
What is Chebyshev’s inequality?
Answer
443.7k+ views
Hint: We need to explain the concept of Chebyshev’s inequality by using the concept of an example. Chebyshev’s inequality is a concept used for random variables to determine the maximum number of extreme values. Its equation can be represented by
Complete step by step solution:
In order to answer this question, let us first explain the concept of Chebyshev’s inequality. It is an important concept in probability used to determine the extreme values. We can write its equation as follows,
Here, X is a random variable having a mean given by and a finite variance k is a positive real number such that which represents a certain distance away from the mean expressed in terms of standard deviation. Let us consider the example of calculating the event of tossing a coin 10 times in which we are required to calculate the probability of getting the number of tails greater than 7 or less than 3. The boundary value can be found using Chebyshev's inequality.
Let X be the number of tails. The expected value is given by the product of the number of tries and the probability of getting a tail which is 0.5
The variance can be calculated as,
Standard deviation can be calculated as
This is the same as the value k. Hence, using Chebyshev’s inequality for more than 7 tails and less than 3 tails can be given by,
This can be simplified to,
Hence, the maximum probability for the case of obtaining more than 7 tails and less than 3 tails is given by 0.4.
Hence, we have explained the concept of Chebyshev’s inequality.
Note: We need to note that Chebyshev's inequality is an important concept used for many mathematical problems. It can be used to determine the outliers of a group of clustered data. It can also determine the characteristics of probability distributions.
Complete step by step solution:
In order to answer this question, let us first explain the concept of Chebyshev’s inequality. It is an important concept in probability used to determine the extreme values. We can write its equation as follows,
Here, X is a random variable having a mean given by
Let X be the number of tails. The expected value is given by the product of the number of tries and the probability of getting a tail which is 0.5
The variance can be calculated as,
Standard deviation can be calculated as
This is the same as the value k. Hence, using Chebyshev’s inequality for more than 7 tails and less than 3 tails can be given by,
This can be simplified to,
Hence, the maximum probability for the case of obtaining more than 7 tails and less than 3 tails is given by 0.4.
Hence, we have explained the concept of Chebyshev’s inequality.
Note: We need to note that Chebyshev's inequality is an important concept used for many mathematical problems. It can be used to determine the outliers of a group of clustered data. It can also determine the characteristics of probability distributions.
Recently Updated Pages
Master Class 12 Business Studies: Engaging Questions & Answers for Success
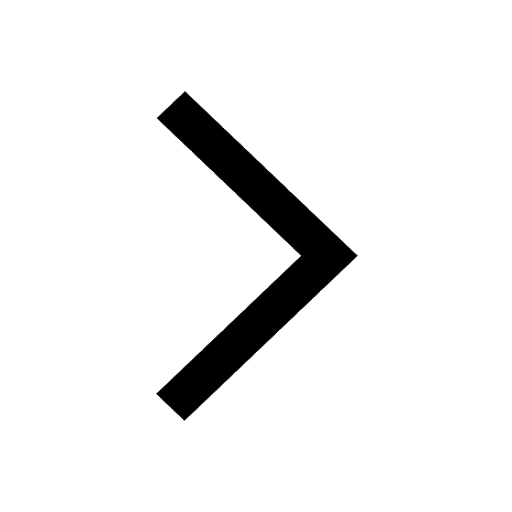
Master Class 12 Economics: Engaging Questions & Answers for Success
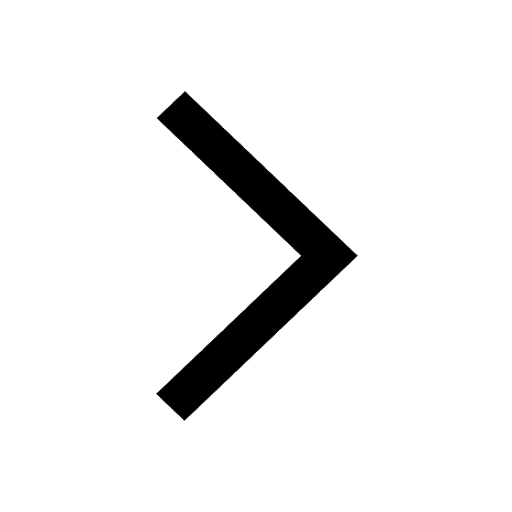
Master Class 12 Maths: Engaging Questions & Answers for Success
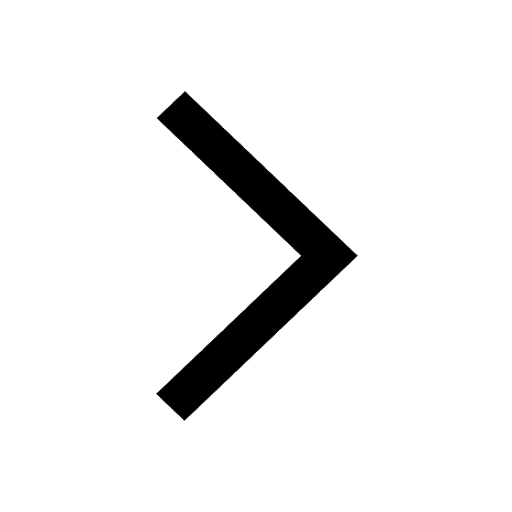
Master Class 12 Biology: Engaging Questions & Answers for Success
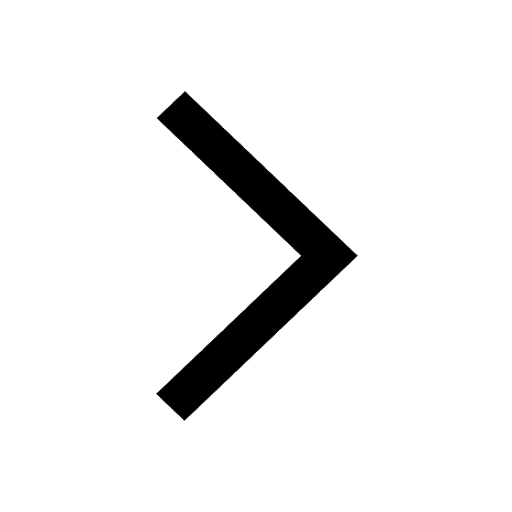
Master Class 12 Physics: Engaging Questions & Answers for Success
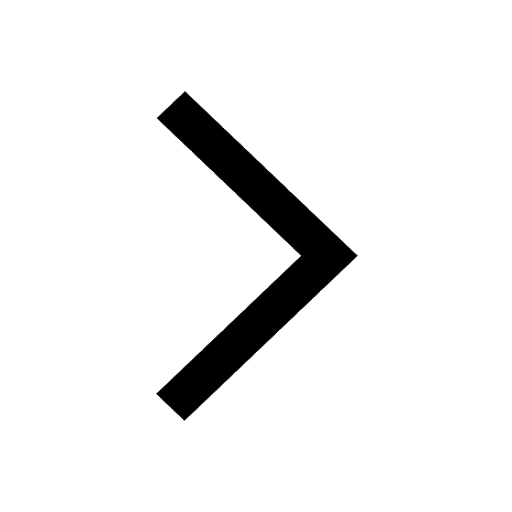
Master Class 12 English: Engaging Questions & Answers for Success
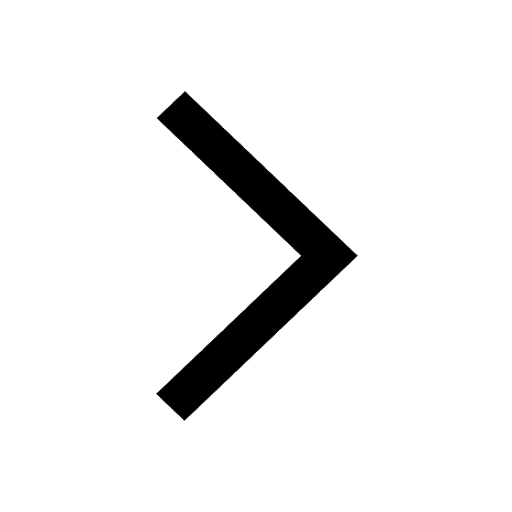
Trending doubts
Why should a magnesium ribbon be cleaned before burning class 12 chemistry CBSE
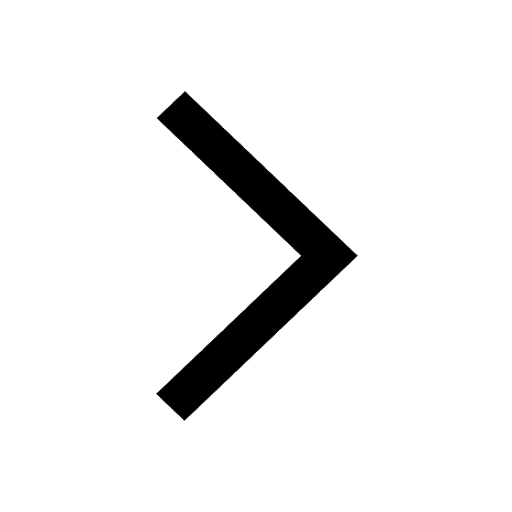
A renewable exhaustible natural resources is A Coal class 12 biology CBSE
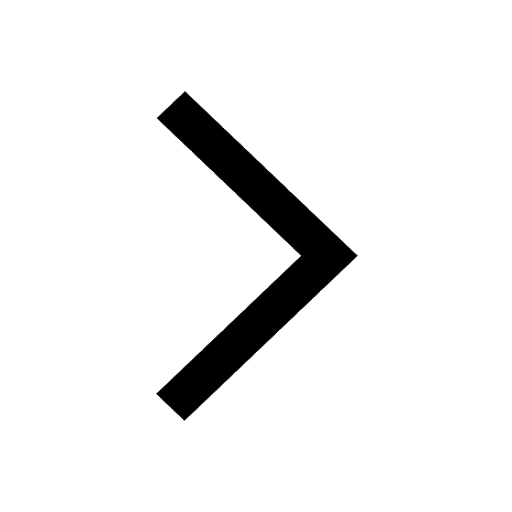
Megasporangium is equivalent to a Embryo sac b Fruit class 12 biology CBSE
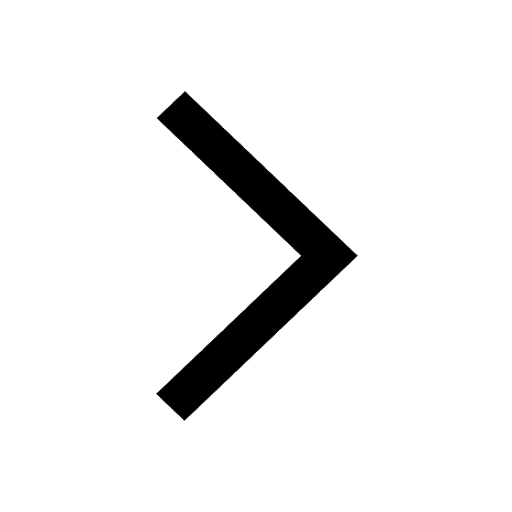
What is Zeises salt and ferrocene Explain with str class 12 chemistry CBSE
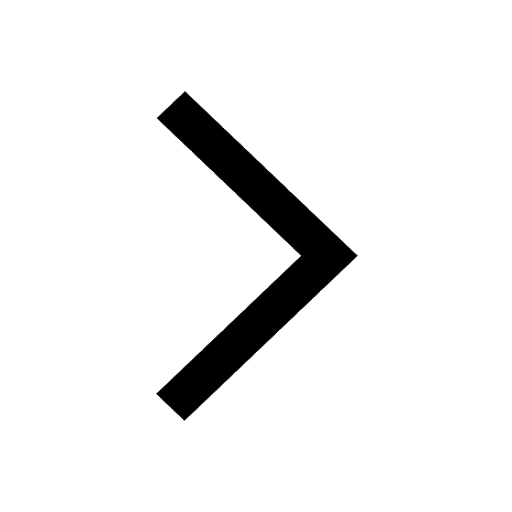
How to calculate power in series and parallel circ class 12 physics CBSE
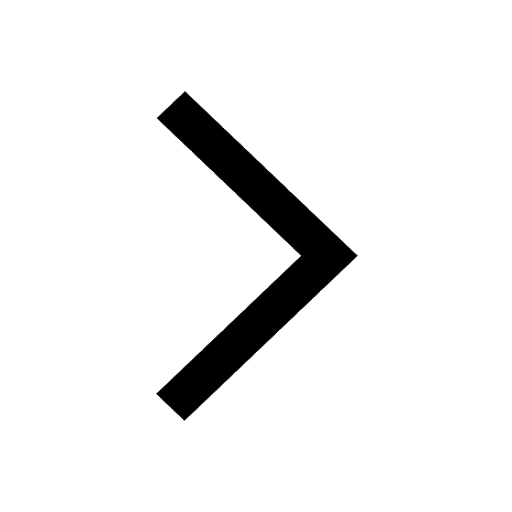
Anal style is present in A Male cockroach B Female class 12 biology CBSE
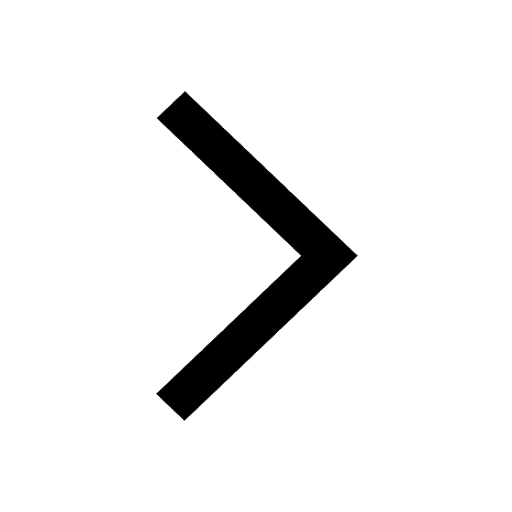