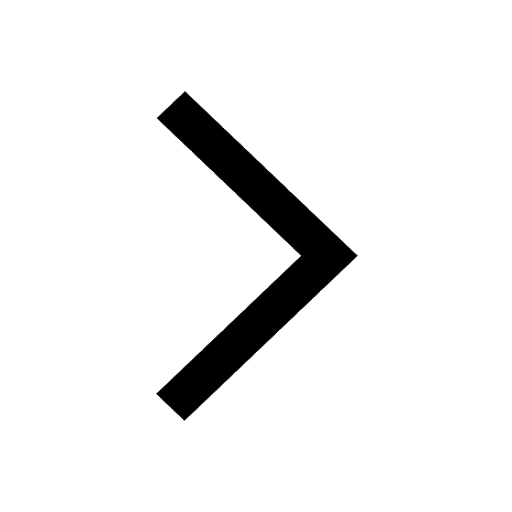
FAQs on Mean Squared Error
1. Why are MSEs Never Negative?
Mean Squared Error is not just only never negative but is also always positive. The reason behind this is the randomness of the errors. It can also happen because of the lack of information about estimators. Since MSE is also the indicator of the quality of the points as they are responsible for understanding the errors based on their distance from the regression line. Also, if you look at the formula carefully, the errors are squared. As a result all negative signs also become positive. Though values that are closer to zero are considered to be better in this regard as they represent less loss and errors, the MSE values are always positive and never zero or negative.
2. How is MSE Used in Forecasting?
Since the values are estimated, Mean Square Errors are responsible for showing errors and also put great emphasis on the large errors that can make a great impact. Therefore, since MSEs are focused on future predictions, they can help in decision making through estimations. Since the errors are squared and are always positive, they provide the largest error score possible from the given coordinates. This makes it possible to know the worst possible forecasts aiding in contingency management. If there is no error, the mean squared error is zero which makes it an ideal situation. Though it is highly improbable to find it, this method gives pretty accurate outcomes that can be relied upon for forecasting.