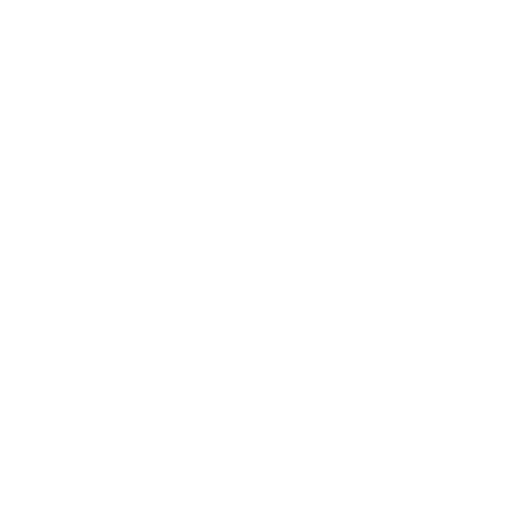

Exponential Distribution can be defined as the continuous probability distribution that is generally used to record the expected time between occurring events. It is also known as the negative exponential distribution, because of its relationship to the Poisson process. An exponential distribution example could be that of the measurement of radioactive decay of elements in Physics, or the period (starting from now) until an earthquake takes place can also be expressed in an exponential distribution.
For any event where the answer to reliability questions aren't known, in such cases, the elapsed time can be considered as a variable with random numbers. As long as the event keeps happening continuously at a fixed rate, the variable shall go through an exponential distribution.
It can be expressed in the mathematical terms as:
\[f_{X}(x) = \left\{\begin{matrix} \lambda \; e^{-\lambda x} & x>0\\ 0& otherwise \end{matrix}\right.\]
where e represents a natural number
λ = mean time between the events, also known as the rate parameter and is λ > 0
x = random variable
Exponential Probability Distribution Function
The exponential Probability density function of the random variable can also be defined as:
\[f_{x}(x)\] = \[\lambda e^{-\lambda x}\mu(x)\]
Exponential Distribution Graph
(Image to be added soon)
The above graph depicts the probability density function in terms of distance or amount of time difference between the occurrence of two events. The terms, lambda (λ) and x define the events per unit time and time respectively, and when λ=1 and λ=2, the graph depicts both the distribution in separate lines.
Cumulative Distribution Function of Exponential Distribution
Taking from the previous probability distribution function:
\[f_{x}(x)\] = \[\lambda e^{-\lambda x}\mu(x)\]
Forx \[\geq\] 0, the CDF or Cumulative Distribution Function will be:
\[f_{x}(x)\] = \[\int_{0}^{x}\lambda e - \lambda t\; dt\] = \[1-e^{-\lambda x}\]
For x < 0,
Fx(x) = 0
Mean and Variance of Exponential Distribution
The expected value of the given exponential random variable X can be expressed as:
E[x] = \[\int_{0}^{\infty}x \lambda e - \lambda x\; dx\]
= \[\frac{1}{\lambda}\int_{0}^{\infty}ye^{-y}\; dy\]
= \[\frac{1}{\lambda}[-e^{-y}\;-\; ye^{-y}]_{0}^{\infty}\]
E[X] = \[\frac{1}{\lambda}\] is the mean of exponential distribution.
Now for the variance of the exponential distribution:
\[EX^{2}\] = \[\int_{0}^{\infty}x^{2}\lambda e^{-\lambda x}dx\]
= \[\frac{1}{\lambda^{2}}\int_{0}^{\infty}y^{2}e^{-y}dy\]
= \[\frac{1}{\lambda^{2}}[-2e^{-y}-2ye^{-y}-y^{2}e^{-y}]\]
= \[\frac{2}{\lambda^{2}}\]
Var (X) = EX2 - (EX)2 = \[\frac{2}{\lambda^{2}}\] - \[\frac{1}{\lambda^{2}}\] = \[\frac{1}{\lambda^{2}}\]
Therefore the expected value and variance of exponential distribution is \[\frac{1}{\lambda}\] and \[\frac{2}{\lambda^{2}}\] respectively.
Exponential Random Variable Sum
The Gamma random variable of the exponential distribution with rate parameter λ can be expressed as:
\[Z=\sum_{i=1}^{n}X_{i}\]
Here, Z = gamma random variable
and Xi and n = independent variables
Memorylessness Property of Exponential Distribution
Amongst the many properties of exponential distribution, one of the most prominent is its memorylessness. To understand it better, you need to consider the exponential random variable in the event of tossing several coins, until a head is achieved. If nothing as such happens, then we need to start right from the beginning, and this time around the previous failures do not affect the new waiting time.
P (X > x + a | X > a ) = P ( X > x )
Here X is an exponential parameter >0 .
Therefore, X is the memoryless random variable.
The above equation depicts the possibility of getting heads at time length 't' that isn't dependent on the amount of time passed (x) between the events without getting heads.
Exponential Distribution Applications
The exponential distribution is one of the most popular continuous distribution methods, as it helps to find out the amount of time passed in between events. From testing product reliability to radioactive decay, there are several uses of the exponential distribution.
It is with the help of exponential distribution in biology and medical science that one can find the time period between the DNA strand mutations.
Understanding the height of gas molecules under a static, given temperature and pressure within a stable gravitational field.
It also helps in deriving the period-basis (bi-annually or monthly) highest values of rainfall.
Exponential Distribution Example Problems
Question: If a certain computer part lasts for ten years on an average, what is the probability of a computer part lasting more than 7 years?
Answer: For solving exponential distribution problems,
Take x = the amount of time in years for a computer part to last,
Since the average amount of time ( \[\mu\] ) = 10 years, therefore, m is the lasting parameter
m = \[\frac{1}{\mu}\]= \[\frac{1}{10}\] = 0.1
Therefore, for P(x>7) = 1 - P(x<7)
P(X<x) = 1 - \[e^{-mx}\]
That is, for P(X>x) = 1 - ( 1 - \[e^{-mx}\] )
P(X>x) = \[e^{-mx}\]
Thus, putting the values of m and x according to the equation,
P(X>x) = \[e^{(-o.1)(7)}\]
= 0.4966
Hence the probability of the computer part lasting more than 7 years is 0.4966 0.5.
FAQs on Exponential Distribution
1. What is the Formula for Exponential Distribution?
There exists a unique relationship between the exponential distribution and the Poisson distribution. Taking the time passed between two consecutive events following the exponential distribution with the mean as μ of time units. Since the time length 't' is independent, it cannot affect the times between the current events. Now the Poisson distribution and formula for exponential distribution would work accordingly. It can be expressed as:
P (X = x) = me-mx
= 1/μ e(1/μ)(x)
Here, m is the rate parameter and depicts the avg. time between events.
The relationship between Poisson and exponential distribution can be helpful in solving problems on exponential distribution.
2. What is the Median of an Exponential Distribution?
As the random variable with the exponential distribution can be represented in a density function as:
f(x) = e(-x/a)/A
where x represents any non-negative number.
e = mathematical constant with the value of 2.71828
As the probability density for any negative value of x =0, therefore integrating the equation gives;
0.5 = ∫0M f(x) dx
0.5 = -e-M/A + 1
ln(½) = -M/A
Therefore, once we multiply A on each of the sides, the median would be:
M = A ln2
which represents the median for exponential distribution in the given equation.
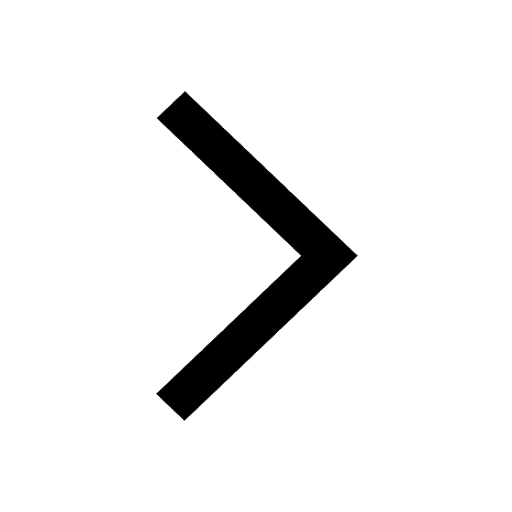
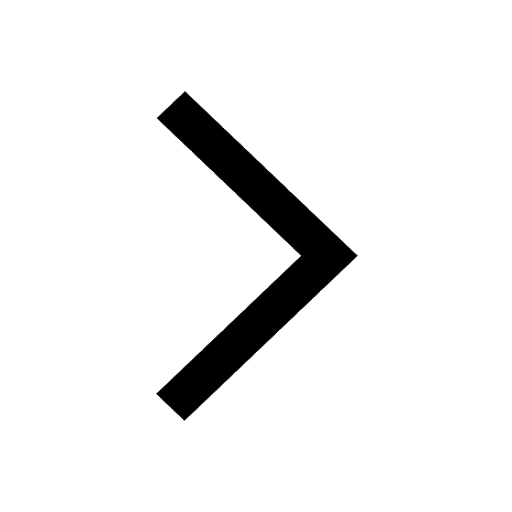
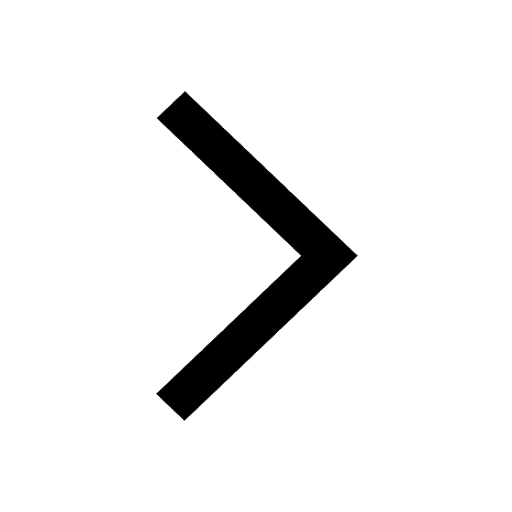
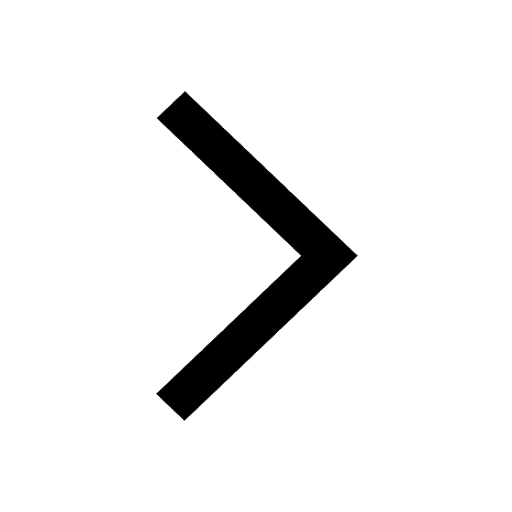
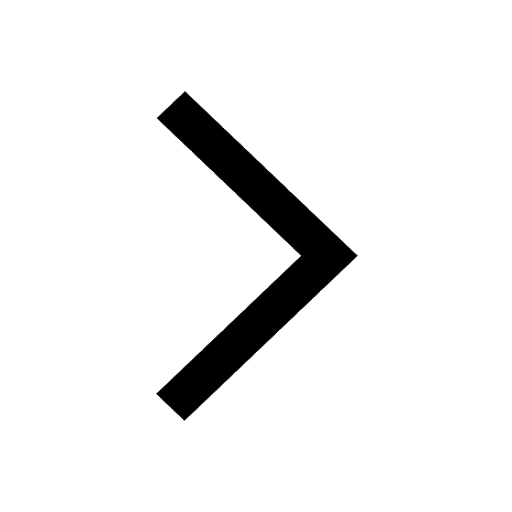
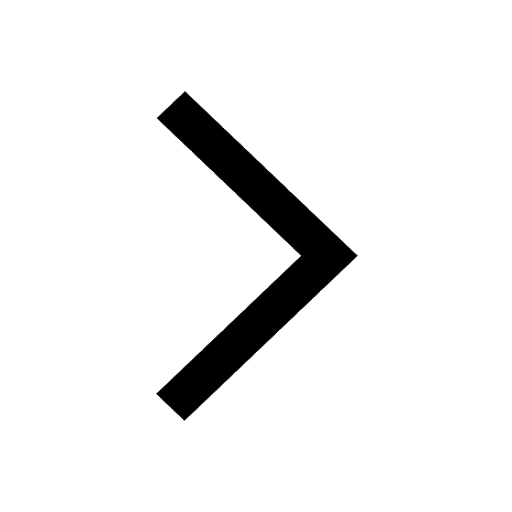
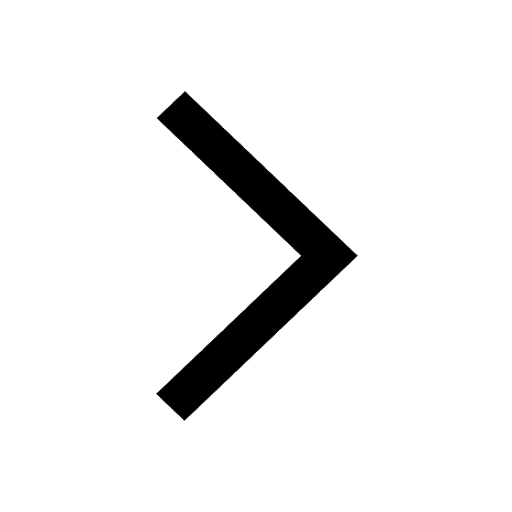
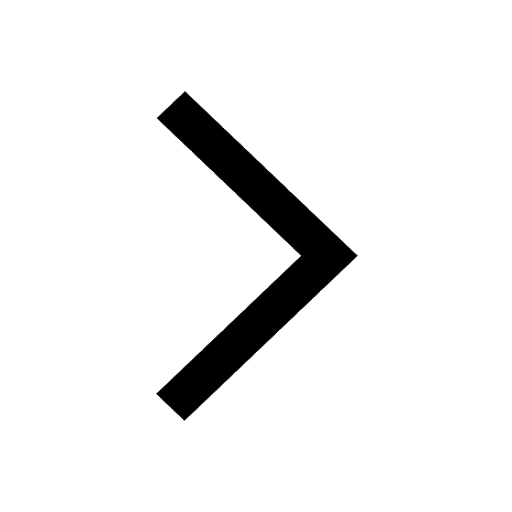
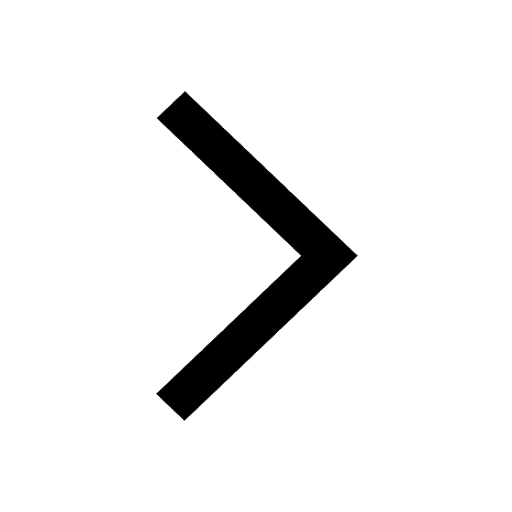
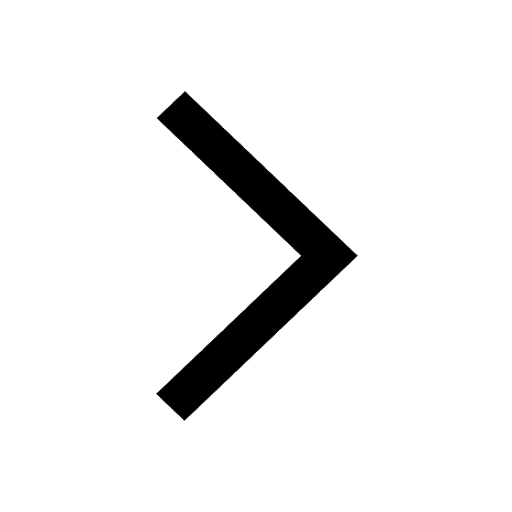
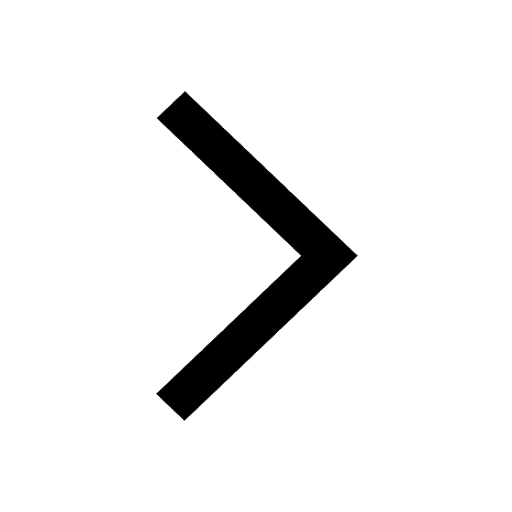
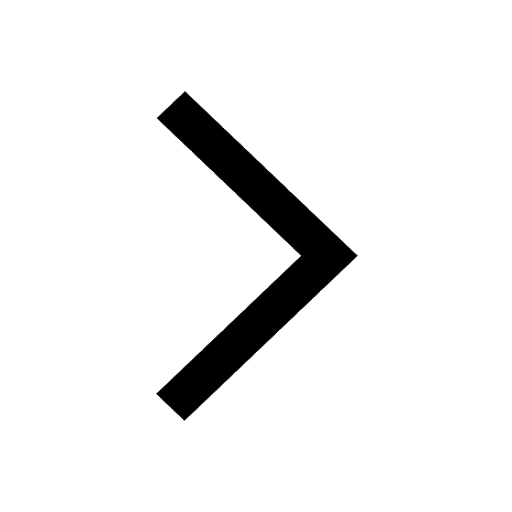
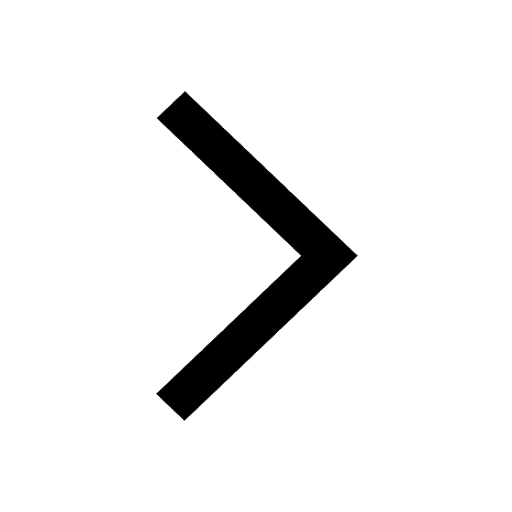
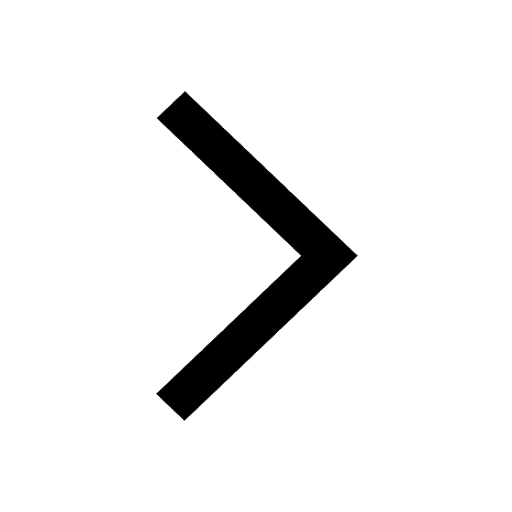
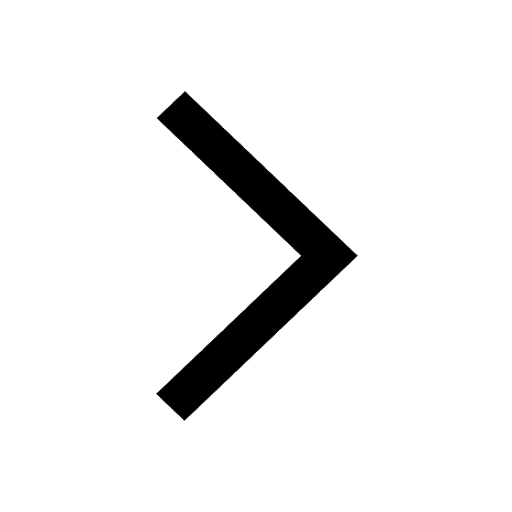
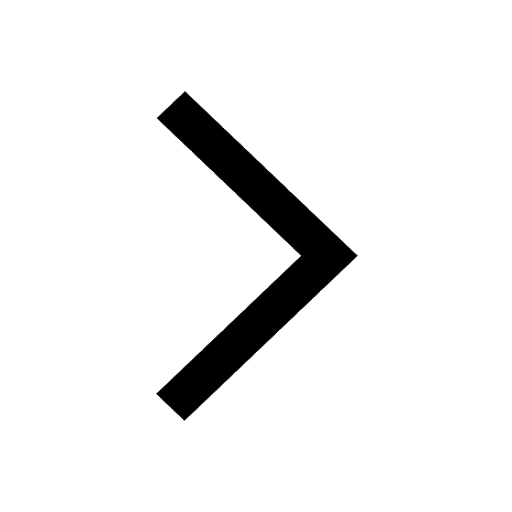
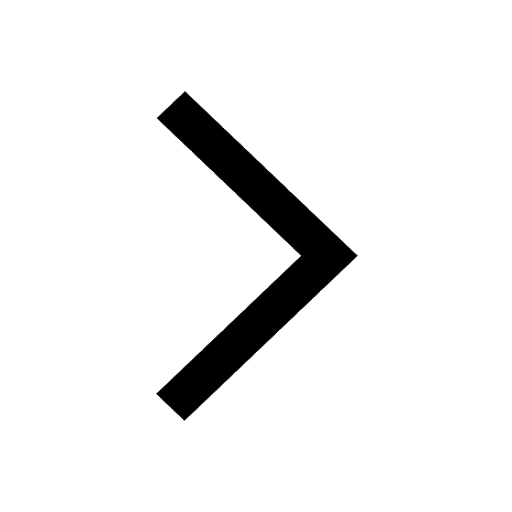
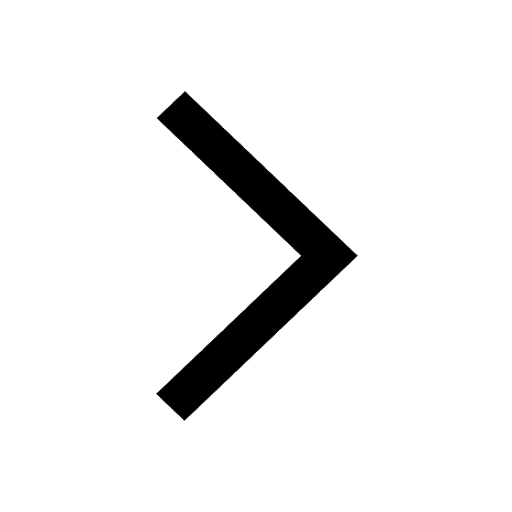