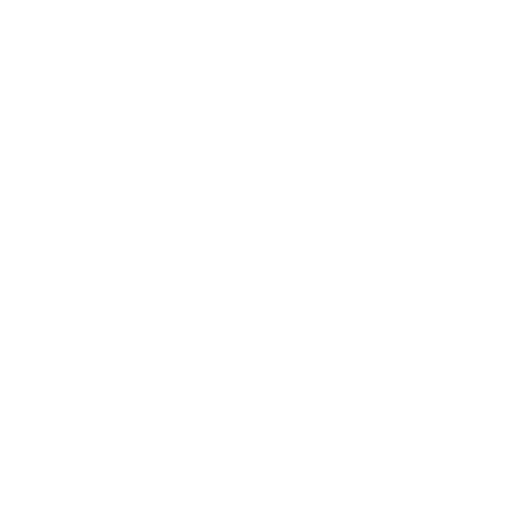
Bias Definition In Statistics from Vedantu
A bias is the deliberate or involuntary favouring of one class or outcome over other potential groups or outcomes in the chosen set of data. If you are asked to define bias in statistics- it is a phenomenon that occurs when a model or data set is unrepresentative. This sampling procedure highlights some grave issues for the researcher as a simple raise cannot ease it in sample size. Bias portrays the actual variation between the expected value and the real value of the parameter considered for the assay. There are multiple sources of bias that result in this. It is a drawback in statistical analysis and needs to be rectified in order to provide accurate data investigation.
In this article, all types of bias have been discussed in detail to help you identify potential sources of bias while planning a sample survey. On identifying a probable bias, it is important to determine whether the result is an overestimate or an underestimate.
A statistical term that means a systematic deviation from the actual value. Bias a sampling procedure which may show certain issues for a researcher, since a mere increase cannot reduce it in sample size. The difference between the expected value and the real value of the parameter is what Bias is. Bias can be described as a kind of phenomenon which can occur when any model or any data is unresponsive.
Bias in Statistics can be of many types and is classified into two parts, the Measurement Bias and The Non Representative Sampling Bias.
Different Types of Bias in Statistics
The major types of bias that can significantly affect the job of a data scientist or analyst are:
Selection bias
Self-selection bias
Recall bias
Observer bias
Survivorship bias
Omitted variable bias
Cause-effect bias
Funding bias
Cognitive bias
Spectrum Bias
Data-Snooping Bias
Omitted-Variable Bias
Exclusion Bias
Analytical Bias
Reporting Bias
As per the sampling method in statistics, bias can be critically segregated into two major classifications:
Measurement Bias.
This takes place for the entire duration when carrying out a survey, and the reasons for its consequences can be said to be because of the following;
The Error Takes Place Only When Recording Data.
When recording any data, we get errors because of the malfunction of the instruments used for data collection, or, also due to the ineffective handling of the tools by the concerned data collection people.
Leading Questions for survey.
Preparations of the questions that are required for the survey might be put in a manner which is interviewer -friendly, answers will be according to the interests of the interviewer, questions that will be answered which are preferred by the interviewer/researcher. There should be more choices for them to get a proper report.
False Responses from Respondents.
Situations can arise when many responders misunderstand the questions and give an incorrect option.
In the care of slightly older respondents, when they are expected to fill the survey answers by remembering their previous experiences, this may cause further misunderstanding and this could fetch incorrect inputs due to weak record keeping.
Non-Representative Bias (Selection Bias):
This happens when a survey sample represents the population inaccurately, which is due to working involuntarily with only a specific division of population and here the sample becomes unrepresentative of the whole population.
The major types of selection bias are:
Undercoverage Bias which occurs when some respondents of the sample population are not wholly represented. The reason behind such a bias is the convenience of sampling, which takes place when the data is collected from an easily accessible source. Example can be the local supermarket.
Non-response Bias, occurs when the individuals who are identified to represent a survey are unwilling or unable to participate in the survey. In this case, the respondents have an upper hand regarding the survey’s outcome.
Voluntary response bias occurs when members who take samples are the self-selected volunteers. For example, the call-in radio shows. These Responses give a faulty and wrong representation of the overall population who are in favor of strong opinions.
Volunteer Bias in statistics can be described by the situation where the population that volunteers for the trials may not represent the targeted respondents.
Survivorship Bias refers to that type of survey which calls for the survival of a lengthy process for being counted as a complete response that gives rise to biased sampling.
All information that defines bias in statistics is included in this article with special focus on different kinds of bias, leading to a clear idea about identification as well as rectification of bias in data analysis.
Did You Know?
An estimator in statistics is a set of protocols for estimating a quantity based on collected data. A biased estimator is the one that gives a false reflection of the population parameter. Suppose you are in a party, playing the game of “bell the cat” where you get to stick the bell to the cat’s picture while being blindfolded. The person, who pins the bell closest to where the bell should go on the neck, wins the game. But unfortunately, even after trying ten times, you tend to put the bell either on the nose or the stomach or the ears of the cat. In this case, your estimation about the location of the exact position of where the bell must be pinned to is a biased estimator.
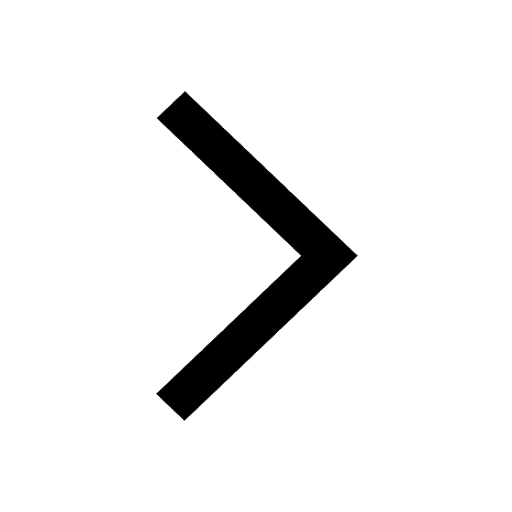
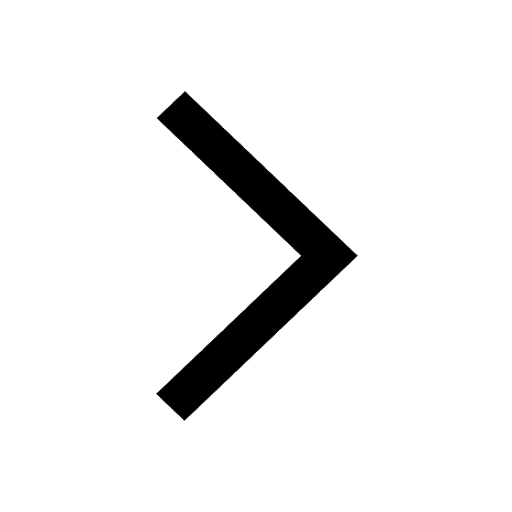
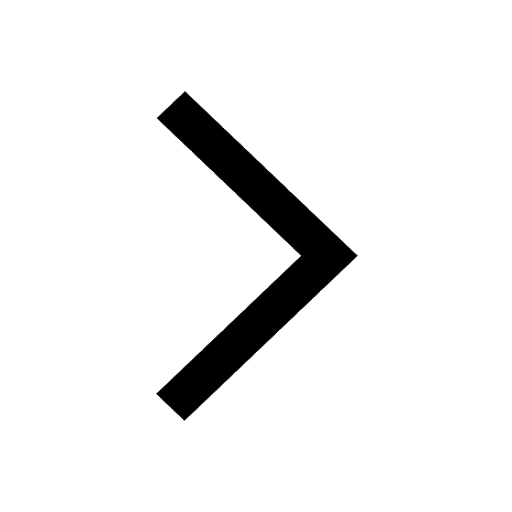
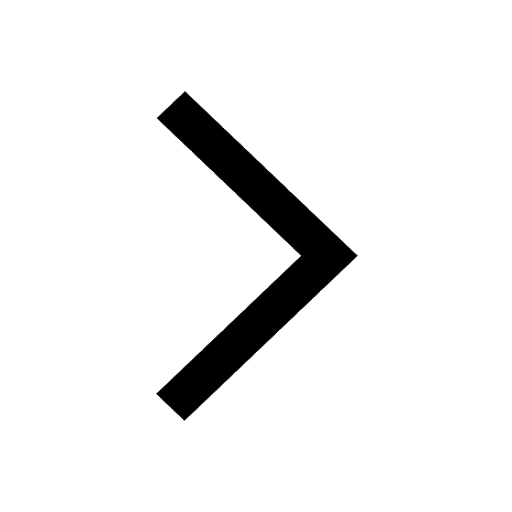
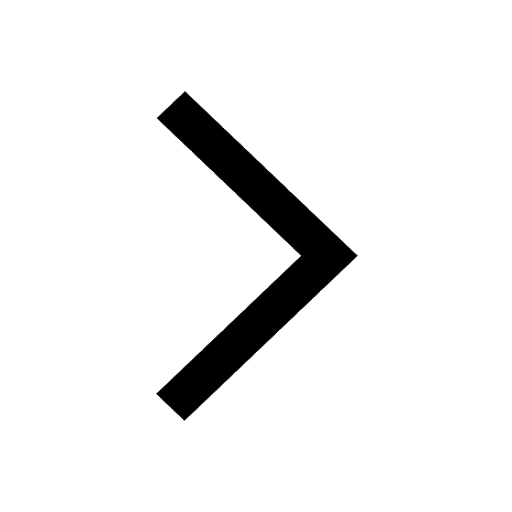
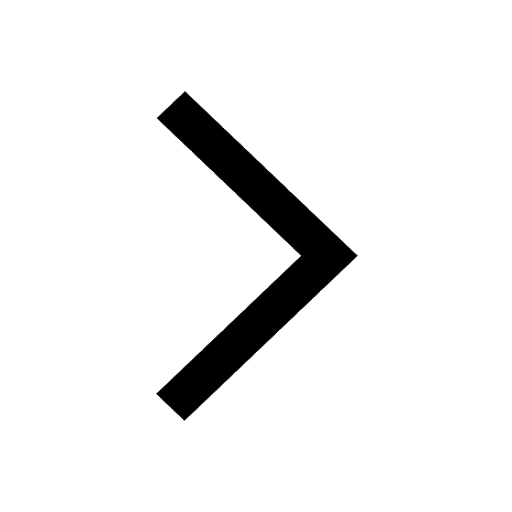
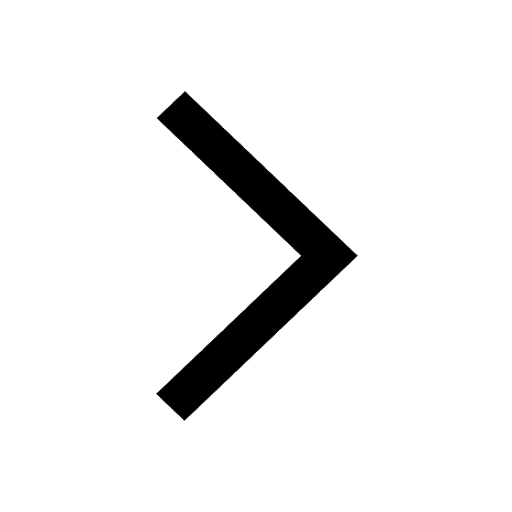
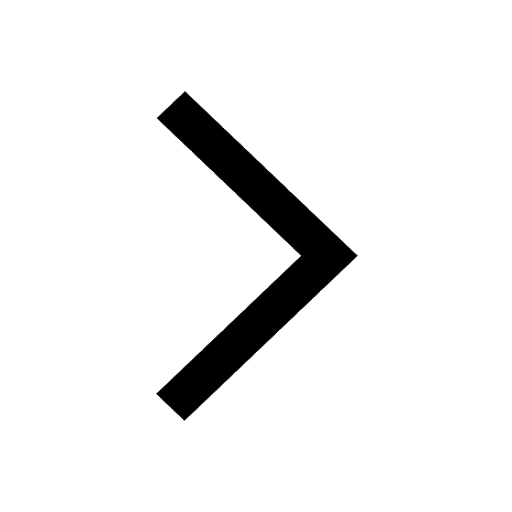
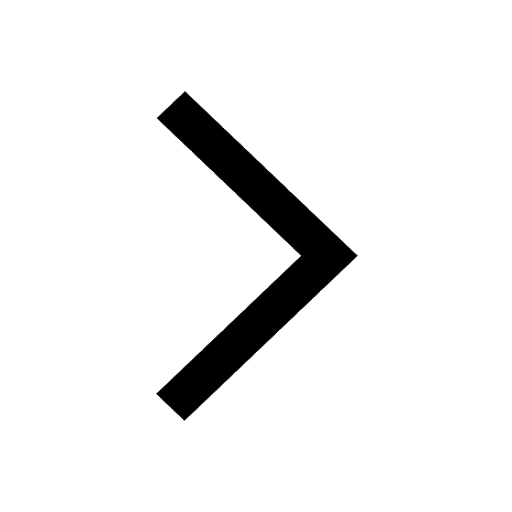
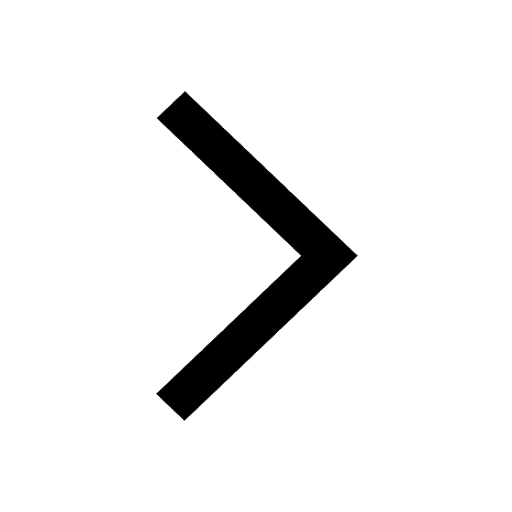
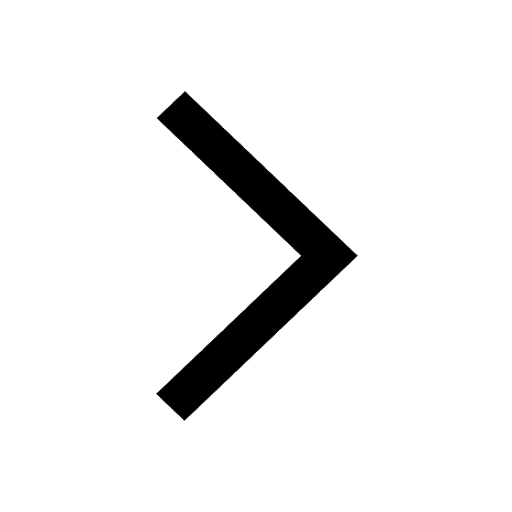
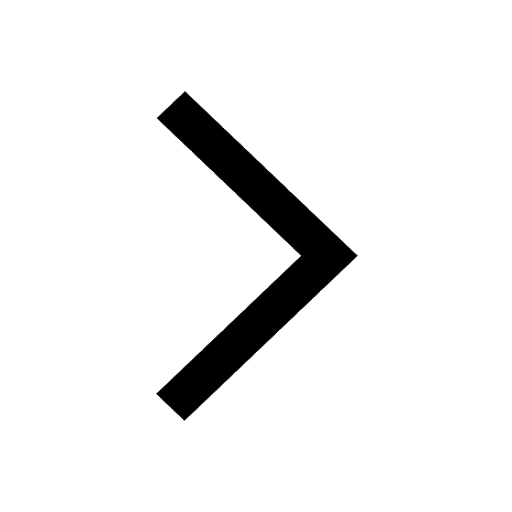
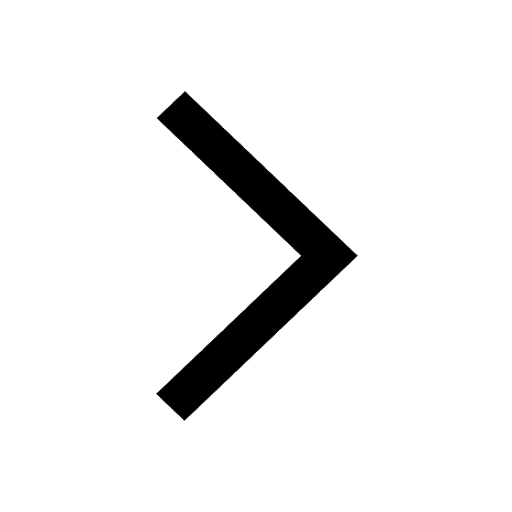
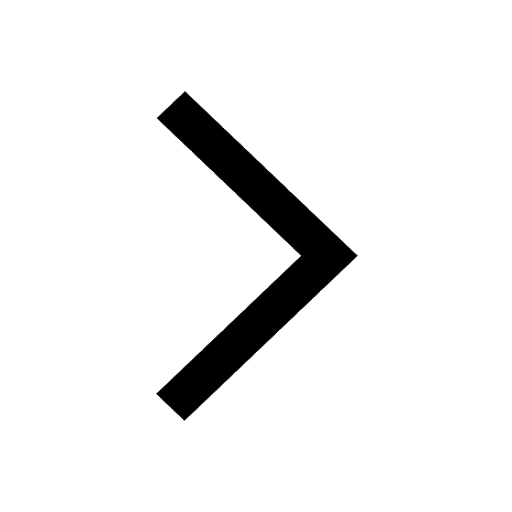
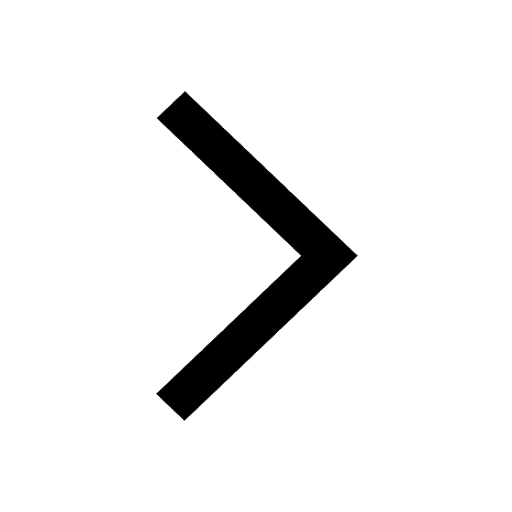
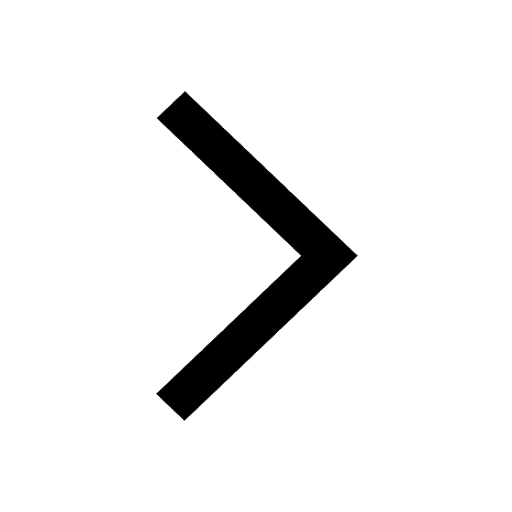
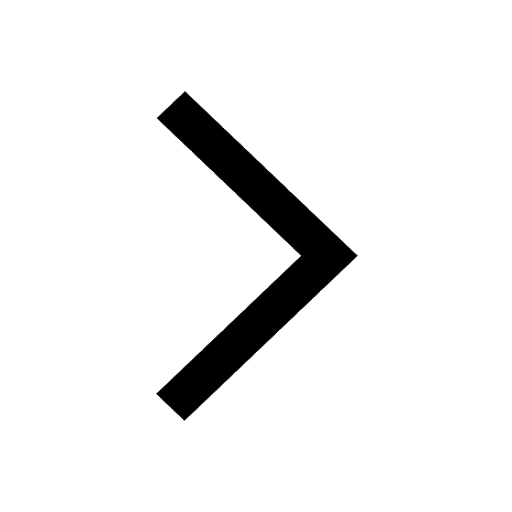
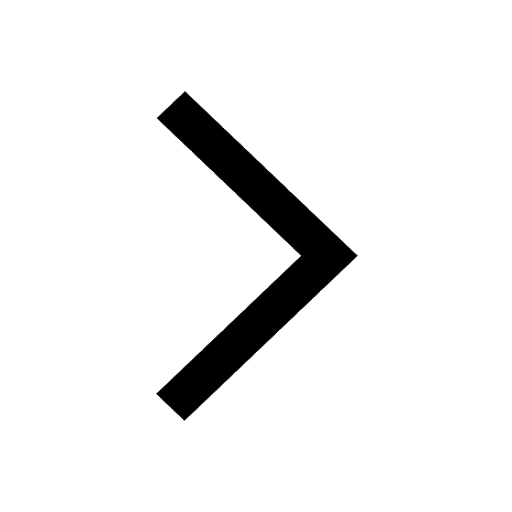
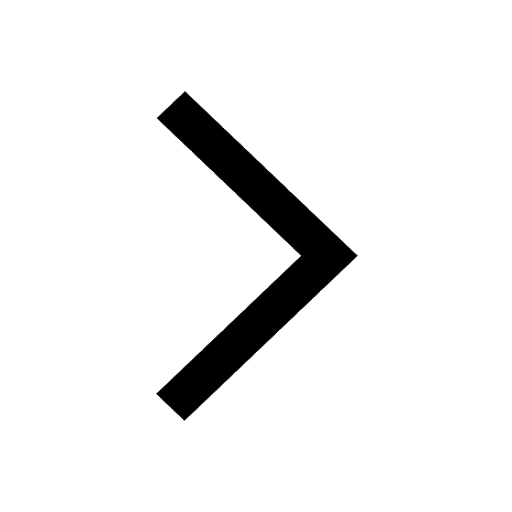
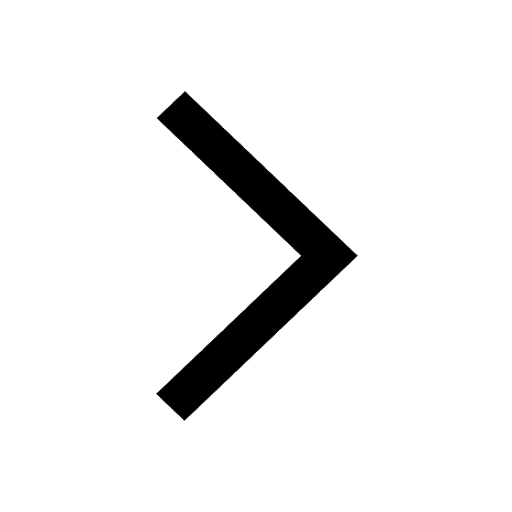
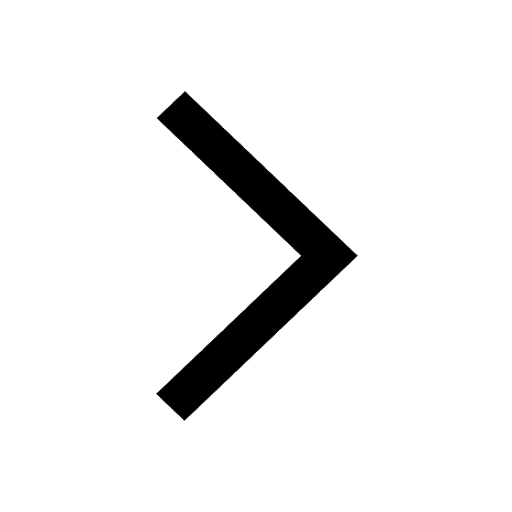
FAQs on Bias
1. What do you mean by Bias in Statistics?
The term Bias,in Statistics is a term which describes the various tendencies of the measurement process. Evaluation of the over or underestimation of the values of the population parameter is what Bias does. The difference between the expected value and the real value of the parameter is what Bias is. Bias can be described to be the systematic derivation from the actual value.The involuntary favoring of one outcome over other potential groups in a chosen set of data.
2. Mention different types of Cognitive Bias?
A manner in which the framework of information can influence a respondent’s ability to make decisions is known as Cognitive and Cognitive Bias can be of various types:
Overconfidence Bias; Self-Serving Bias; Framing Cognitive Bias; Hindsight Bias; Herd Mentality; Loss Aversion; Narrative Fallacy; Representative Heuristic
And some of the common factors that can help identify all the types are
i) the interference with human individuality, and ii) those that lead to judgment deviating from coherent impartiality.
3. How to control Information Bias?
The Information Bias can be controlled by the implementation of a homogeneous mode of operation for collecting data across various groups which can be done by ensuring that the scientists or the persons conducting the survey are all aware of the participants' backgrounds. A major priority here is proper training of the interviewers who are eligible to collect data using a particular standardized way. And at atleast two different sources but from the same background should be included in order to study variations.
4. Why is it important to have a clear concept of Physics?
An important subject which is taught to every student from school and always been a subject that needs to be understood clearly. It is a fundamental science from where we can get the latest and the most advanced knowledge and techniques of technology. It also contributes to the technological infrastructure and we get lots of formulas that are used in everyday life, by people from all walks of life. Hence, a basic understanding of Physics always is considered to be of utmost importance for everyone.
5. Where can we get study material ?
It is important to practice some of the important questions in order to do well. Vedantu offers these important questions along with answers that have been formulated in a well structured, well researched, and easy to understand manner. The NCERT and other study material with Chapter wise solutions are very easily accessible from Vedantu.com. Practicing and studying with the help of these questions along with the solutions enable the students to measure their level of proficiency, and also allows them to understand the difficult questions with ease.
6. What are the Different Types of Cognitive Bias?
Cognitive can be defined as an orderly manner in which the framework of information influences the respondent’s decision-making ability. The different types of cognitive bias are:
Overconfidence bias
Self-serving Bias
Herd Mentality
Loss Aversion
Narrative Fallacy
Anchoring Bias
Hindsight Bias
Representative Heuristic
Confirmation Bias
Framing Cognitive Bias
The common factors that can identify all types of cognitive bias are:
interferes with human individuality
lead to a judgment that deviates from coherent impartiality
7. How Can Information Bias be Controlled?
The foremost method to control information bias is by implementing homogeneous modus operandi for collecting data across groups. This can be done by ensuring that the scientists or the surveyor are unaware of the background of the participants. Proper training of interviewers that are completely eligible to handle and collect data using a standardized method is one of the priorities. It is also important to implement strategies to identify potential sources of bias. At least, two different sources from the same background must be included to study the variation in responses.