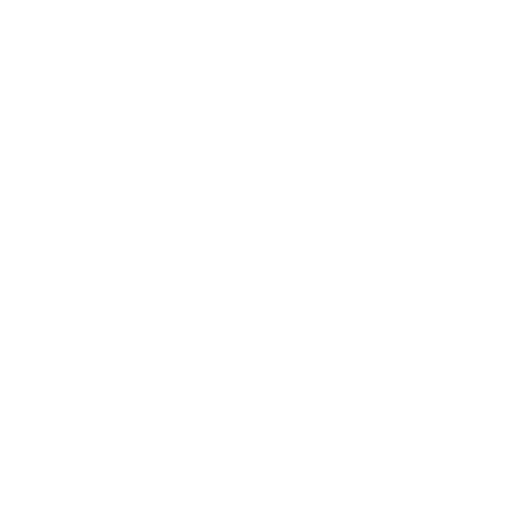
Statistical Inference Definition
Statistical Inference is defined as the procedure of analyzing the result and making conclusions from data based on random variation. The two applications of statistical inference are hypothesis testing and confidence interval. Statistical inference is the technique of making decisions about the parameters of a population that relies on random sampling. It enables us to assess the relationship between dependent and independent variables. The idea of statistical inference is to estimate the uncertainty or sample to sample variation. It enables us to deliver a range of value for the true value of something in the population. The components used for making the statistical inference are:
Sample Size
Variability in the sample
Size of the observed difference
What are the two Types of Statistical Inference?
Although, there are different types of statistical inference that are used to draw conclusions such as Pearson Correlation, Bi-varaite Regression, Multivariate regression, Anova or T-test and Chi-square statistic and contingency table.
But, the most important two types of statistical inference that are primarily used are
Confidence Interval
Hypothesis testing
Statistical Inference Procedure
Following Steps are Included in the Statistical Inference Procedure
Start with a theory
Design research hypothesis
Implement the variables
Acknowledge the population to which the results should be applied.
Draw up the null hypothesis for this population.
Assemble the sample of children from the population and begin the study.
Implement the statistical test to examine if the collected sample properties are sufficiently different from what is expected under the null hypotheses to be able to reject the null hypothesis.
Statical Interference Solutions
Statistical inference solutions deliver efficient use of statistical data with respect to the group of individuals or trials. It manages with every character,including the collection, investigation and analysis of data and organizing the collection of data. Through statistical inference solutions, people can gain knowledge after initiating their work in multiple fields. Some of the statistical inference solution facts are as follows:
It is a usual method to predict that the observed samples are independent observations from a population type such as poison or normal.
Statistical inference solution helps to evaluate the parameter(s) of the expected model such as normal mean or binomial proportion.
Importance of Statistical Inference
Statistical Inference is significant to examine the data properly. To make an effective solution, accurate data analysis is important to interpret the results of the research. Inferential statistics is used in the future prediction for varied observations in different fields. It enables us to make inferences about the data. It also helps us to deliver a probable range of values for the true value of something in the population. Statical inference is used in different fields such as:
Business Analysis
Artificial Intelligence
Financial Analysis
Fraud Detection
Machine Learning
Pharmaceutical Sector
Share market.
What is Statistical Inference Example
The Statistical Inference example is given below help you to understand the concepts clearly:
From the Shuffled Pack of 52 Cards, a Card is Drawn. The Trial is Repeated for a Maximum of 400 Times and Different Suits of Cards are Given Below :
What is the probability of getting the following suits, if the card is drawn randomly
Diamond Card
Black Card
Except for spade
Solution: Through statistical inference solution.
Total number of events = 400
i.e. 100 + 90+120+90 = 400
The probability of winning a diamond card is :
Total number of trials in which diamond card is drawn= 90
Hence, P(diamond card )= 90/4000
= 0.22
The probability of winning black card is :
Number of trial in which black card appeared = 100 + 90 = 190
Hence, P(black card) = 190./400 = 0.48
Except spade
Number of trials other than spade appeared = 90 + 100 + 120 = 310
Hence, P(except spade) = 310/400 = 0.78
Solved Examples
1. A bad containing 2 yellow balls, 3 red balls, and 5 black balls. Only one ball is drawn at random from the bag. What is the probability that the black ball is drawn?
Solution: Through statistical inference solution.
Total number of balls in a bag = 10
i.e 2 + 3+ 5 = 10
Number of black balls= 5
Probability of getting a black balls = Number of balck balls/ Total number of balls
= 5/10
= 1/2
Hence, the probability of getting black balls is 1/2.
2. A card is drawn from a pack of 52 cards. What is the probability that the card drawn is a face card ( Jack, King and Queen only)?
Solution: Total number of cards= 52
Number of a face card in a pack of 52 cards= 12
Probability of receiving a face card = 12/52 = 3/13
Hence, the probability of receiving face card is 3/13
Facts
The birth date of statistics is 1662 when John Gaunt and William Petty originated early human statical and census methods that deliver a framework for modern demography.
Sir Ronald Fisher, a British Mathematician and Biologist is the father of modern statistics and experimental design.
Statistics was called the science of state or the science of kings in ancient times. As it was used by States or KIngs.
Quiz Time
1. Which of the given below is considered as a random variable?
The outcome from a roll of the die
The outcome of the flip of a coin
The outcome of the exam
All of the above
2. Statistical inference is the method of drawing the formal conclusion form the given data
True
False
3. Hypothesis testing and estimation are the two important branches of the field of inferential statistics.
True
False
4. The optimum use of the laws of probability to make an inference and draw the statical conclusion about the population on the basis of sample data is referred to as
Descriptive statistics
Inferential statistics
Sample Statistics
Population Statistics
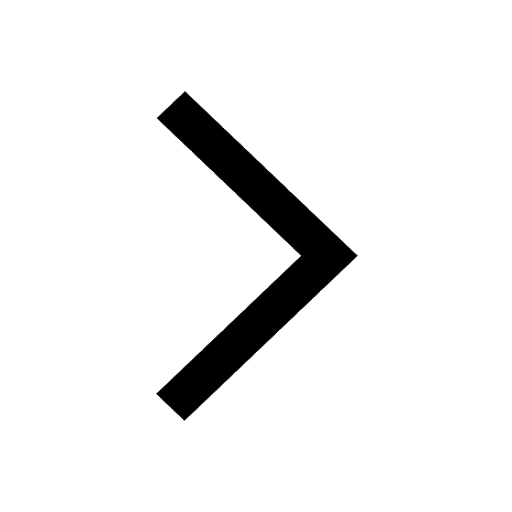
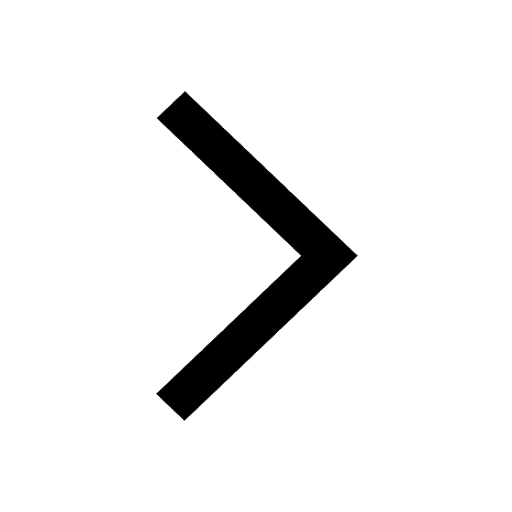
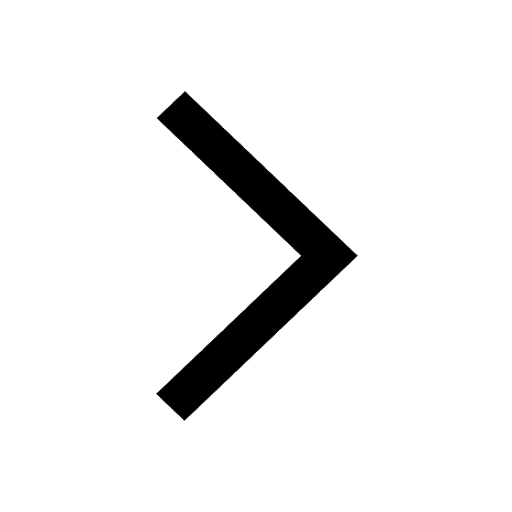
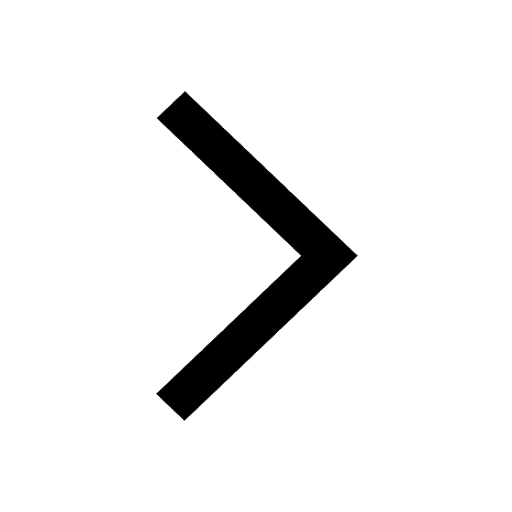
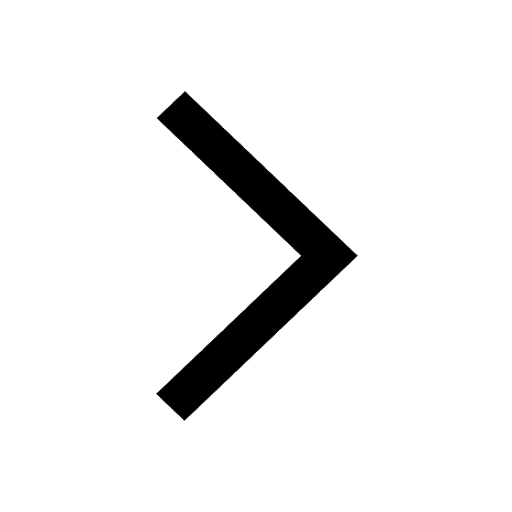
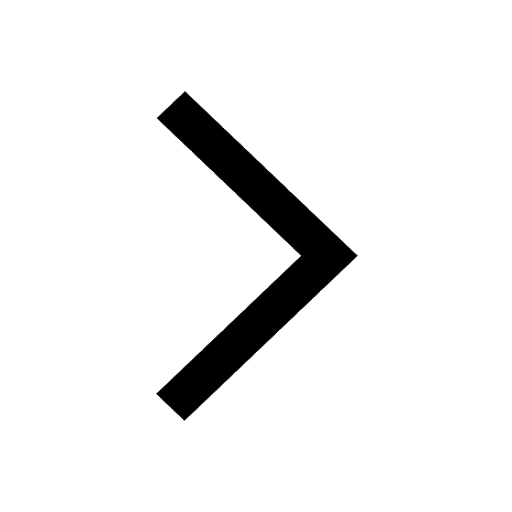
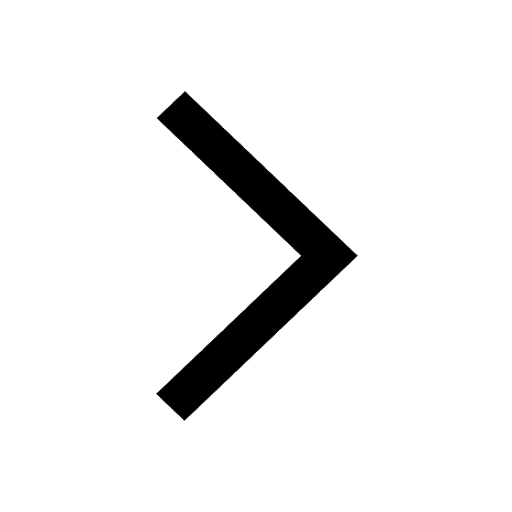
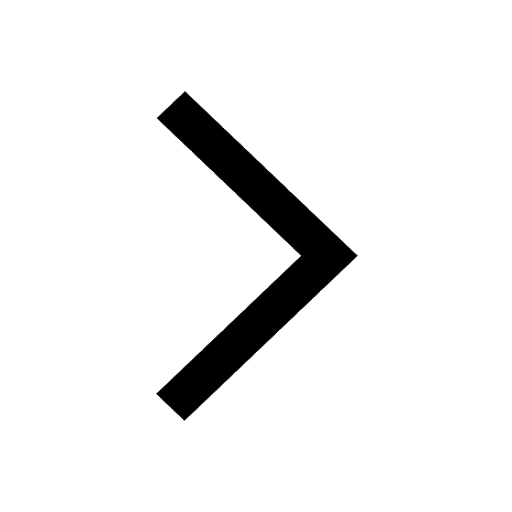
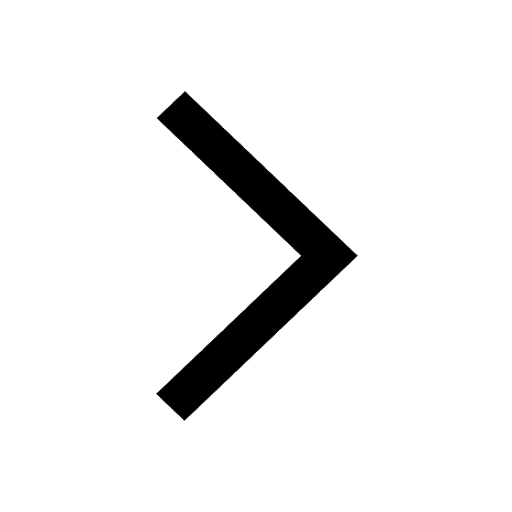
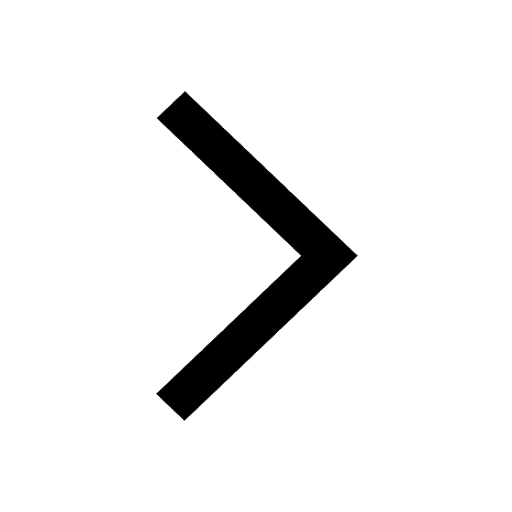
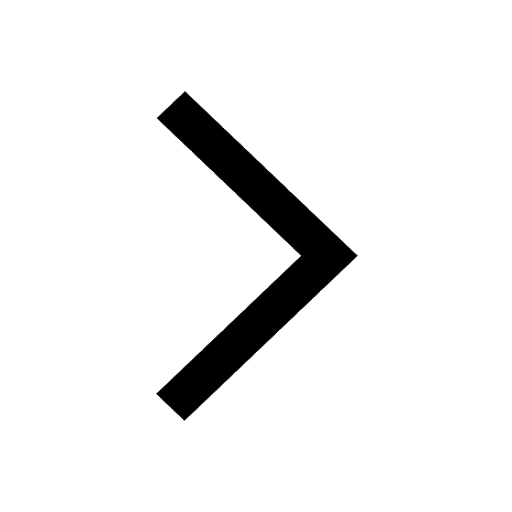
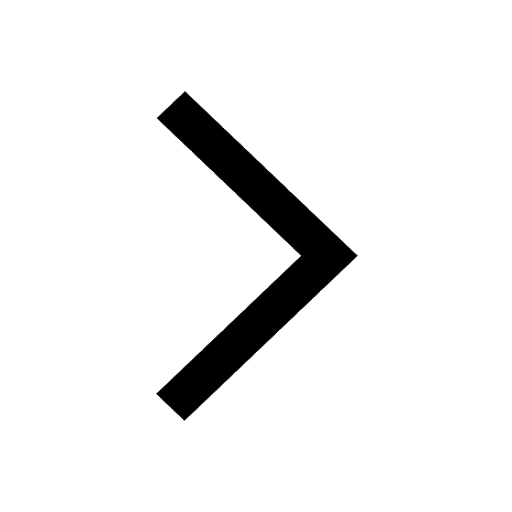
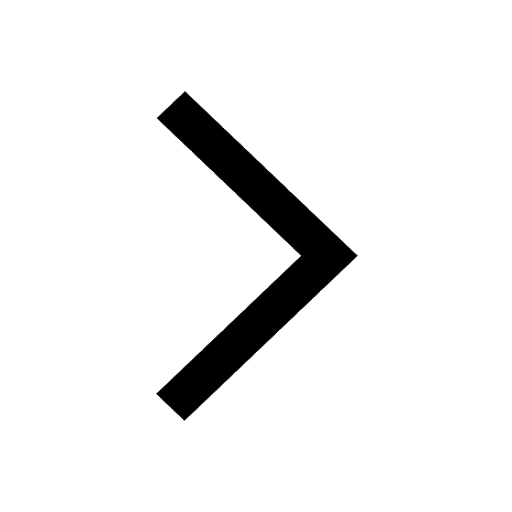
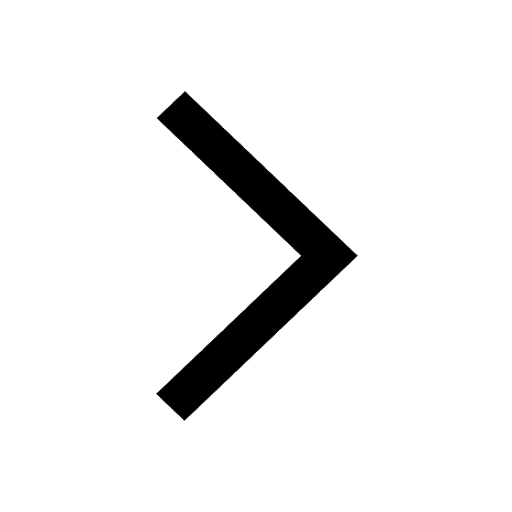
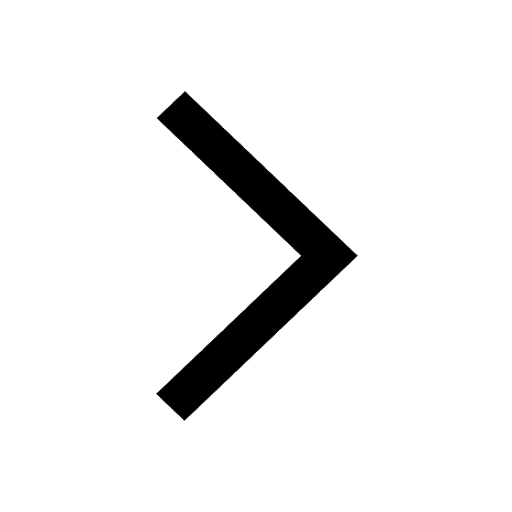
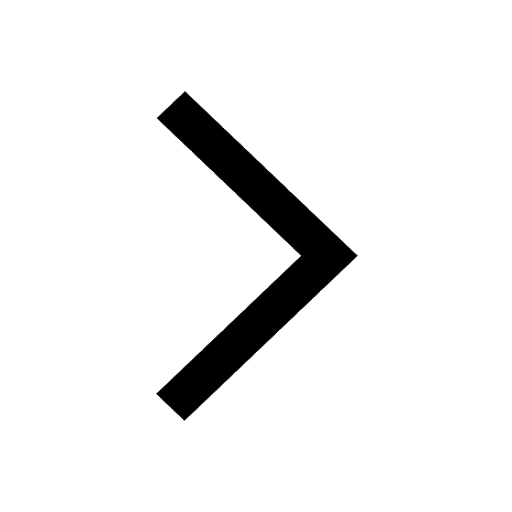
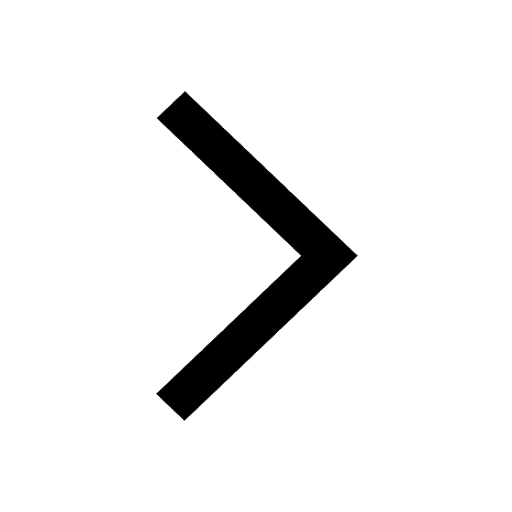
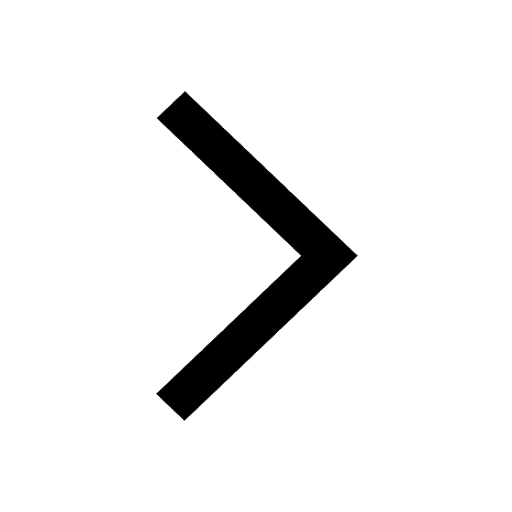
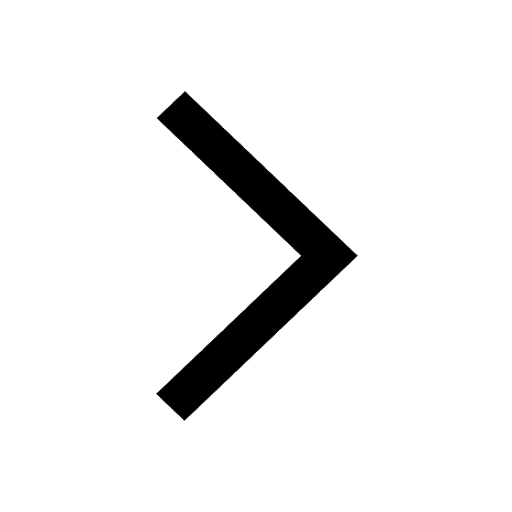
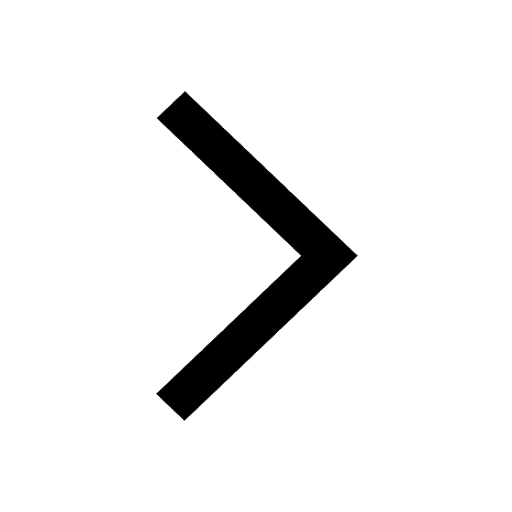
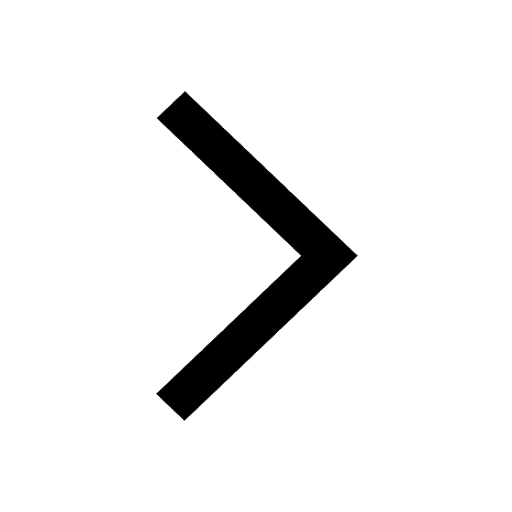
FAQs on Statistical Inference
1. What is Statistics? Explain its Types.
Statistics is the detailed study of collection, organization, interpretation and presentation of data. In other ways, it is a form of mathematical analysis that collects and summarizes the data. It is used in different areas such as business, manufacturing, psychology, government, manufacturing, humanities etc. Statistics data is collected using a sample procedure or other different methods.
The two types of statistical procedures to analyze data are descriptive statistics and inferential statistics. Inferential data are used when data is examined as a subdivision of a particular population where descriptive statistics are used to assess data from a sample practicing the mean or standard deviation.
The statistic is classified into two groups. The two distinct types of statics are:
Descriptive Statistics
Inferential Statistics
In Statistics, descriptive statistics outline the given data whereas inferential statistics enable you to make estimations about the data. In inferential statistics, the data are drawn from the sample and enable you to generalise the population. Basically, inference means “guess” which implies making inference about something. So, statistical inference means making inference about the large population. It uses different statistical analysis techniques to draw conclusions about the population.
2. What is the Aim of Learning Statistical Inference?
Inferential statistics use data from the sample and then make inferences about the larger population, from which the sample can be drawn. Statistical inference aims to draw conclusions from a sample and derive them to the large population. It examines the probabilities of characteristics of the sample through probability theory. The most common methods used are hypothesis tests, Analysis of variance etc.
For example: Suppose, we are interested to know the exam marks of all the students in India. But, it is not possible to measure the examination marks of all the students in India. So for this, we will measure the marks of a smaller sample of 1000 students. This small sample enables us to characterize the marks of the larger population of Indian students.