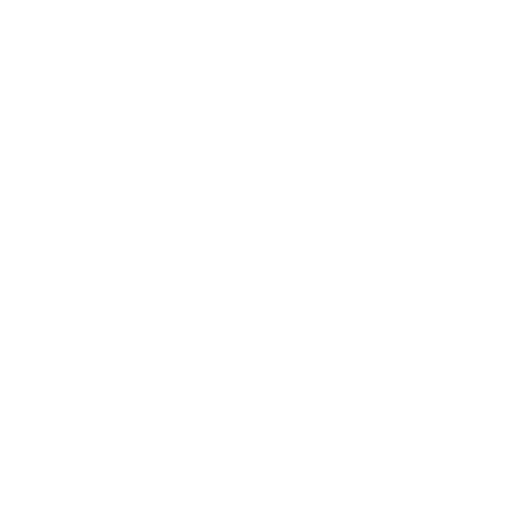
Correlation and regression are techniques used to establish relationships between variables. We use the word correlation in our life every day to denote any type of association. For example, there is a correlation between foggy days and wheezing attacks. Similarly, regression examples are present in business during the launching of a program, etc., where it is said “thinking backward,” i.e., you project what you want to do and then start planning on what all is needed to be done to reach that point.
This article will know the details of correlation regression and get to know correlation and regression formula. If you want to get a clear picture of all aspects of correlation and regression, you can download this correlation and regression pdf for ease of use.
Correlation
If we break up the word correlation, the part “Co” means “together,” and relation is how two things are related concerning each other. In statistical terms, using correlation you can quantify the strength and the direction of the relationship between two variables. Here the assumption is that the association is linear, i.e., there will be an increment or decrement in one variable by a fixed amount when there is a unit change (increment or decrement) in the other variable.
Correlation Coefficient
The correlation coefficient measures the strength or degree of association between the two variables and is denoted by r. It is also called Pearson’s coefficient as Karl Pearson invented it, and it measures linear associations. For a curved line, one needs other, more complex measures of correlation.
[Image will be Uploaded Soon]
The scale of correlation ranges from -1 to 0 to +1. If there is a complete and strong correlation between two variables, the values are either +1 or -1, depending on whether it is a positive or a negative correlation. If there is no correlation, then the value of the correlation coefficient will be 0.
Positive Correlation
When the value of one variable increases with an increase in another variable, then it is a positive correlation between variables. For example, as you grow in height, your weight also increases. With an increase in the temperature of a location, its ice cream sales also go up.
Negative Correlation
When the value of one variable decreases with an increase in another variable, then it is a negative correlation between variables. For example, when you exercise more, your weight reduces more, or as you go higher up a mountain, the temperature decreases.
The formula for the correlation coefficient is given by:
rab = \[\sum\] (ai - \[\bar{a}\]) (bi - \[\bar{b}\]) / \[\sqrt{\sum (a_{i} - \bar{a})^{2} \sum (b_{i} - \bar{b})^{2} }\]
Where;
rab = correlation coefficient of the relationship between variables a and b
ai = values of variable a in the sample
\[\bar{a}\] = mean of values of variable a
bi = values of variable b in the sample
\[\bar{b}\] = mean of values of variable b
Regression Definition
Regression analysis meaning a statistical technique to collect a set of data to make predictions. Regression involves finding out a relationship between a dependent variable and one (or more) independent variables.
As the first step of regression statistics, one would usually make a scatter plot to get a rough shape of the data in hand. Then you can choose any one of the regression methods which fits the data best. The shape of the scatter plot (curve, parabola, straight line, etc.) would determine which method you choose for your regression analysis.
To verify the correctness of the regression model chosen, many tests are performed. Suppose the model is found to be satisfactory. In that case, the regression equation thus estimated can be used to predict the values of a dependent variable based on the given values of independent variables.
Linear Regression Model
A regression analysis expresses the relationship between one or more predictor variables with that of an outcome variable quantitatively. We will see a regression analysis example to understand the concept better. The impact of age, gender, and diet on a person's height is common regression examples. Here age, gender, and diet are predictor variables, and height is the outcome variable.
Linear regression is of two types:
1. Simple linear regression - This method employs a single predictor value. In this there is:
A single Predictor, independent, or explanatory variable
An Outcome, response, or dependent variable.
The table below displays the sales of a company for 5 continuous years and the amount they spent on advertising.
Here, if we take advertising expenditure as a predictor variable and sales as the outcome variable, the linear regression estimate can be given by the
Equation:
Sales = 168 + 23 * expenditure on advertising
From the above equation, we can say that if there is a 1 million increase in advertising, sales will increase by 23 million USD. If there is no advertising, then sales would be expected to be at 168 million USD.
Multiple linear regression - A single predictor value gives us a simple linear regression formula. In the real world, there is always more than one predictor; hence we employ multiple regression formulas on it. So in the above example of sales, if we also add “year” as another predictor variable, then our formula would change to:
Sales = 323 + 14 * expenditure on advertising + 47 * year
This equation can be interpreted to say every one million increments in advertising would increase sales by 14 million, and the sales would also grow by 47 million per year (due to non-advertising factors).
[Image will be Uploaded Soon]
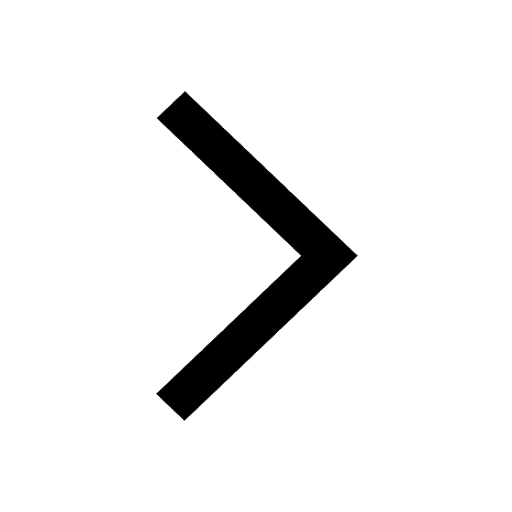
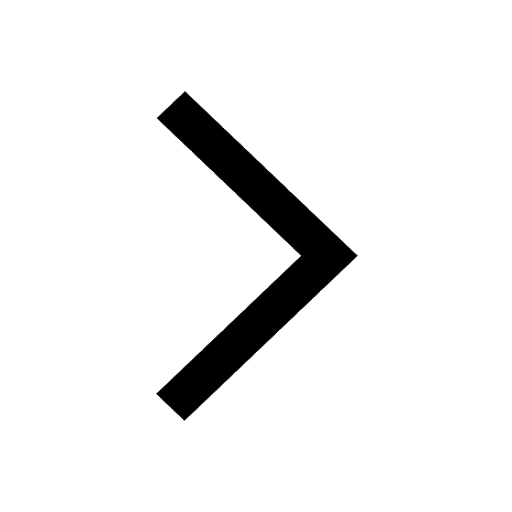
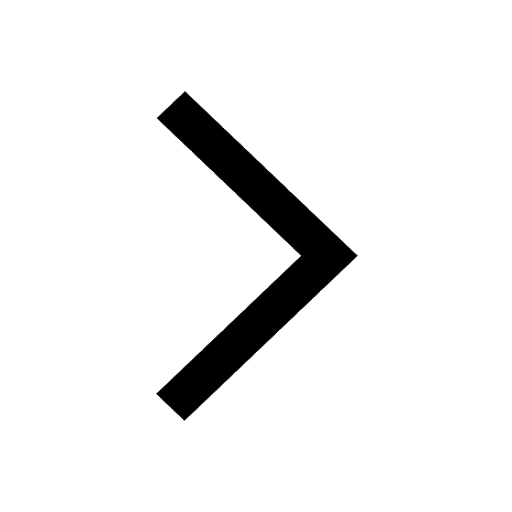
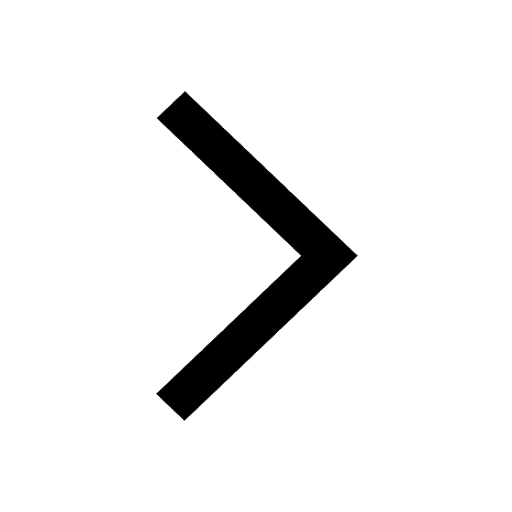
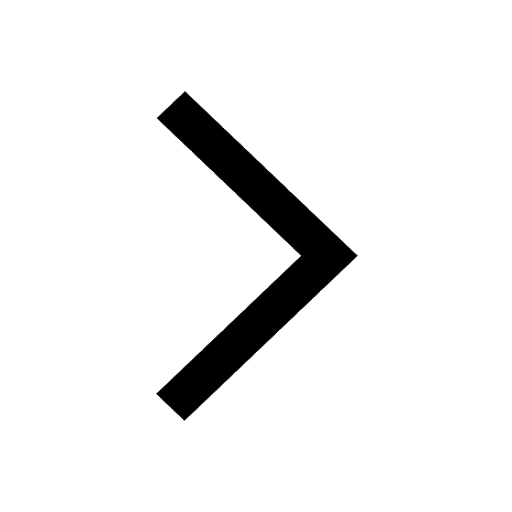
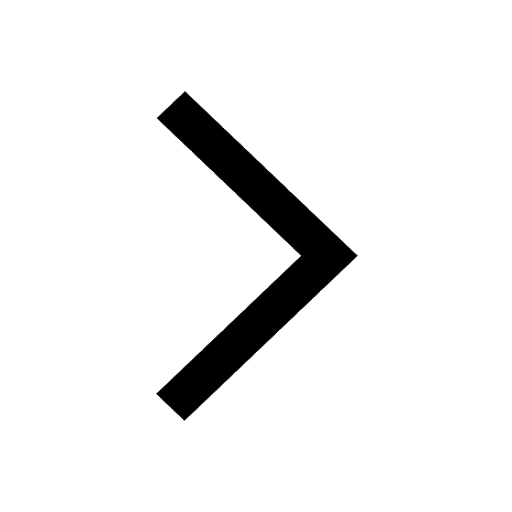
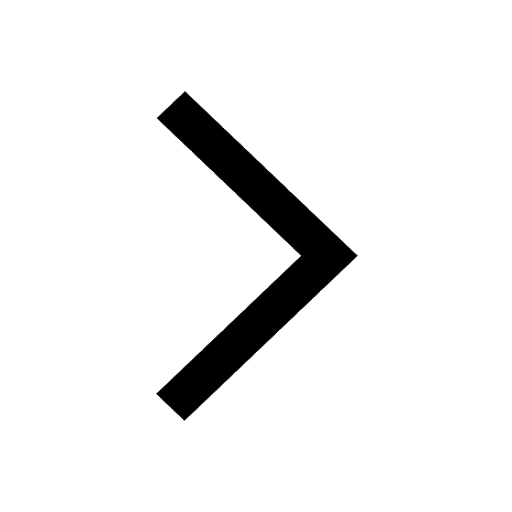
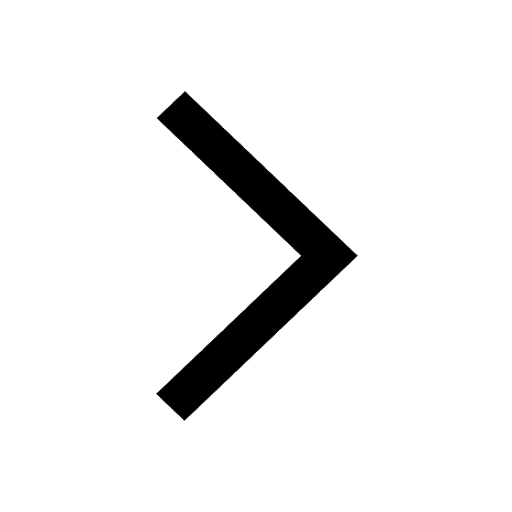
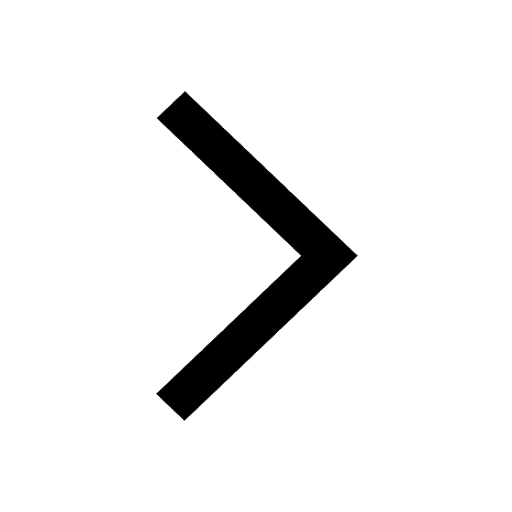
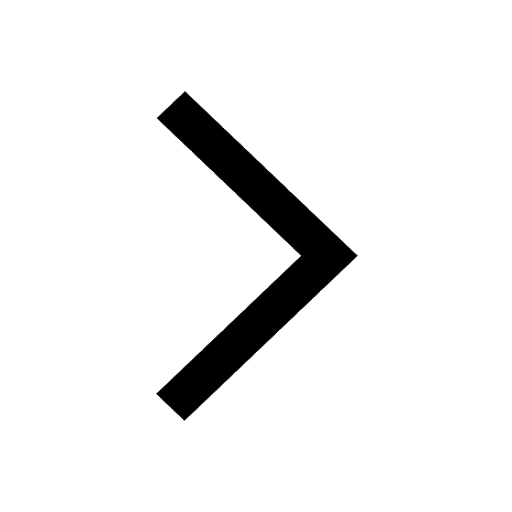
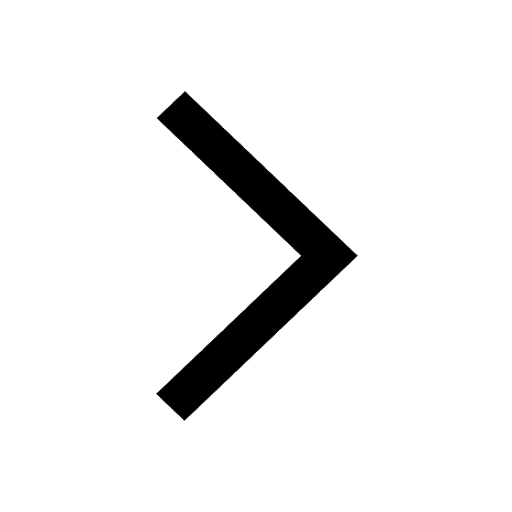
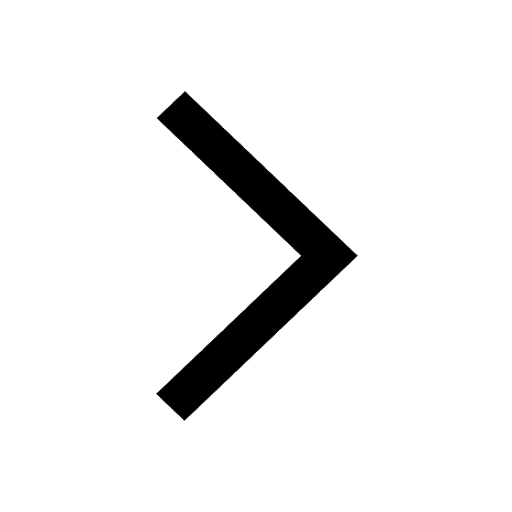
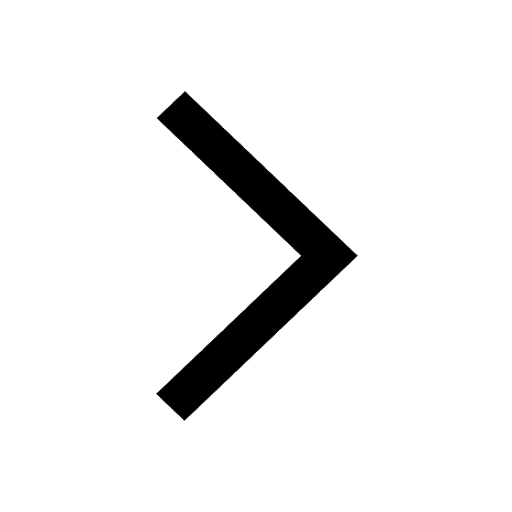
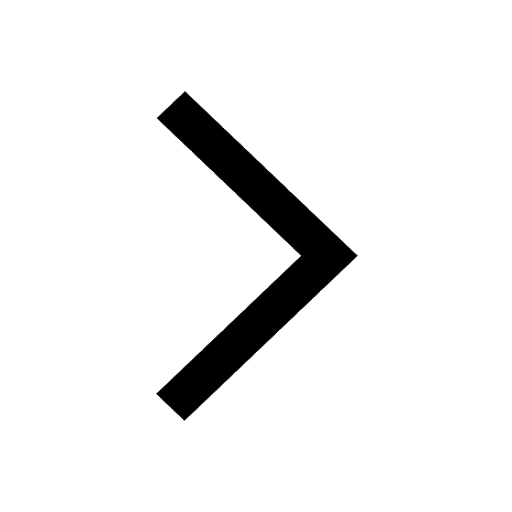
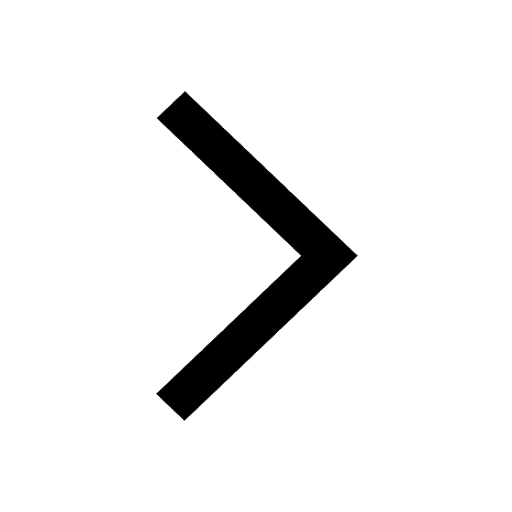
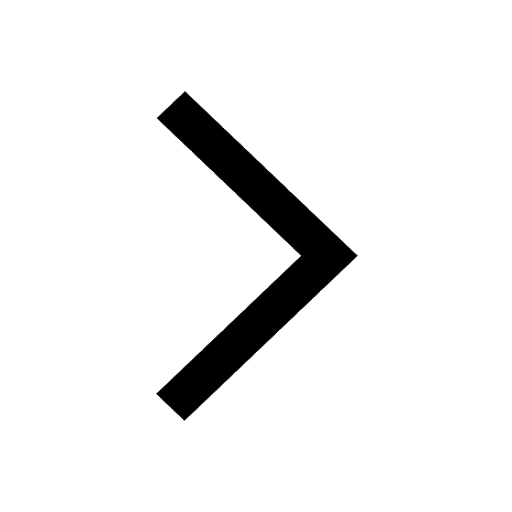
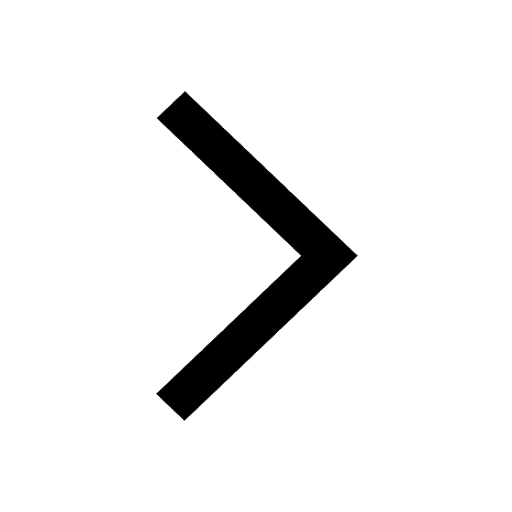
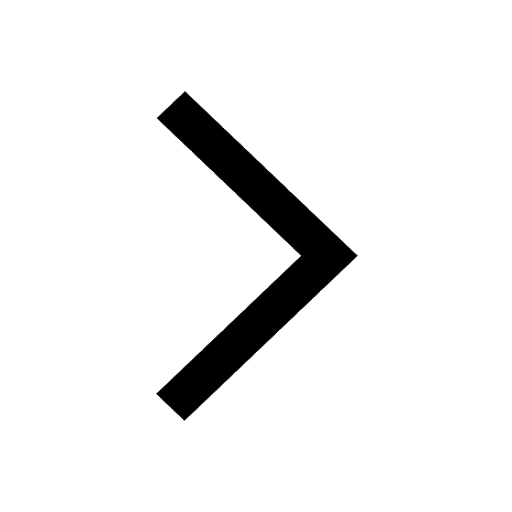
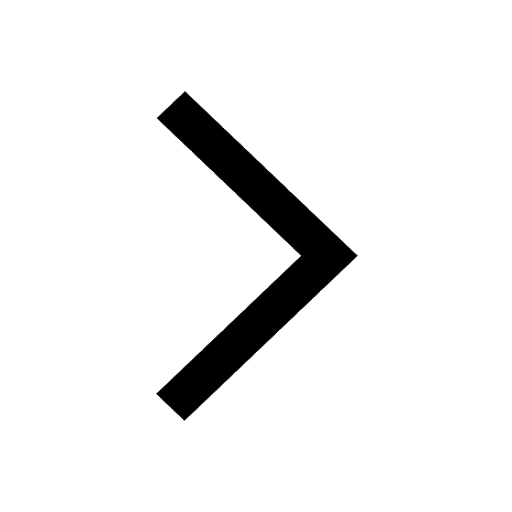
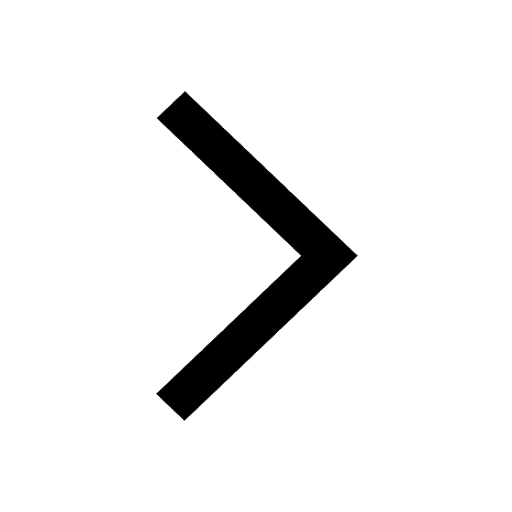
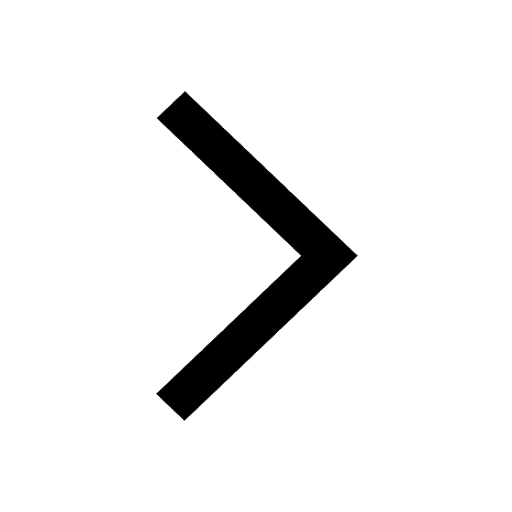
FAQs on Correlation and Regression
Q1: Compare Correlation and Regression Analysis Techniques and When Should You Use Which One.
Ans: Both correlation and regression are used in statistics for describing relationships between variables. They have similarities as well as significant differences. The primary use of regression is to develop equations or models which can predict a key response (R) from a set of predictor values (P). Whereas, the main use of correlation is to quickly give us the direction and strength of the relationship between a set of 2 (or more) variables.
The table below provides a summary of key similarities and differences between correlation and regression:
Context | Correlation | Regression |
Tells us the direction of the relationship | yes | yes |
Can we interchange variables | yes | no |
Can predict and be used to optimize | No | yes |
Can be extended to fit curvilinear relationships | No | yes |
Gives cause and effect | No | It attempts to establish that. |
Variable V1 is random | Yes | No |
Variable V2 is random | Yes | Yes |
When to use | When you need a quick summary of the direction and strength of pairwise variables | When you want to predict and optimize the numeric response of V2 from V1 where V1 is a variable that impacts V2. |
[Image will be Uploaded Soon]
Q2: What are Some of the Business Applications of Regression Analysis?
Ans: Regression is used in the business primary for 5 purposes:
[Image will be Uploaded Soon]
Predictive analysis - One of the most important applications of regression analysis is to forecast risks and future opportunities in business. For example, demand analysis can predict the number of items that a consumer is likely to repurchase.
Operation Efficiency - You can optimize your business processes using regression techniques. For example, a factory manager can build a statistical model based on the shelf life of cookies and the oven temperature they are baked in.
Decision supporting - There is an overload of data in businesses ranging from finances, customer purchases, operations, and so on. Regression analysis is bringing a scientific approach to reduce raw data into meaningful information that managers can use for an informed decision.
Error correction - Sometimes, one can make intuitive choices that might result in errors. For example, a manager might think that extending working hours may increase sales and revenue. But regression analysis might show otherwise. The operating expenses to work longer hours might not justify the increase in sales. So, you can avoid such errors with efficient regression analysis.
Gain new insights - Businesses have an accumulation of a massive volume of unorganized data right now. This data is capable of giving new insights if a proper regression analysis technique is employed.