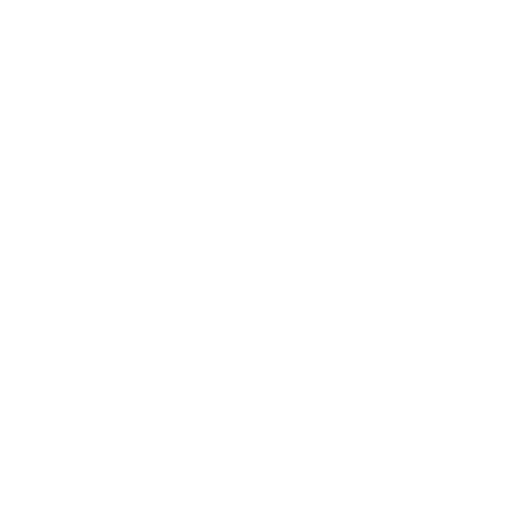

What is Beta Distribution?
The Beta Distribution is the type of the probability distribution related to probabilities that typically models the ancestry of probabilities. The Beta curve distribution is a versatile and resourceful way of describing outcomes for the percentages or the proportions. As the Beta Distribution basically represents the probability, its domain is restricted between 0 and 1. For instance, what is the possibility of Vladmir Putin winning the next presidential election in Russia? While some might think the probability for that is 0.2, others might think it is 0.25. The Beta Distribution is a concept that provides a way of explaining this.
The Examples of Beta Distribution
The Beta Distribution can be used for representing the different probabilities as follows.
The likelihood of the audience rating the new movie release.
The click-through rate of the website, which is the proportion of visitors.
The conversion rate for buyers actually purchasing from your website.
What is the survival chance of a person having blood cancer.
The Formula for the Beta Distribution
The standard formula for Beta Distribution pdf is as follows.
\[f(x)=\frac{(x−a)^{p−1}(b−x)^{q−1}}{B(p,q)(b−a)^{p+q−1}} \] a≤x≤b;p,q>0
Here, p and q represent the shape parameters. ‘A’ and ‘b’ are used for representing lower and the upper bounds respectively for the distribution. B(p, q) is the beta function. The beta function has this formula:
\[ B(\alpha,\beta) = \int_{1}^{0}t^{(α−1)}(1−t)^{(\beta−1)}dt. \]
An event where the value of a = 0, and b = 1, is known as the standard Beta Distribution. Mathematical equation or formula related to standard Beta Distribution can be described as: \[F (x) = \frac{x^{p−1} (1−x)^{q−1}}{ B (p,q)} \] 0≤x≤1;p,q>0.
Generally the usual form of the distribution is described with regards to scale and location parameters. The beta is a little different in the sense the usual distribution regarding upper and lower bounds is described. However, the scale and location parameters are specified in the form of lower and the upper limits as mentioned below.
Location = a
Scale = b - a
The Application of Beta Density Function
Beta Distribution is implemented and integrated in a wide range of applications like Bayesian hypothesis testing, task duration modelling, and Rule of Succession. The Beta Distribution is particularly the right project and planning control systems such as CPM and PERT primarily due to the fact that function is contrived by the interval with the max value of 1 and min value of 0.
Solved Examples
There are various examples of Beta Distribution probability and solving them can help the students to understand them well and prepare for their exams. For instance you can find out about the probability of someone going out on a movie with you using the method of Beta Distribution. You can refer to the Vedantu notes for numerous solved examples and explanations behind it.
FAQs on Beta Distribution
1. How is Beta Distribution useful for probabilities?
If we only consider probability distribution for representing the probability, then any arbitrary distribution across (0,1) would work in that order. And making one of these should be easy. You need to lay hold on the function which doesn’t boom at any point between 0 and 1 and also remains positive. Now you can integrate it from 0 to 1, and then divide the function using that outcome. You obtain the probability distribution which can be employed directly to represent the probability.
2. Why is beta distribution considered special?
The Beta Distribution is considered the conjugate before Bernoulli, binomial, geometric distributions, and negative binomial in the Bayesian hypotesizing.As the machine learning scientist, you specific is hardly ever complete and you must keep updating the model as new data flows in and this is why there is an insistence on usage of the Bayesian Inference. The computation involved in the Bayesian inference can be quite difficult or unimaginable. However, if we can execute closed-form beta formulas with conjugate prior, computation then becomes a walkover.
3. What are the common uses of beta distribution?
The Beta Distribution model is commonly used to document uncertainty regarding probability of the success of random experiments. In project management, the 3 point technique known as Beta Distribution is deployed that recognizes the uncertainty in estimation of project time. It also provides quantitative tools that are powerful combined with basic stats for computing the confidence levels of expected completion time. The Beta Distribution is also widely used in PERT for producing the bell-shaped curve.
4. What can i expect from the vedantu notes on “beta distribution”?
You can expect accurate and extremely insightful notes on “Beta Distribution” from Vedantu. These notes are drafted by the expert professionals with high proficiency in the subject. These notes cover all aspects related to the Beta Distribution including definition, function, formula, applications, logic, and solved examples. These notes are specially created keeping the students’ comprehension capabilities in mind to provide them with the best resource for learning.
5. How can i download the notes on “beta distribution” from vedantu?
You can conveniently download the “Beta Distribution” notes from Vedantu in the PDF file format. This file containing the detailed notes can be downloaded from the app or the website for free. You need to visit this specific section under maths, and then click on the “Download PDF” option that is provided here. The PDF file containing the notes on “Beta Distribution” will now be downloaded on your device.
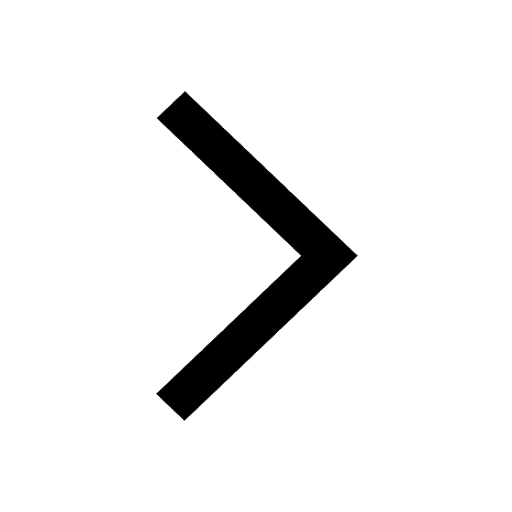
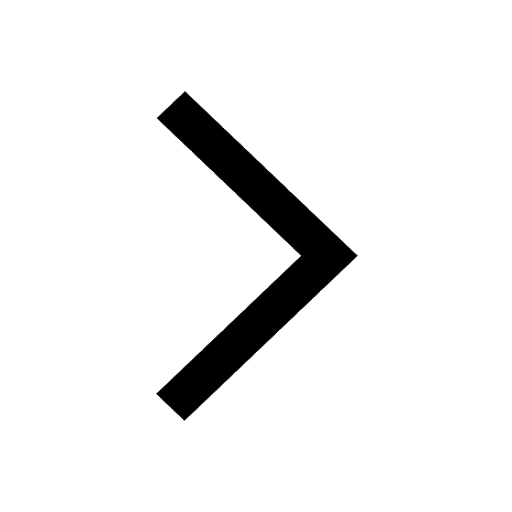
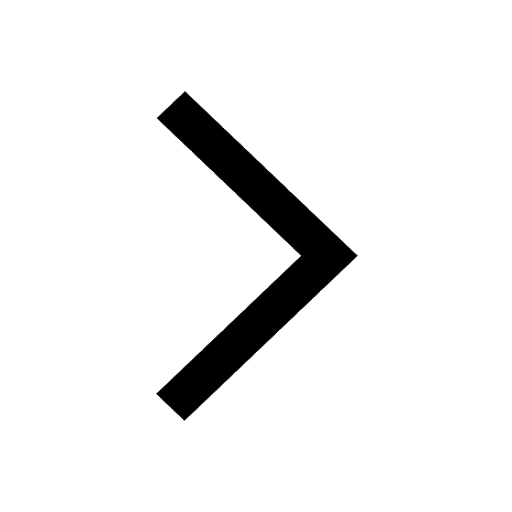
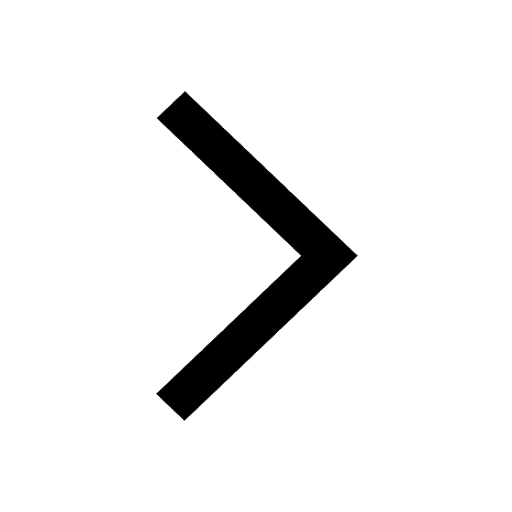
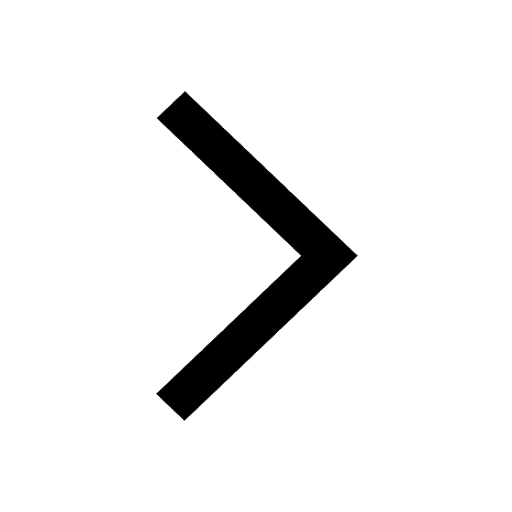
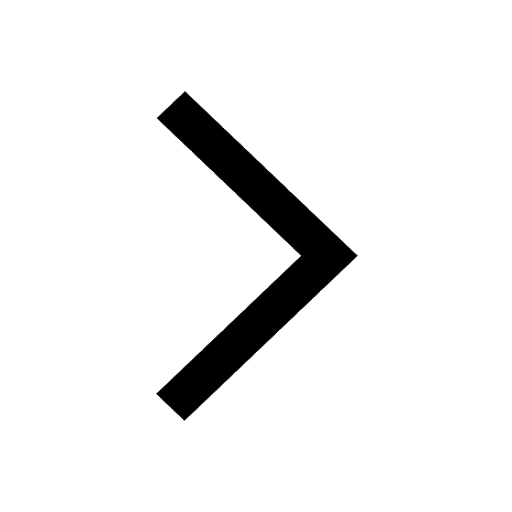
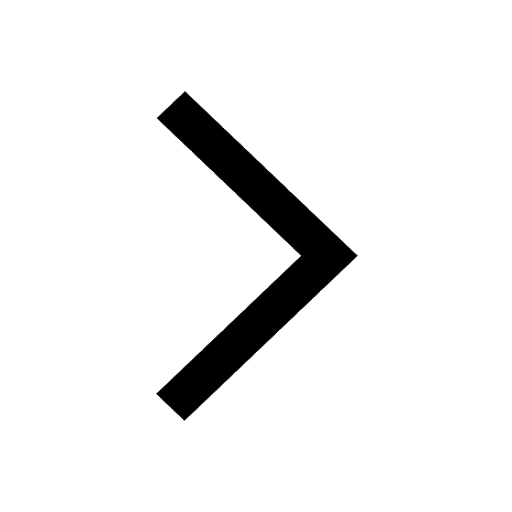
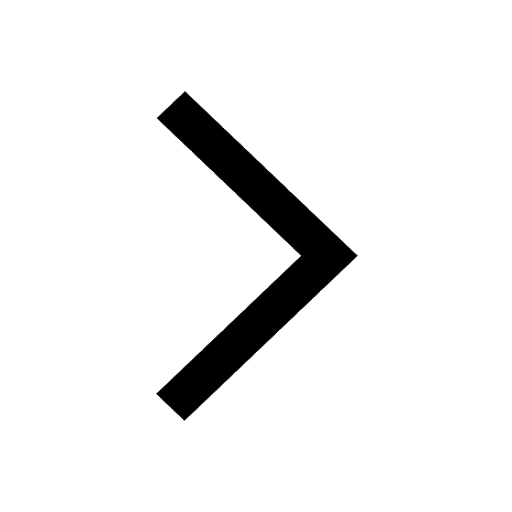
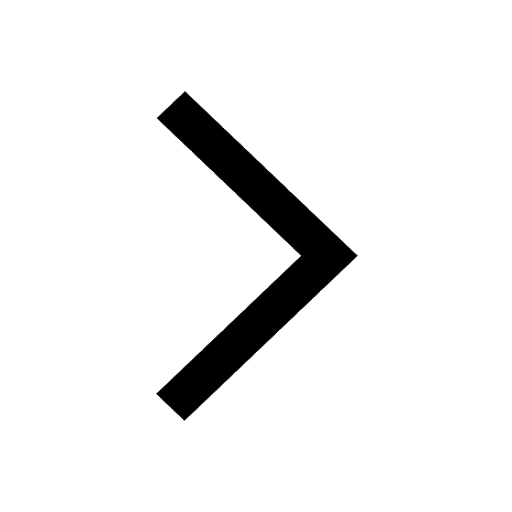
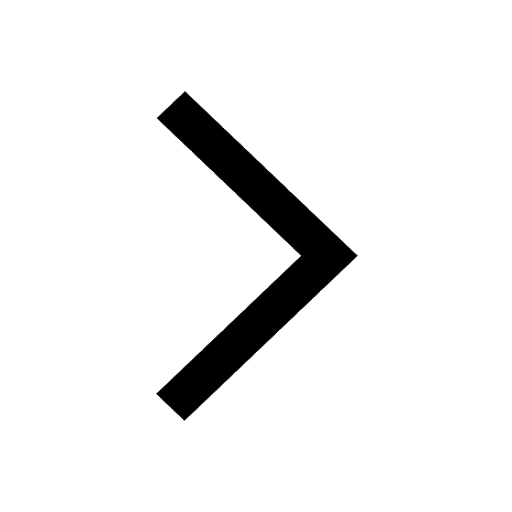
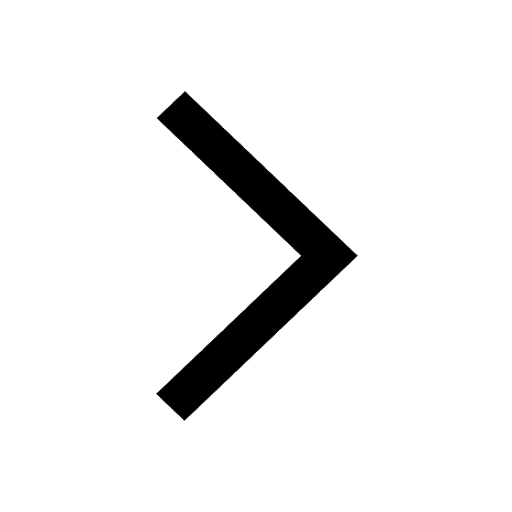
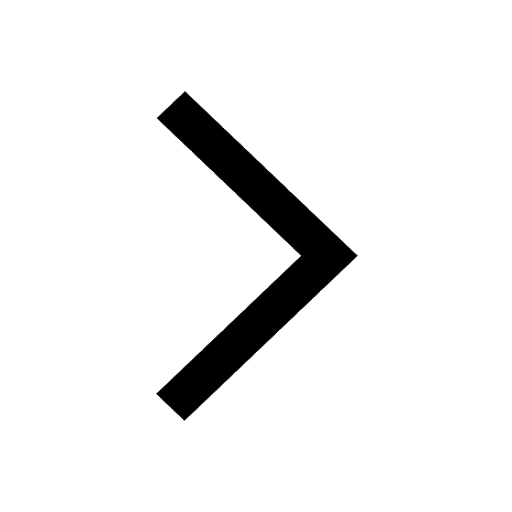
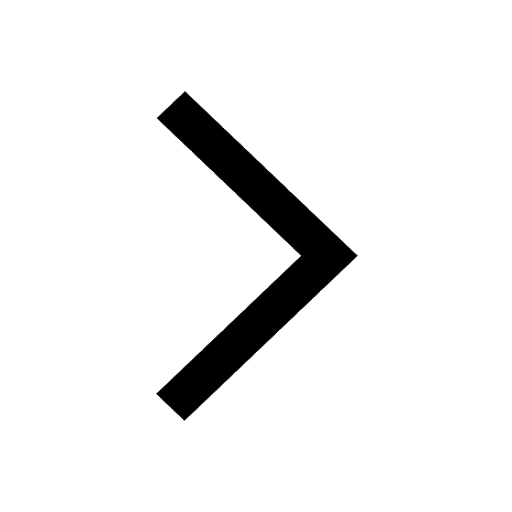
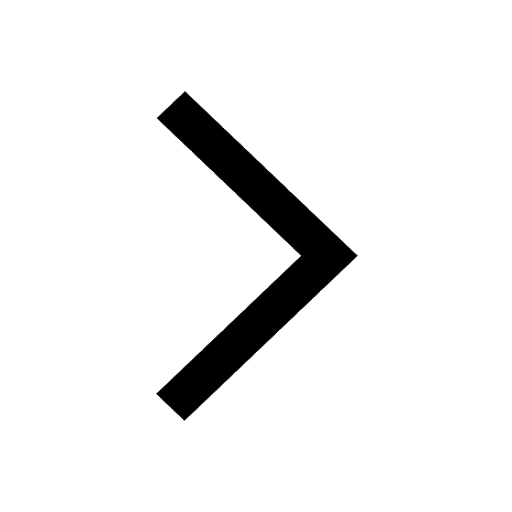
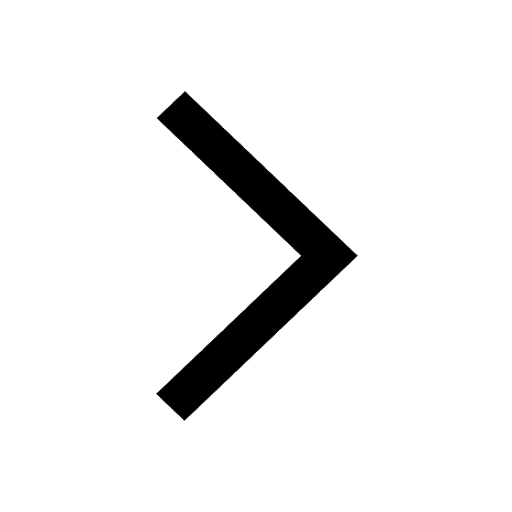
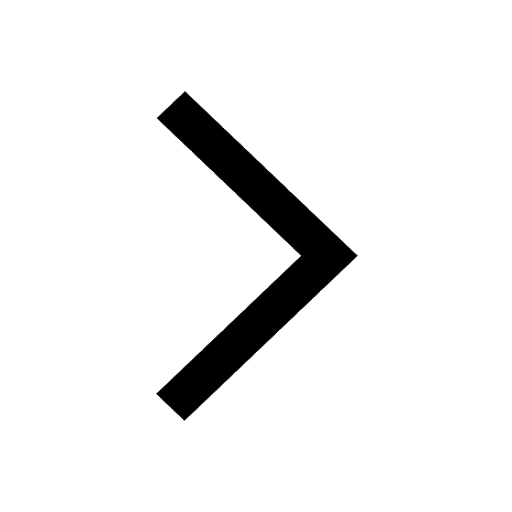
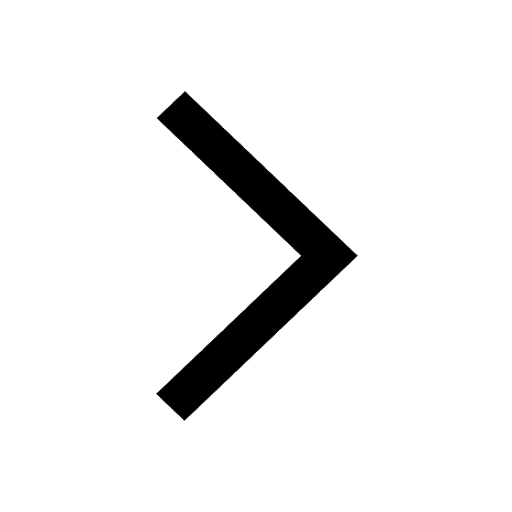
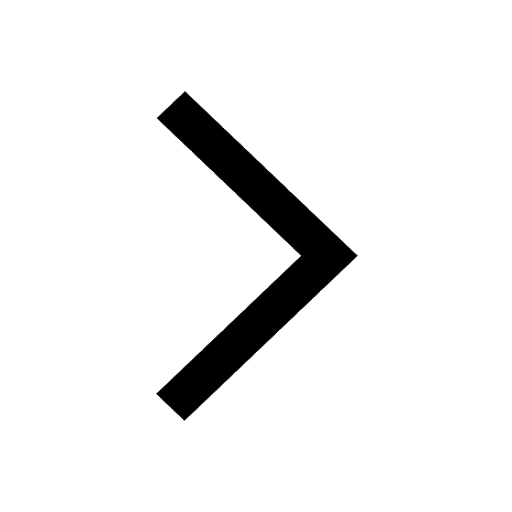