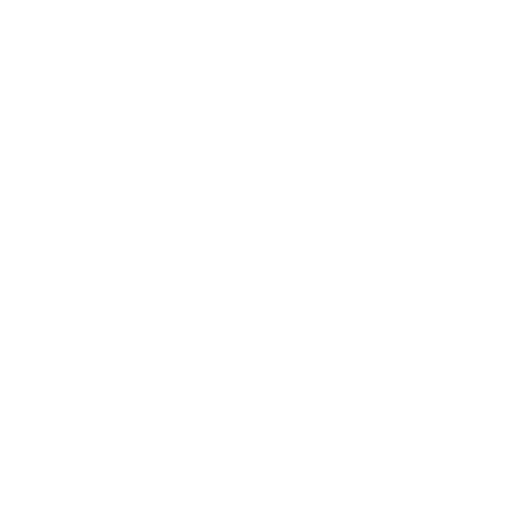

What is Covariance in Statistics?
Statistics is a major phenomenon in mathematics and all of us have studied it in all forms. Covariance is a part of statistics and it is the measure of the relationship between two random variables or random problems. This helps us figure out the changes in different variables. As the word itself suggests, Co-variance, lets different variables correlate and vary.
Covariance is somewhat related to variance The difference is just that 'variance' talks about a single variable, whereas 'covariance' talks about two variables. One more thing to note about covariance is that it might produce positive as well as negative values of variables. These given values can also be known as the following:
1. Positive Covariance - This tells us that the two variables are supposed to go in the same direction.
2. Negative Covariance - This tells us that the two variables tend to go about in inverse directions.
Covariance Formula in Statistics
Definition: Suppose X and Y are random variables with means µXand µY. The covariance of X and Y is defined as -
cov(x,y) = \[\frac{\sum _{i=1}^{n}\left ( x_{i}-\bar{x} \right )\left ( y_{i}-\bar{y} \right )}{n-1}\]
where xi= the values of the X- variable
yi = the values of the X- variable
x = Mean or the average of the X variable
y = Mean or the average of the Y variable
N = Number of datapoints
Cov(x,y) = Covariance of variables x and y
In this Covariance formula in statistics, we can see that the covariance of the two variables x and y is equal to the sum of the products of the differences of each value and the mean of its variables and finally divided by one less than the total number of data points. The x and y with a bar represent the means of each variable.
As Statistics have a formula or a solution for everything, covariance has a formula that includes some important points that students should notice. Those points are mentioned below-
Covariance is known to measure and observe the relationship between the two variables, their movement, and asset prices.
Whenever two variables move together, they are considered to be positive covariance. Whereas, on the other hand, when they move inversely, they are considered to be negative covariance.
By comparing or pairing assets with negative covariance, risks and volatility are reduced.
It is also a significant tool that ascertains what securities to put in the portfolio in modern portfolio theory.
Covariance Evaluation
To understand the covariance one should know how it works, it evaluates the positioning of two variables moving together. When two stocks, let stock A and stock B move together, when stock A moves higher and also stock B moves higher equally and vice versa for the negative fall then these both stocks are said to be positive covariance. Security holdings are diversified and calculated through the covariance, in finance.
Covariance - Applications
The covariance has much-diversified application with respect to financing and the modern portfolio theory like -
CAPM stands for a capital asset pricing model that is calculated to expect the return that an asset would offer.
The formula of the model key variables is created through the covariance between a market and its security.
The covariance help to evaluate a systematic risk compared to the market as a whole.
Properties of Covariance
CovX, c = 0 for any constant c.
CovaX, Y = a · CovX, Y CovX, aY = a · CovX, Y
CovX, Y = CovY, X
CovX, X = VarX
Bilinearity (a.k.a. distributive property): CovX+Y, Z = CovX, Z + CovY, Z CovX, Y+Z = CovX, Y + CovX, Z
What is the Formula for Covariance? (Population and Sample Covariance Formula)
Notations in the Formula for Covariance
xi= data value of x
yi = data value of y
x̄ = mean of x
ȳ = mean of y
N = number of data values.
Key Takeaways (Covariance in Finance)
Covariance is known to be a statistical tool that can be used to determine the relationship between the movement of any two asset prices.
When two stocks tend to move together, then they are seen as having a positive covariance; when they move inversely, the covariance is basically negative.
Covariance can be a significant tool in modern portfolio theory used to ascertain what securities to put in a portfolio.
Risk and volatility can also be reduced in a portfolio by pairing assets that have a negative covariance.
Questions to be Solved
1: Compute the value of covariance i.e Cov(x,y) for the given data set.
Solution: First, Let’s find the mean of each variable. We know the formula for covariance
Thus, x̄ =(98+87+90+85+95+75)/6 = 88. 33
ȳ =(15+12+10+10+16+7)/6 = 11.67
Now, we subtract each value from its respective mean and then multiply these new values together.
The next step is to add all the products together, which is 125.66.
Now, divide the above value by (n-1) i.e by (6 – 1) i.e. 5.
Therefore, Cov(x,y) = 125.66/5 = 25.132
FAQs on Covariance Formula
1. What is Covariance Used for?
Covariance is a measure of how changes in one variable are associated with changes in a second variable. Covariance specifically measures the degree to which two variables are linearly associated. However, the concept of covariance is also often used informally as a general measure of how monotonically related two variables are.
2. What is the difference between Covariance and Correlation?
In simple words, both terms measure the relationship and the dependency between two variables. The term “Covariance” generally indicates the direction of the linear relationship between any given variables, suppose x and y. Whereas “Correlation” is known to measure both the strength and direction of the linear relationship between any two variables.
3. What does a Negative Covariance Mean?
Negative covariance is an indication that the movement in one variable is opposite to the movement of the other variable.
4. Can the Covariance be Greater than 1?
Covariance values are not standardized. Therefore, the covariance can range from negative infinity to positive infinity, this is the range or limit. Thus, the value for a perfect linear relationship depends on the data values. Because the data are not standardized, it is difficult to determine the strength of the relationship between the variables.
5. Why is Correlation Preferred Over Covariance?
Now, when it comes to making a choice, which is a better measure of the relationship between two variables, correlation is preferred over covariance, because it remains unaffected by the change in location and scale, and can be used to make a comparison between two pairs of variables too.
6. What is the covariance and what are its negative and positive effects?
As the word itself suggests, Co-variance, lets different variables correlate and vary. Covariance is a part of statistics and it is the measure of the relationship between two random variables or random problems. Positive Covariance tells us that the two variables are supposed to go in the same direction. Negative Covariance tells us that the two variables tend to go about in inverse directions. This helps us figure out the changes in different variables.
7. What is the effect of covariance on the stocks of a company?
Covariance makes two stocks move simultaneously If stock A and stock B move together, when stock A move higher and also stock B moves higher equally and vice versa for the negative fall then these both stocks are said to be positive covariance. It means that two stocks increase or decrease their rate together. Security holdings are diversified and calculated through the covariance, in finance.
8. What is the measure that the formula of covariance defies?
Covariance is known to measure and observe the relationship between the two variables, their movement, and asset prices. It is also a significant tool that ascertains what securities to put in the portfolio in modern portfolio theory. By comparing or pairing assets with negative covariance, risks and volatility are reduced. Also, we can understand the nature of stock.
9. What are the uses of Covariance?
Covariance has diversified use in finance. Among all the uses the major use of the covariance is to evaluate the change of a variable with another variable. It is specially used to measure the degree of linearly associated two variables. Many of the associates use covariance for their informal use to measure how two variables are monotonically related.
10. Why does covariance need at least two variables?
The word itself suggests, Co-variance, which lets different variables correlate and vary. Hence when the term co-relation is used it signifies relation. The difference is just that 'variance' talks about a single variable, whereas 'covariance' talks about two variables. And to establish a relationship there should be a minimum of two variables to relate. Hence, in covariance, there should be a minimum of two variables so that they can be evaluated together. This helps us figure out the changes in different variables.
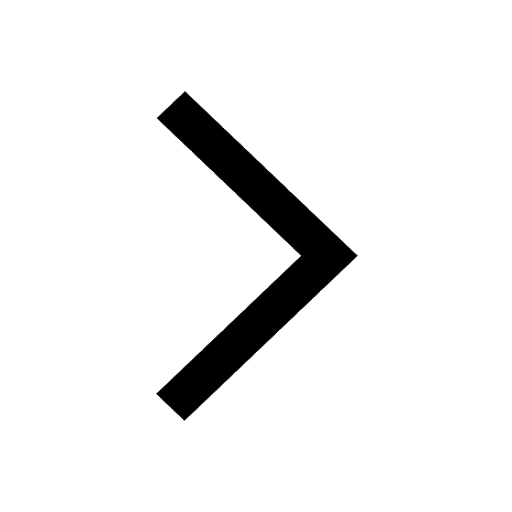
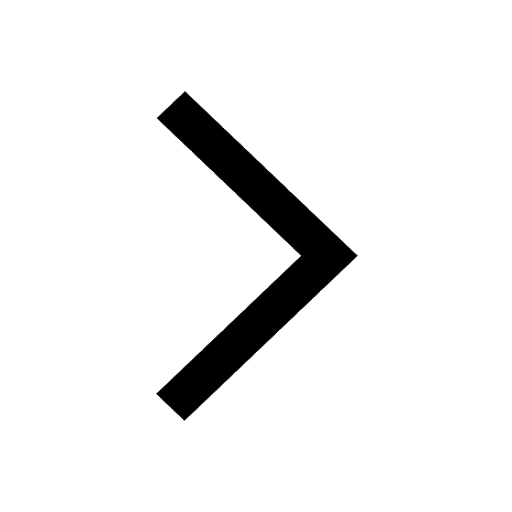
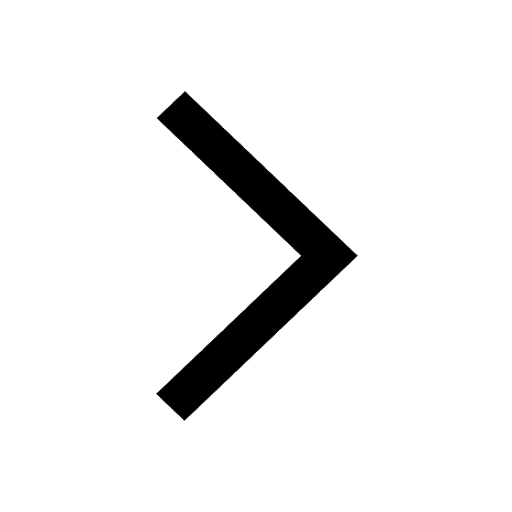
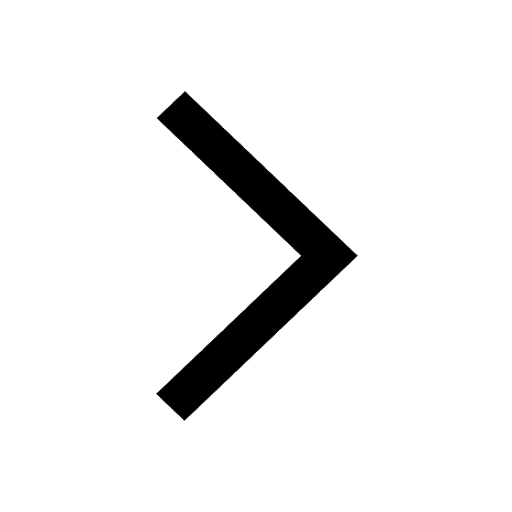
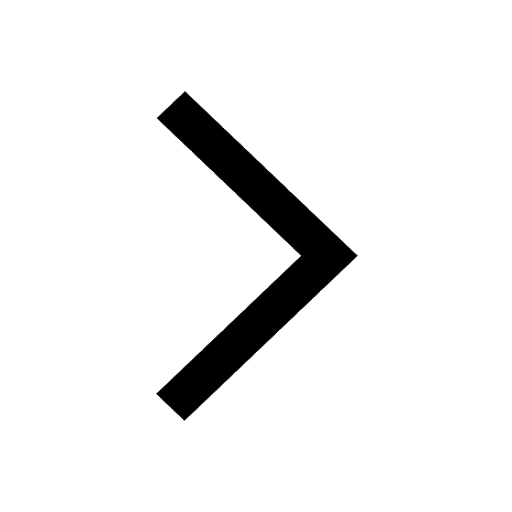
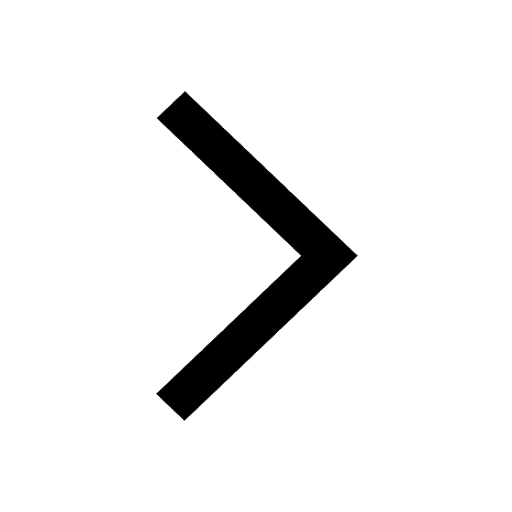